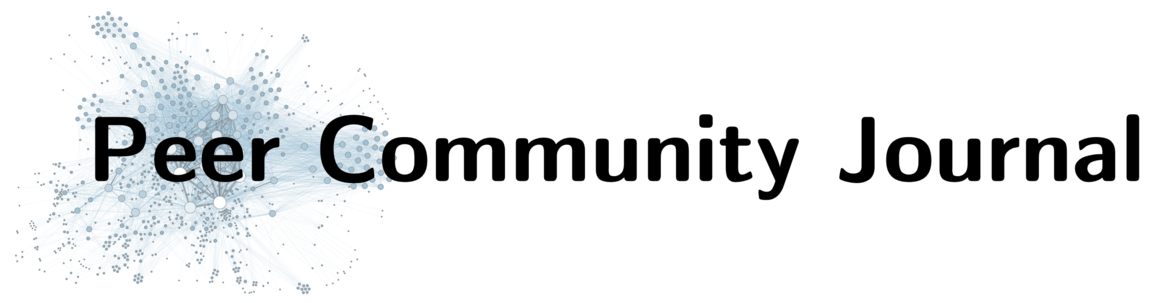
Section: Mathematical & Computational Biology
Topic: Biophysics and computational biology, Genetics/Genomics
HMMploidy: inference of ploidy levels from short-read sequencing data
10.24072/pcjournal.178 - Peer Community Journal, Volume 2 (2022), article no. e60.
Get full text PDF Peer reviewed and recommended by PCI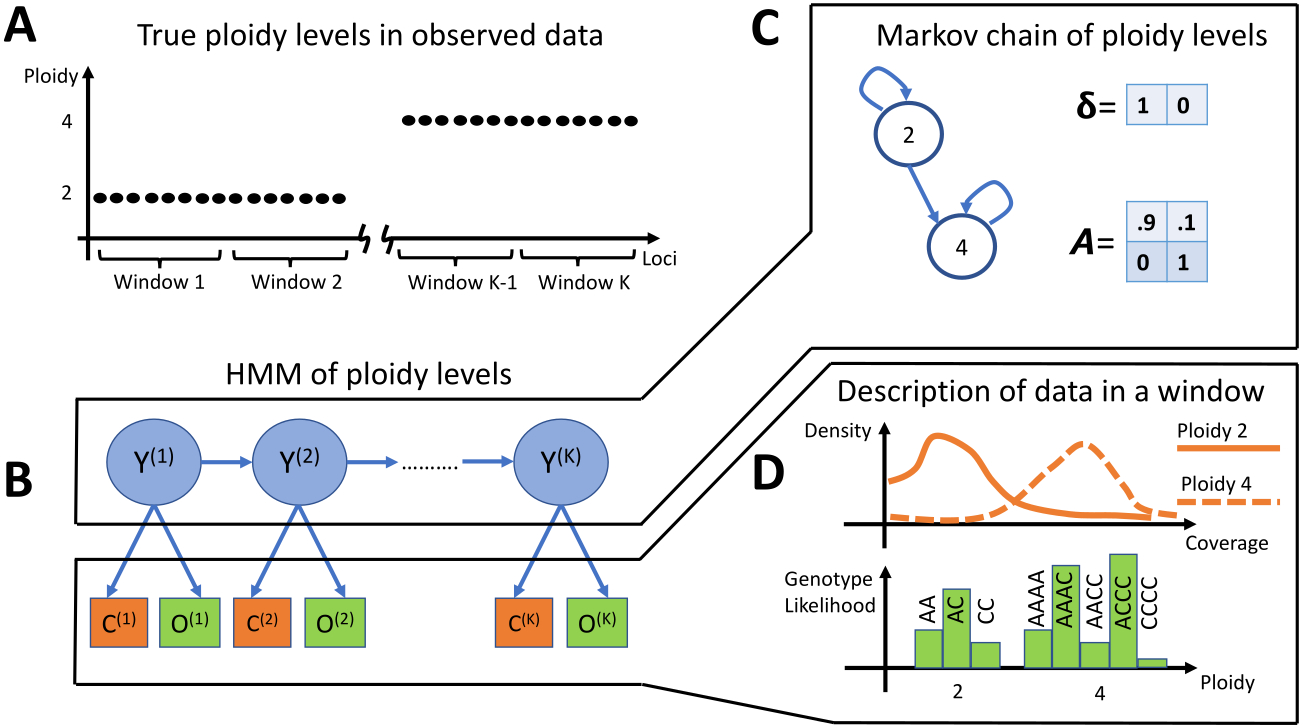
The inference of ploidy levels from genomic data is important to understand molecular mechanisms underpinning genome evolution. However, current methods based on allele frequency and sequencing depth variation do not have power to infer ploidy levels at low- and mid-depth sequencing data, as they do not account for data uncertainty. Here we introduce HMMploidy, a novel tool that leverages the information from multiple samples and combines the information from sequencing depth and genotype likelihoods. We demonstrate that HMMploidy outperforms existing methods in most tested scenarios, especially at low-depth with large sample size. We apply HMMploidy to sequencing data from the pathogenic fungus Cryptococcus neoformans and retrieve pervasive patterns of aneuploidy, even when artificially downsampling the sequencing data. We envisage that HMMploidy will have wide applicability to low-depth sequencing data from polyploid and aneuploid species.
Type: Software tool
Soraggi, Samuele 1, 2; Rhodes, Johanna 3; Altinkaya, Isin 2, 4, 5; Tarrant, Oliver 2; Balloux, Francois 6; Fisher, Matthew C 3; Fumagalli, Matteo 2, 7
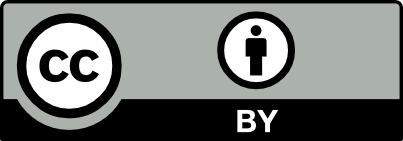
@article{10_24072_pcjournal_178, author = {Soraggi, Samuele and Rhodes, Johanna and Altinkaya, Isin and Tarrant, Oliver and Balloux, Francois and Fisher, Matthew C and Fumagalli, Matteo}, title = {HMMploidy: inference of ploidy levels from short-read sequencing data}, journal = {Peer Community Journal}, eid = {e60}, publisher = {Peer Community In}, volume = {2}, year = {2022}, doi = {10.24072/pcjournal.178}, url = {https://peercommunityjournal.org/articles/10.24072/pcjournal.178/} }
TY - JOUR AU - Soraggi, Samuele AU - Rhodes, Johanna AU - Altinkaya, Isin AU - Tarrant, Oliver AU - Balloux, Francois AU - Fisher, Matthew C AU - Fumagalli, Matteo TI - HMMploidy: inference of ploidy levels from short-read sequencing data JO - Peer Community Journal PY - 2022 VL - 2 PB - Peer Community In UR - https://peercommunityjournal.org/articles/10.24072/pcjournal.178/ DO - 10.24072/pcjournal.178 ID - 10_24072_pcjournal_178 ER -
%0 Journal Article %A Soraggi, Samuele %A Rhodes, Johanna %A Altinkaya, Isin %A Tarrant, Oliver %A Balloux, Francois %A Fisher, Matthew C %A Fumagalli, Matteo %T HMMploidy: inference of ploidy levels from short-read sequencing data %J Peer Community Journal %D 2022 %V 2 %I Peer Community In %U https://peercommunityjournal.org/articles/10.24072/pcjournal.178/ %R 10.24072/pcjournal.178 %F 10_24072_pcjournal_178
Soraggi, Samuele; Rhodes, Johanna; Altinkaya, Isin; Tarrant, Oliver; Balloux, Francois; Fisher, Matthew C; Fumagalli, Matteo. HMMploidy: inference of ploidy levels from short-read sequencing data. Peer Community Journal, Volume 2 (2022), article no. e60. doi : 10.24072/pcjournal.178. https://peercommunityjournal.org/articles/10.24072/pcjournal.178/
PCI peer reviews and recommendation, and links to data, scripts, code and supplementary information: 10.24072/pci.mcb.100010
Conflict of interest of the recommender and peer reviewers:
The recommender in charge of the evaluation of the article and the reviewers declared that they have no conflict of interest (as defined in the code of conduct of PCI) with the authors or with the content of the article.
[1] Differential expression analysis for sequence count data, Genome Biology, Volume 11 (2010) no. 10 | DOI
[2] ploidyNGS: visually exploring ploidy with Next Generation Sequencing data, Bioinformatics, Volume 33 (2017) no. 16, pp. 2575-2576 | DOI
[3] Tetraploidy accelerates adaption under drug-selection in a fungal pathogen, bioRxiv, 2021 | DOI
[4] AbsCN-seq: a statistical method to estimate tumor purity, ploidy and absolute copy numbers from next-generation sequencing data, Bioinformatics, Volume 30 (2014) no. 8, pp. 1056-1063 | DOI
[5] Context is everything: aneuploidy in cancer, Nature Reviews Genetics, Volume 21 (2019) no. 1, pp. 44-62 | DOI
[6] Pattern recognition and machine learning, Springer, 2006
[7] Inference in Hidden Markov Models, Springer Series in Statistics, Springer New York, New York, NY, 2005 | DOI
[8] Statistical inference, Thomson Learning, 2002
[9] Departure from Hardy Weinberg Equilibrium and Genotyping Error, Frontiers in Genetics, Volume 8 (2017) | DOI
[10] Size Does Matter: Why Polyploid Tumor Cells are Critical Drug Targets in the War on Cancer, Frontiers in Oncology, Volume 4 (2014) | DOI
[11] The Causes and Consequences of Polyploidy in Normal Development and Cancer, Annual Review of Cell and Developmental Biology, Volume 27 (2011) no. 1, pp. 585-610 | DOI
[12] Chromosomal Copy Number Variation, Selection and Uneven Rates of Recombination Reveal Cryptic Genome Diversity Linked to Pathogenicity, PLoS Genetics, Volume 9 (2013) no. 8 | DOI
[13] Sequenza: allele-specific copy number and mutation profiles from tumor sequencing data, Annals of Oncology, Volume 26 (2015) no. 1, pp. 64-70 | DOI
[14] Polyploidy: A Biological Force From Cells to Ecosystems, Trends in Cell Biology, Volume 30 (2020) no. 9, pp. 688-694 | DOI
[15] Dynamic genome plasticity during unisexual reproduction in the human fungal pathogen Cryptococcus deneoformans, PLOS Genetics, Volume 17 (2021) no. 11 | DOI
[16] Quantifying Population Genetic Differentiation from Next-Generation Sequencing Data, Genetics, Volume 195 (2013) no. 3, pp. 979-992 | DOI
[17] ngsTools: methods for population genetics analyses from next-generation sequencing data, Bioinformatics, Volume 30 (2013) no. 10, pp. 1486-1487 | DOI
[18] Haplotype-based variant detection from short-read sequencing, arXiv (2012) | DOI
[19] Mendelian Proportions in a Mixed Population, Science, Volume 28 (1908) no. 706, pp. 49-50 | DOI
[20] Detecting selection-induced departures from Hardy-Weinberg proportions, Genetics Selection Evolution, Volume 41 (2009) no. 1 | DOI
[21] Advancements in Next-Generation Sequencing, Annual Review of Genomics and Human Genetics, Volume 17 (2016) no. 1, pp. 95-115 | DOI
[22] Temporal Pattern Generation Using Hidden Markov Model Based Unsupervised Classification, Advances in Intelligent Data Analysis, Springer Berlin Heidelberg, Berlin, Heidelberg, 1999, pp. 245-256 | DOI
[23] The Sequence Alignment/Map format and SAMtools, Bioinformatics, Volume 25 (2009) no. 16, pp. 2078-2079 | DOI
[24] The Genome of the Basidiomycetous Yeast and Human Pathogen Cryptococcus neoformans, Science, Volume 307 (2005) no. 5713, pp. 1321-1324 | DOI
[25] Dose Response Effect of High‐Dose Fluconazole for HIV‐Associated Cryptococcal Meningitis in Southwestern Uganda, Clinical Infectious Diseases, Volume 47 (2008) no. 12, pp. 1556-1561 | DOI
[26] A beginner's guide to low‐coverage whole genome sequencing for population genomics, Molecular Ecology, Volume 30 (2021) no. 23, pp. 5966-5993 | DOI
[27] Cryptococcus: from environmental saprophyte to global pathogen, Nature Reviews Microbiology, Volume 14 (2016) no. 2, pp. 106-117 | DOI
[28] The Genome Analysis Toolkit: A MapReduce framework for analyzing next-generation DNA sequencing data, Genome Research, Volume 20 (2010) no. 9, pp. 1297-1303 | DOI
[29] Sequencing technologies — the next generation, Nature Reviews Genetics, Volume 11 (2010) no. 1, pp. 31-46 | DOI
[30] Ploidy variation as an adaptive mechanism in human pathogenic fungi, Seminars in Cell & Developmental Biology, Volume 24 (2013) no. 4, pp. 339-346 | DOI
[31] Genotype and SNP calling from next-generation sequencing data, Nature Reviews Genetics, Volume 12 (2011) no. 6, pp. 443-451 | DOI
[32] Comparative Genomics of Serial Isolates of Cryptococcus neoformans Reveals Gene Associated With Carbon Utilization and Virulence, G3 Genes|Genomes|Genetics, Volume 3 (2013) no. 4, pp. 675-686 | DOI
[33] A tutorial on hidden Markov models and selected applications in speech recognition, Proceedings of the IEEE, Volume 77 (1989) no. 2, pp. 257-286 | DOI
[34] A Population Genomics Approach to Assessing the Genetic Basis of Within-Host Microevolution Underlying Recurrent Cryptococcal Meningitis Infection, G3 Genes|Genomes|Genetics, Volume 7 (2017) no. 4, pp. 1165-1176 | DOI
[35] Illuminating Choices for Library Prep: A Comparison of Library Preparation Methods for Whole Genome Sequencing of Cryptococcus neoformans Using Illumina HiSeq, PLoS ONE, Volume 9 (2014) no. 11 | DOI
[36] Tracing Genetic Exchange and Biogeography of Cryptococcus neoformans var. grubii at the Global Population Level, Genetics, Volume 207 (2017) no. 1, pp. 327-346 | DOI
[37] The polyploidy and its key role in plant breeding, Planta, Volume 243 (2016) no. 2, pp. 281-296 | DOI
[38] Azole Heteroresistance in Cryptococcus neoformans: Emergence of Resistant Clones with Chromosomal Disomy in the Mouse Brain during Fluconazole Treatment, Antimicrobial Agents and Chemotherapy, Volume 57 (2013) no. 10, pp. 5127-5130 | DOI
[39] HMMploidy, Zenodo, 2022 | DOI
[40] HMMploidy, OSF, 2022 | DOI
[41] HMMploidy: inference of ploidy levels from short-read sequencing data, bioRxiv (2022) no. 2021.06.29.450340 | DOI
[42] Dynamic ploidy changes drive fluconazole resistance in human cryptococcal meningitis, Journal of Clinical Investigation, Volume 129 (2019) no. 3, pp. 999-1014 | DOI
[43] Practical low-coverage genomewide sequencing of hundreds of individually barcoded samples for population and evolutionary genomics in nonmodel species, Molecular Ecology Resources, Volume 17 (2017) no. 2, pp. 194-208 | DOI
[44] The evolutionary significance of polyploidy, Nature Reviews Genetics, Volume 18 (2017) no. 7, pp. 411-424 | DOI
[45] From FastQ Data to High‐Confidence Variant Calls: The Genome Analysis Toolkit Best Practices Pipeline, Current Protocols in Bioinformatics, Volume 43 (2013) no. 1 | DOI
[46] Deletion‐bias in DNA double‐strand break repair differentially contributes to plant genome shrinkage, New Phytologist, Volume 214 (2017) no. 4, pp. 1712-1721 | DOI
[47] Über den Nachweis der Vererbung beim Menschen, Ver. Vaterl. Naturkd. Württemb, Volume 64 (1908), pp. 369-382
[48] nQuire: a statistical framework for ploidy estimation using next generation sequencing, BMC Bioinformatics, Volume 19 (2018) no. 1 | DOI
[49] Rational Inferences about Departures from Hardy-Weinberg Equilibrium, The American Journal of Human Genetics, Volume 76 (2005) no. 6, pp. 967-986 | DOI
[50] The frequency of polyploid speciation in vascular plants, Proceedings of the National Academy of Sciences, Volume 106 (2009) no. 33, pp. 13875-13879 | DOI
[51] Adaptation to Fluconazole via Aneuploidy Enables Cross-Adaptation to Amphotericin B and Flucytosine in Cryptococcus neoformans, Microbiology Spectrum, Volume 9 (2021) no. 2 | DOI
[52] Cellular Stress Associated with Aneuploidy, Developmental Cell, Volume 44 (2018) no. 4, pp. 420-431 | DOI
Cited by Sources: