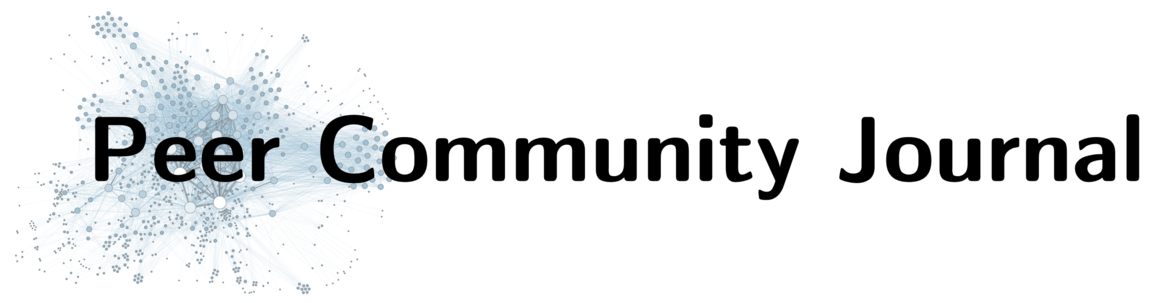
Section: Mathematical & Computational Biology
Topic:
Biophysics and computational biology,
Applied mathematics
Biology-Informed inverse problems for insect pests detection using pheromone sensors
Corresponding author(s): Labarthe, Simon (simon.labarthe@inrae.fr)
10.24072/pcjournal.520 - Peer Community Journal, Volume 5 (2025), article no. e19.
Get full text PDF Peer reviewed and recommended by PCI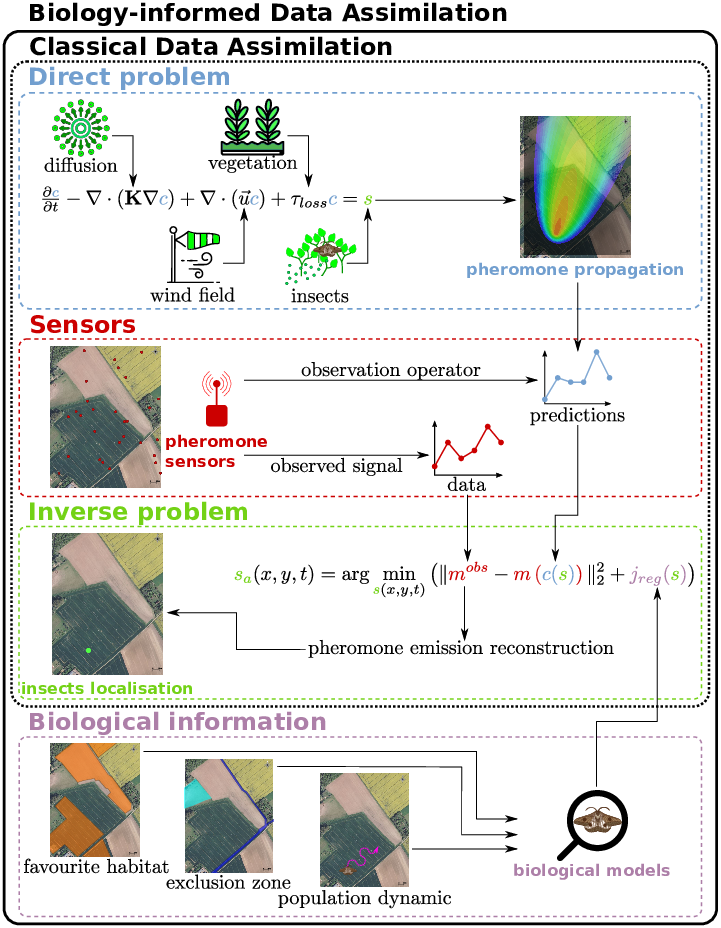
Most insects have the ability to modify the odor landscape in order to communicate with their conspecies during key phases of their life cycle such as reproduction. They release pheromones in their nearby environment, volatile compounds that are detected by insects of the same species with exceptional specificity and sensitivity. Efficient pheromone detection is then an interesting lever for insect pest management in a precision agroecological culture context. A precise and early detection of pests using pheromone sensors offers a strategy for pest management before infestation. In this paper, we develop a biology-informed inverse problem framework that leverages temporal signals from a pheromone sensor network to build insect presence maps. Prior biological knowledge is introduced in the inverse problem by the mean of a specific penalty, using population dynamics PDE residuals. We benchmark the biological-informed penalty with other regularization terms such as Tikhonov, LASSO or composite penalties in a simplified toy model. We use classical comparison criteria, such as target reconstruction error, or Jaccard distance on pest presence-absence. But we also use more task-specific criteria such as the number of informative sensors during inference. Finally, the inverse problem is solved in a realistic context of pest infestation in an agricultural landscape by the fall armyworm (Spodoptera frugiperda).
Type: Research article
Malou, Thibault 1; Parisey, Nicolas 2; Adamczyk-Chauvat, Katarzyna 1; Vergu, Elisabeta 1; Laroche, Béatrice 1, 3; Calatayud, Paul-André 4, 5; Lucas, Philippe 6; Labarthe, Simon 1, 7, 8
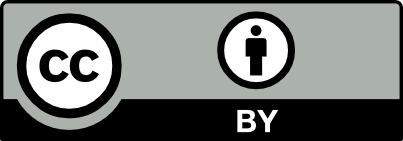
@article{10_24072_pcjournal_520, author = {Malou, Thibault and Parisey, Nicolas and Adamczyk-Chauvat, Katarzyna and Vergu, Elisabeta and Laroche, B\'eatrice and Calatayud, Paul-Andr\'e and Lucas, Philippe and Labarthe, Simon}, title = {Biology-Informed inverse problems for insect pests detection using pheromone sensors}, journal = {Peer Community Journal}, eid = {e19}, publisher = {Peer Community In}, volume = {5}, year = {2025}, doi = {10.24072/pcjournal.520}, language = {en}, url = {https://peercommunityjournal.org/articles/10.24072/pcjournal.520/} }
TY - JOUR AU - Malou, Thibault AU - Parisey, Nicolas AU - Adamczyk-Chauvat, Katarzyna AU - Vergu, Elisabeta AU - Laroche, Béatrice AU - Calatayud, Paul-André AU - Lucas, Philippe AU - Labarthe, Simon TI - Biology-Informed inverse problems for insect pests detection using pheromone sensors JO - Peer Community Journal PY - 2025 VL - 5 PB - Peer Community In UR - https://peercommunityjournal.org/articles/10.24072/pcjournal.520/ DO - 10.24072/pcjournal.520 LA - en ID - 10_24072_pcjournal_520 ER -
%0 Journal Article %A Malou, Thibault %A Parisey, Nicolas %A Adamczyk-Chauvat, Katarzyna %A Vergu, Elisabeta %A Laroche, Béatrice %A Calatayud, Paul-André %A Lucas, Philippe %A Labarthe, Simon %T Biology-Informed inverse problems for insect pests detection using pheromone sensors %J Peer Community Journal %D 2025 %V 5 %I Peer Community In %U https://peercommunityjournal.org/articles/10.24072/pcjournal.520/ %R 10.24072/pcjournal.520 %G en %F 10_24072_pcjournal_520
Malou, Thibault; Parisey, Nicolas; Adamczyk-Chauvat, Katarzyna; Vergu, Elisabeta; Laroche, Béatrice; Calatayud, Paul-André; Lucas, Philippe; Labarthe, Simon. Biology-Informed inverse problems for insect pests detection using pheromone sensors. Peer Community Journal, Volume 5 (2025), article no. e19. doi : 10.24072/pcjournal.520. https://peercommunityjournal.org/articles/10.24072/pcjournal.520/
PCI peer reviews and recommendation, and links to data, scripts, code and supplementary information: 10.24072/pci.mcb.100313
Conflict of interest of the recommender and peer reviewers:
The recommender in charge of the evaluation of the article and the reviewers declared that they have no conflict of interest (as defined in the code of conduct of PCI) with the authors or with the content of the article.
[1] Sparse Optimization for Inverse Problems in Atmospheric Modelling, Environmental Modelling & Software, Volume 79 (2016), pp. 256-266 | DOI
[2] Statistical Inference for Random T-tessellations Models. Application to Agricultural Landscape Modeling, Annals of the Institute of Statistical Mathematics, Volume 76 (2024) no. 3, pp. 447-479 | DOI
[3] Introduction to the Principles and Methods of Data Assimilation in the Geosciences, 2014
[4] Modelling Mate-Finding Allee Effects and Populations Dynamics, with Applications in Pest Control, Population Ecology, Volume 51 (2009), pp. 445-458 | DOI
[5] Aggregation and the competitive exclusion principle, Journal of Theoretical Biology, Volume 136 (1989) no. 1, pp. 57-66 | DOI
[6] Insect Odorscapes: From Plant Volatiles to Natural Olfactory Scenes, Frontiers in Physiology, Volume 10 (2019), p. 972 | DOI
[7] Increase in Crop Losses to Insect Pests in a Warming Climate, Science, Volume 361 (2018) no. 6405, pp. 916-919 | DOI
[8] Pest Risk Assessment of Spodoptera Frugiperda for the European Union, EFSA Journal, Volume 16 (2018) no. 8 | DOI
[9] A Theoretical Model for Particulate Transport from an Elevated Source in the Atmosphere, IMA Journal of Applied Mathematics, Volume 27 (1981) no. 3, pp. 359-371 | DOI
[10] The Wave of Advance of Advantageous Genes, Annals of eugenics, Volume 7 (1937) no. 4, pp. 355-369
[11] Local vs. Non-Local Interactions in Population Dynamics, Journal of Mathematical Biology (1989) | DOI
[12] Rheomergy: Collective Behaviour Mediated by Active Flow-Based Recruitment, Proceedings of the Royal Society A, Volume 479 (2023) no. 2269, p. 20220470 | DOI
[13] Precision Agriculture and Food Security, Science, Volume 327 (2010) no. 5967, pp. 828-831 | DOI
[14] Pattern and Waves for a Model in Population Dynamics with Nonlocal Consumption of Resources, Mathematical Modelling of Natural Phenomena, Volume 1 (2006) no. 1, pp. 63-80 | DOI
[15] Scientific Review of the Impact of Climate Change on Plant Pests, FAO on behalf of the IPPC Secretariat, 2021 | DOI
[16] Inverse Problems for Partial Differential Equations, Applied Mathematical Sciences, 127, Springer International Publishing, Cham, 2017 | DOI
[17] Iterative Regularization Methods for Nonlinear Ill-Posed Problems, 6, Walter de Gruyter, 2008 | DOI
[18] Local Movement in Herbivorous Insects: Applying a Passive Diffusion Model to Mark-Recapture Field Experiments, Oecologia, Volume 57 (1983) no. 3, pp. 322-327 | DOI
[19] Initiation of Slime Mold Aggregation Viewed as an Instability, Journal of Theoretical Biology, Volume 26 (1970) no. 3, pp. 399-415 | DOI
[20] Model for Chemotaxis, Journal of Theoretical Biology, Volume 30 (1971) no. 2, pp. 225-234 | DOI
[21] An Ultrasensitive Electrochemical Impedance-Based Biosensor Using Insect Odorant Receptors to Detect Odorants, Biosensors and Bioelectronics, Volume 126 (2019), pp. 207-213 | DOI
[22] Variational Algorithms for Analysis and Assimilation of Meteorological Observations: Theoretical Aspects, Tellus A: Dynamic Meteorology and Oceanography, Volume 38 (1986) no. 2, pp. 97-110 | DOI
[23] Dispersion modeling of air pollutants in the atmosphere: a review, Open Geosciences, Volume 6 (2014) no. 3 | DOI
[24] Optimal Control of Systems Governed by Partial Differential Equations, 170, Springer Verlag, 1971
[25] Olfactory Biosensor Using Odorant-Binding Proteins from Honeybee: Ligands of Floral Odors and Pheromones Detection by Electrochemical Impedance, Sensors and Actuators B: Chemical, Volume 193 (2014), pp. 420-427 | DOI
[26] An Inverse Gaussian Plume Approach for Estimating Atmospheric Pollutant Emissions from Multiple Point Sources, Atmospheric Environment, Volume 44 (2010) no. 8, pp. 1097-1107 | DOI
[27] BI-DA-companion-code, Zenodo (2024) | DOI
[28] Pherosensor-Toolbox, Zenodo, 2024 | DOI
[29] Pherosensor-toolbox: a Python package for Biology-Informed Data Assimilation, Journal of Open Source Software, Volume 9 (2024) no. 101, p. 6863 | DOI
[30] Using Insect Electroantennogram Sensors on Autonomous Robots for Olfactory Searches, Journal of Visualized Experiments (2014) no. 90, p. 51704 | DOI
[31] Empirical atmospheric deposition parameters—A survey, Atmospheric Environment, Volume 13 (1979) no. 5, pp. 571-585 | DOI
[32] Behavioral Ecology of Pheromone-Mediated Communication in Moths and Its Importance in the Use of Pheromone Traps, Annual Review of Entomology, Volume 36 (1991) no. 1, pp. 407-430 | DOI
[33] A Novel Bio-Engineering Approach to Generate an Eminent Surface-Functionalized Template for Selective Detection of Female Sex Pheromone of Helicoverpa Armigera, Scientific Reports, Volume 6 (2016) no. 1, p. 37355 | DOI
[34] Mathematical Biology: I. An Introduction, Springer, 2002
[35] Locating a Compact Odor Source Using a Four-Channel Insect Electroantennogram Sensor, Bioinspiration & Biomimetics, Volume 6 (2011) no. 1, p. 016002 | DOI
[36] Tracking the Sex Pheromone of Codling Moth against a Background of Host Volatiles with an Electronic Nose, Crop Protection, Volume 27 (2008) no. 10, pp. 1295-1302 | DOI
[37] Lectures on Convex Optimization, 137, Springer, 2018 | DOI
[38] Modelling Fluctuating Populations, John Wiley, 1982
[39] Crop Losses to Pests, The Journal of Agricultural Science, Volume 144 (2006) no. 1, pp. 31-43 | DOI
[40] Dynamical Aspects of Animal Grouping: Swarms, Schools, Flocks, and Herds, Advances in Biophysics, Volume 22 (1986), pp. 1-94 | DOI
[41] Atmospheric Turbulence. Models and Methods for Engineering Applications, Wiley, New York, 1984
[42] Proximal Algorithms, Foundations and trends in Optimization, Volume 1 (2014) no. 3, pp. 127-239 | DOI
[43] Optimal Spatial Monitoring of Populations Described by Reaction–Diffusion Models, Journal of Theoretical Biology, Volume 534 (2022), p. 110976 | DOI
[44] Light-Weight Portable Electroantennography Device as a Future Field-Based Tool for Applied Chemical Ecology, Journal of Chemical Ecology, Volume 46 (2020) no. 7, pp. 557-566 | DOI
[45] Transport Equations in Biology, Birkhäuser Basel, 2006 | DOI
[46] Application of Adjoint Tracer Transport Equations for Evaluating Source Parameters, Atmospheric Environment, Volume 32 (1998) no. 17, pp. 3039-3050 | DOI
[47] Physics-Informed Neural Networks: A Deep Learning Framework for Solving Forward and Inverse Problems Involving Nonlinear Partial Differential Equations, Journal of Computational Physics, Volume 378 (2019), pp. 686-707 | DOI
[48] An Objective Analysis Based on the Variational Method, Journal of the Meteorological Society of Japan. Ser. II, Volume 36 (1958) no. 3, pp. 77-88 | DOI
[49] The Global Burden of Pathogens and Pests on Major Food Crops, Nature Ecology & Evolution, Volume 3 (2019) no. 3, pp. 430-439 | DOI
[50] Spatial Distribution of Dispersing Animals, Journal of mathematical biology, Volume 9 (1980), pp. 85-96 | DOI
[51] Spatial Segregation of Interacting Species, Journal of Theoretical Biology, Volume 79 (1979) no. 1, pp. 83-99 | DOI
[52] Random Dispersal in Theoretical Populations, Biometrika, Volume 38 (1951) no. 1/2, pp. 196-218 | DOI
[53] A Review of Current Issues in Air Pollution Modeling and Simulation, Computational Geosciences, Volume 11 (2007), pp. 159-181 | DOI
[54] Bio-Inspired Sensor for Insect Pheromone Analysis Based on Polyaniline Functionalized AFM Cantilever Sensor, Sensors and Actuators B: Chemical, Volume 191 (2014), pp. 643-649 | DOI
[55] Selecting and Interpreting Measures of Thematic Classification Accuracy, Remote Sensing of Environment, Volume 62 (1997) no. 1, pp. 77-89 | DOI
[56] The Mathematics of Atmospheric Dispersion Modeling, SIAM Review, Volume 53 (2011) no. 2, pp. 349-372 | DOI
[57] Mathematical modeling of population dynamics with Allee effect, Nonlinear Dynamics, Volume 85 (2016) no. 1, pp. 1-12 | DOI
[58] Mathematical Models for the Aedes Aegypti Dispersal Dynamics: Travelling Waves by Wing and Wind, Bulletin of mathematical Biology, Volume 67 (2005), pp. 509-528 | DOI
[59] Towards accurate inference of insect presence landscapes from pheromone sensor networks, Peer Community in Mathematical and Computational Biology (2025) | DOI
[60] Inverse Problem Theory and Methods for Model Parameter Estimation, 89, SIAM, 2005 | DOI
[61] Regression Shrinkage and Selection via the Lasso, Journal of the Royal Statistical Society: Series B (Methodological), Volume 58 (1996) no. 1, pp. 267-288 | DOI
[62] Model-Error Estimation in 4D-Var, Quarterly Journal of the Royal Meteorological Society, Volume 133 (2007) no. 626, pp. 1267-1280 | DOI
[63] Sex Pheromone of Fall Armyworm,Spodoptera Frugiperda (J.E. Smith): Identification of Components Critical to Attraction in the Field, Journal of Chemical Ecology, Volume 12 (1986) no. 9, pp. 1909-1926 | DOI
[64] Laboratory characterization of metal‐oxide sensors intended for in situ analyses of pheromones – SOMMSA approach, physica status solidi (a), Volume 209 (2012) no. 5, pp. 935-939 | DOI
[65] Systems Biology Informed Deep Learning for Inferring Parameters and Hidden Dynamics, PLOS Computational Biology, Volume 16 (2020) no. 11, p. e1007575 | DOI
Cited by Sources: