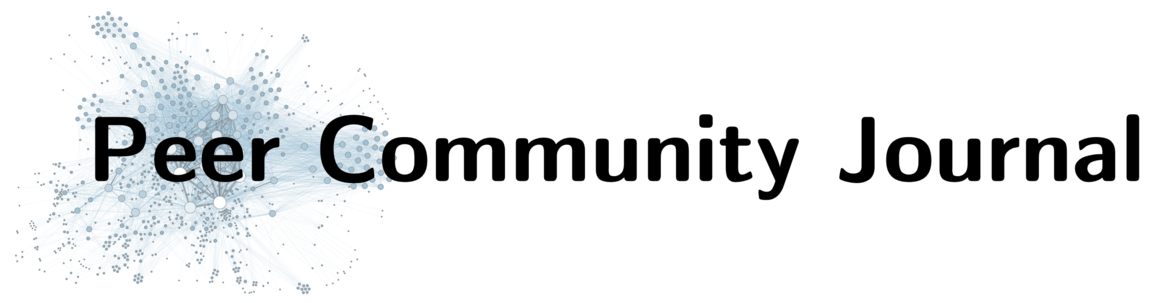
Section: Ecology
Topic:
Ecology,
Statistics
Using informative priors to account for identifiability issues in occupancy models with identification errors
Corresponding author(s): Monchy, Célian (celian.monchy@cefe.cnrs.fr)
10.24072/pcjournal.511 - Peer Community Journal, Volume 5 (2025), article no. e8.
Get full text PDF Peer reviewed and recommended by PCI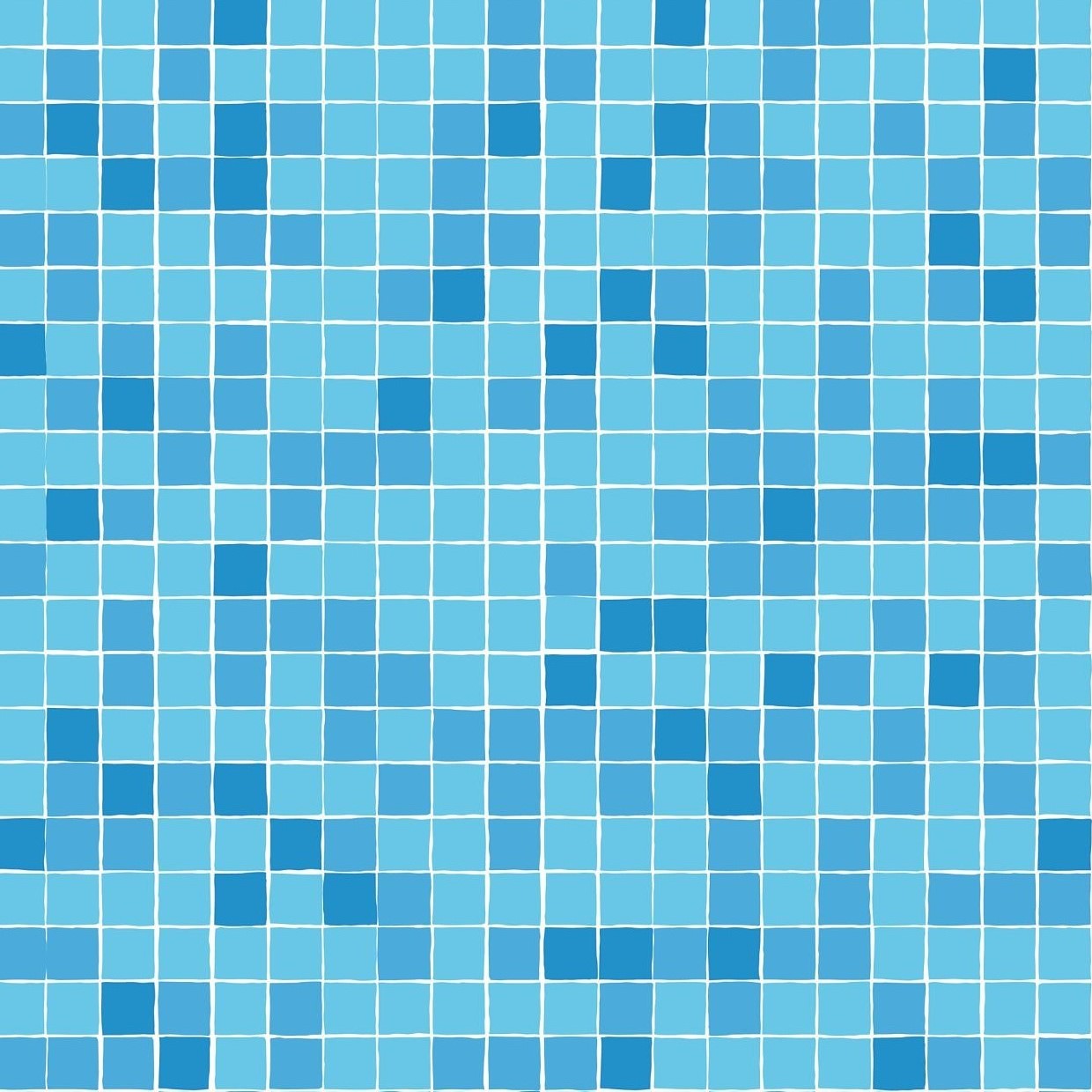
Non-invasive monitoring techniques like camera traps, autonomous recording units and environmental DNA are increasingly used to collect data for understanding species distribution. These methods have prompted the development of statistical models to suit specific sampling designs and get reliable ecological inferences. Site occupancy models estimate species occurrence patterns, accounting for the possibility that the target species may be present but unobserved. Here, two key processes are crucial: detection, when a species leaves signs of its presence, and identification where these signs are accurately recognized. While both processes are prone to error in general, wrong identifications are often considered as negligible with in situ observations. When applied to passive bio-monitoring data, characterized by datasets requiring automated processing, this second source of error can no longer be ignored as misclassifications at both steps can lead to significant biases in ecological estimates. Several model extensions have been proposed to address these potential errors. We propose an extended occupancy model that accounts for the identification process in addition to detection. Similar to other recent attempts to account for false positives, our model may suffer from identifiability issues, which usually require another source of data with perfect identification to resolve them. As an alternative when such data are unavailable, we propose leveraging existing knowledge of the identification process within a Bayesian framework by incorporating this knowledge through an informative prior. Through simulations, we compare different prior choices that encode varying levels of information, ranging from cases where no prior knowledge is available, to instances with accurate metrics on the performance of the identification, and scenarios based on generally accepted assumptions. We demonstrate that, compared to using a default prior, integrating information about the identification process as a prior reduces bias in parameter estimates. Overall, our approach mitigates identifiability issues, reduces estimation bias, and minimizes data requirements. In conclusion, we provide a statistical method applicable to various monitoring designs, such as camera trap, bioacoustics, or eDNA surveys, alongside non-invasive sampling technologies, to produce ecological outcomes that inform conservation decisions.
Type: Research article
Monchy, Célian 1; Etienne, Marie-Pierre 2; Gimenez, Olivier 1
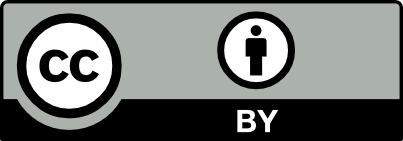
@article{10_24072_pcjournal_511, author = {Monchy, C\'elian and Etienne, Marie-Pierre and Gimenez, Olivier}, title = {Using informative priors to account for identifiability issues in occupancy models with identification errors}, journal = {Peer Community Journal}, eid = {e8}, publisher = {Peer Community In}, volume = {5}, year = {2025}, doi = {10.24072/pcjournal.511}, language = {en}, url = {https://peercommunityjournal.org/articles/10.24072/pcjournal.511/} }
TY - JOUR AU - Monchy, Célian AU - Etienne, Marie-Pierre AU - Gimenez, Olivier TI - Using informative priors to account for identifiability issues in occupancy models with identification errors JO - Peer Community Journal PY - 2025 VL - 5 PB - Peer Community In UR - https://peercommunityjournal.org/articles/10.24072/pcjournal.511/ DO - 10.24072/pcjournal.511 LA - en ID - 10_24072_pcjournal_511 ER -
%0 Journal Article %A Monchy, Célian %A Etienne, Marie-Pierre %A Gimenez, Olivier %T Using informative priors to account for identifiability issues in occupancy models with identification errors %J Peer Community Journal %D 2025 %V 5 %I Peer Community In %U https://peercommunityjournal.org/articles/10.24072/pcjournal.511/ %R 10.24072/pcjournal.511 %G en %F 10_24072_pcjournal_511
Monchy, Célian; Etienne, Marie-Pierre; Gimenez, Olivier. Using informative priors to account for identifiability issues in occupancy models with identification errors. Peer Community Journal, Volume 5 (2025), article no. e8. doi : 10.24072/pcjournal.511. https://peercommunityjournal.org/articles/10.24072/pcjournal.511/
PCI peer reviews and recommendation, and links to data, scripts, code and supplementary information: 10.24072/pci.ecology.100699
Conflict of interest of the recommender and peer reviewers:
The recommender in charge of the evaluation of the article and the reviewers declared that they have no conflict of interest (as defined in the code of conduct of PCI) with the authors or with the content of the article.
[1] Towards estimating marine wildlife abundance using aerial surveys and deep learning with hierarchical classifications subject to error, bioRxiv (2023) | DOI
[2] The use of Bayesian priors in Ecology: The good, the bad and the not great, Methods in Ecology and Evolution, Volume 11 (2020) no. 8, pp. 882-889 | DOI
[3] Accounting for automated identification errors in acoustic surveys, Methods in Ecology and Evolution, Volume 10 (2019) no. 8, pp. 1171-1188 | DOI
[4] A new statistical approach for identifying rare species under imperfect detection, Diversity and Distributions, Volume 28 (2022) no. 5, pp. 882-893 | DOI
[5] Wildlife camera trapping: a review and recommendations for linking surveys to ecological processes, Journal of Applied Ecology, Volume 52 (2015) no. 3, pp. 675-685 | DOI
[6] Improving distribution data of threatened species by combining acoustic monitoring and occupancy modelling, Methods in Ecology and Evolution, Volume 7 (2016) no. 11, pp. 1340-1348 | DOI
[7] Modeling false positive detections in species occurrence data under different study designs, Ecology, Volume 96 (2015) no. 2, pp. 332-339 | DOI
[8] Elicitation by design in ecology: using expert opinion to inform priors for Bayesian statistical models, Ecology, Volume 90 (2009) no. 1, pp. 265-277 | DOI
[9] Determining the parametric structure of models, Mathematical Biosciences, Volume 228 (2010) no. 1, pp. 16-30 | DOI
[10] Uses and Misuses of Environmental DNA in Biodiversity Science and Conservation, Annual Review of Ecology, Evolution, and Systematics, Volume 49 (2018), pp. 209-230 | DOI
[11] Quantifying data quality in a citizen science monitoring program: False negatives, false positives and occupancy trends, Conservation Science and Practice, Volume 1 (2019) no. 7, p. e54 | DOI
[12] Integrating species distribution and occupancy modeling to study hellbender (Cryptobranchus alleganiensis) occurrence based on eDNA surveys, Biological Conservation, Volume 251 (2020), p. 108787 | DOI
[13] From molecules to management: Adopting DNA-based methods for monitoring biological invasions in aquatic environments, Environmental Research, Volume 111 (2011) no. 7, pp. 978-988 | DOI
[14] Geographically Assisted Elicitation of Expert Opinion for Regression Models, Bayesian Analysis, Volume 2 (2007) no. 1, pp. 99-136 | DOI
[15] Evaluation of detection probabilities at the water-filtering and initial PCR steps in environmental DNA metabarcoding using a multispecies site occupancy model, Scientific Reports, Volume 9 (2019) no. 1, p. 3581 | DOI
[16] An approach to rapid processing of camera trap images with minimal human input, Ecology and Evolution, Volume 11 (2021) no. 17, pp. 12051-12063 | DOI
[17] Methods for Eliciting Informative Prior Distributions: A Critical Review, Decision Analysis, Volume 19 no. 3, pp. 189-204 | DOI
[18] Occupancy models for data with false positive and false negative errors and heterogeneity across sites and surveys, Methods in Ecology and Evolution, Volume 6 (2015) no. 12, pp. 1395-1406 | DOI
[19] Component processes of detection probability in camera-trap studies: understanding the occurrence of false-negatives, Mammal Research, Volume 65 (2020) no. 2, pp. 167-180 | DOI
[20] A framework for estimating the sensitivity of eDNA surveys, Molecular Ecology Resources, Volume 16 (2016) no. 3, pp. 641-654 | DOI
[21] Trade-off between deep learning for species identification and inference about predator-prey co-occurrence, Computo (2022) | DOI
[22] Methods for investigating parameter redundancy, Animal Biodiversity and Conservation, Volume 27 (2004) no. 1, pp. 561-572 | DOI
[23] A comparison of biomonitoring methodologies for surf zone fish communities, PLOS ONE, Volume 18 (2023) no. 6, p. e0260903 | DOI
[24] Critical considerations for the application of environmental DNA methods to detect aquatic species, Methods in Ecology and Evolution, Volume 7 (2016) no. 11, pp. 1299-1307 | DOI
[25] Integrating multiple sign types to improve occupancy estimation for inconspicuous species, Ecology and Evolution, Volume 13 (2023) no. 5, p. e10019 | DOI
[26] Modelling environmental DNA data; Bayesian variable selection accounting for false positive and false negative errors, Journal of the Royal Statistical Society: Series C (Applied Statistics), Volume 69 (2020) no. 2, pp. 377-392 | DOI
[27] Dealing with false-positive and false-negative errors about species occurrence at multiple levels, Methods in Ecology and Evolution, Volume 8 (2017) no. 9, pp. 1081-1091 | DOI
[28] Novel community data in ecology-properties and prospects, Trends in Ecology & Evolution, Volume 39 (2024) no. 3, pp. 280-293 | DOI
[29] Framing pictures: A conceptual framework to identify and correct for biases in detection probability of camera traps enabling multi‐species comparison, Ecology and Evolution, Volume 9 (2019) no. 4, pp. 2320-2336 | DOI
[30] Environmental DNA (eDNA) Sampling Improves Occurrence and Detection Estimates of Invasive Burmese Pythons, PLOS ONE, Volume 10 (2015) no. 4, p. e0121655 | DOI
[31] Diagnostic assays and sampling protocols for the detection of Batrachochytrium dendrobatidis, Diseases of Aquatic Organisms, Volume 73 (2007), pp. 175-192 | DOI
[32] Tracking an invasion front with environmental DNA, Ecological Applications, Volume 32 (2022) no. 4, p. e2561 | DOI
[33] Site-occupancy modelling as a novel framework for assessing test sensitivity and estimating wildlife disease prevalence from imperfect diagnostic tests, Methods in Ecology and Evolution, Volume 3 (2012) no. 2, pp. 339-348 | DOI
[34] Efficacy of machine learning image classification for automated occupancy‐based monitoring, Remote Sensing in Ecology and Conservation (2023), pp. 56-71 | DOI
[35] Estimating site occupancy rates when detection probabilities are less than one, Ecology, Volume 83 (2002) no. 8, pp. 2248-2255 | DOI
[36] A Systematic Review of Sources of Variability and Uncertainty in eDNA Data for Environmental Monitoring, Frontiers in Ecology and Evolution, Volume 8 (2020) | DOI
[37] Profiting from prior information in Bayesian analyses of ecological data, Journal of Applied Ecology, Volume 42 (2005) no. 6, pp. 1012-1019 | DOI
[38] Unmodeled observation error induces bias when inferring patterns and dynamics of species occurrence via aural detections, Ecology, Volume 91 (2010) no. 8, pp. 2446-2454 | DOI
[39] Seeking a second opinion: uncertainty in disease ecology: Uncertainty in disease ecology, Ecology Letters, Volume 13 (2010) no. 6, pp. 659-674 | DOI
[40] To model or not to model: false positive detection error in camera surveys, The Journal of Wildlife Management, Volume 87 (2023) no. 3, p. e22365 | DOI
[41] Improving occupancy estimation when two types of observational error occur: non-detection and species misidentification, Ecology, Volume 92 (2011) no. 7, pp. 1422-1428 | DOI
[42] Using informative priors to account for identifiability issues in occupancy models with identification errors, Zenodo, 2024 | DOI
[43] The neglected tool in the Bayesian ecologist's shed: a case study testing informative priors' effect on model accuracy, Ecology and Evolution, Volume 5 (2015) no. 1, pp. 102-108 | DOI
[44] NIMBLE: MCMC, Particle Filtering, and Programmable Hierarchical Modeling (version 1.2.0), Zenodo, 2024 | DOI
[45] Multi-scale occupancy estimation and modelling using multiple detection methods, Journal of Applied Ecology, Volume 45 (2008) no. 5, pp. 1321-1329 | DOI
[46] A deep active learning system for species identification and counting in camera trap images, Methods in Ecology and Evolution, Volume 12 (2021) no. 1, pp. 150-161 | DOI
[47] A comment on priors for Bayesian occupancy models, PLOS ONE, Volume 13 (2018) no. 2, pp. 1-13 | DOI
[48] Prior specification in Bayesian occupancy modelling improves analysis of species occurrence data, Ecological Indicators, Volume 93 (2018), pp. 333-343 | DOI
[49] Do occupancy or detection rates from camera traps reflect deer density?, Journal of Mammalogy, Volume 98 (2017) no. 6, pp. 1547-1557 | DOI
[50] Machine learning and deep learning—A review for ecologists, Methods in Ecology and Evolution, Volume 14 (2023) no. 4, pp. 994-1016 | DOI
[51] The DeepFaune initiative: a collaborative effort towards the automatic identification of European fauna in camera trap images, European Journal of Wildlife Research, Volume 69 (2023) no. 6, p. 113 | DOI
[52] Passive acoustic monitoring provides a fresh perspective on fundamental ecological questions, Functional Ecology, Volume 37 (2023) no. 4, pp. 959-975 | DOI
[53] Generalized Site Occupancy Models Allowing for False Positive and False Negative Errors, Ecology, Volume 87 (2006) no. 4, pp. 835-841 | DOI
[54] Environmental DNA (eDNA): Powerful technique for biodiversity conservation, Journal for Nature Conservation, Volume 71 (2023), p. 126325 | DOI
[55] Site occupancy models in the analysis of environmental DNA presence/absence surveys: a case study of an emerging amphibian pathogen, Methods in Ecology and Evolution, Volume 4 (2013) no. 7, pp. 646-653 | DOI
[56] Recognition of European mammals and birds in camera trap images using deep neural networks, IET Computer Vision (2024) | DOI
[57] Autonomous recording units in avian ecological research: current use and future applications, Avian Conservation and Ecology, Volume 12 (2017) no. 1, p. 14 | DOI
[58] Estimating species misclassification with occupancy dynamics and encounter rates: A semi‐supervised, individual‐level approach, Methods in Ecology and Evolution, Volume 13 (2022) no. 7, pp. 1528-1539 | DOI
[59] Snapshot Serengeti, high-frequency annotated camera trap images of 40 mammalian species in an African savanna, Scientific Data, Volume 2 (2015) no. 1, p. 150026 | DOI
[60] Improving the accessibility and transferability of machine learning algorithms for identification of animals in camera trap images: MLWIC2, Ecology and Evolution, Volume 10 (2020) no. 19, pp. 10374-10383 | DOI
[61] Machine learning to classify animal species in camera trap images: Applications in ecology, Methods in Ecology and Evolution, Volume 10 (2019) no. 4, pp. 585-590 | DOI
[62] Use of Site Occupancy Models to Estimate Prevalence of Myxobolus cerebralis Infection in Trout, Journal of Aquatic Animal Health, Volume 19 (2007) no. 1, pp. 8-13 | DOI
[63] Monitoring endangered freshwater biodiversity using environmental DNA, Molecular Ecology, Volume 21 (2012) no. 11, pp. 2565-2573 | DOI
[64] Improving Precision and Reducing Bias in Biological Surveys: Estimating False-Negative Error Rates, Ecological Applications, Volume 13 (2003) no. 6, pp. 1790-1801 | DOI
[65] An evaluation of platforms for processing camera-trap data using artificial intelligence, Methods in Ecology and Evolution, Volume 14 (2023) no. 2, pp. 459-477 | DOI
[66] The effectiveness and cost of camera traps for surveying small reptiles and critical weight range mammals: A comparison with labour-intensive complementary methods, Wildlife Research, Volume 42 (2015), pp. 414-425 | DOI
[67] Wildlife Insights (https://www.wildlifeinsights.org/about-wildlife-insights-ai)
[68] The importance of including imperfect detection models in eDNA experimental design, Molecular Ecology Resources, Volume 16 (2016) no. 4, pp. 837-844 | DOI
[69] Acoustic monitoring for conservation in tropical forests: examples from forest elephants, Methods in Ecology and Evolution, Volume 8 (2017) no. 10, pp. 1292-1301 | DOI
[70] Elicitation of a Beta Prior for Bayesian Inference in Clinical Trials, Biometrical Journal, Volume 50 (2008) no. 2, pp. 212-223 | DOI
[71] MCMCvis: Tools to visualize, manipulate, and summarize MCMC output, Journal of Open Source Software, Volume 3 (2018) no. 24, p. 640 | DOI
[72] Zooniverse (https://www.zooniverse.org/)
[73] Accounting for false positives and negatives in monitoring data from sensor networks and eDNA, Peer Community in Ecology, Volume 1, p. 100699 | DOI
[74] The Use of Automated Bioacoustic Recorders to Replace Human Wildlife Surveys: An Example Using Nightjars, PLoS ONE, Volume 9 (2014) no. 7, p. e102770 | DOI
Cited by Sources: