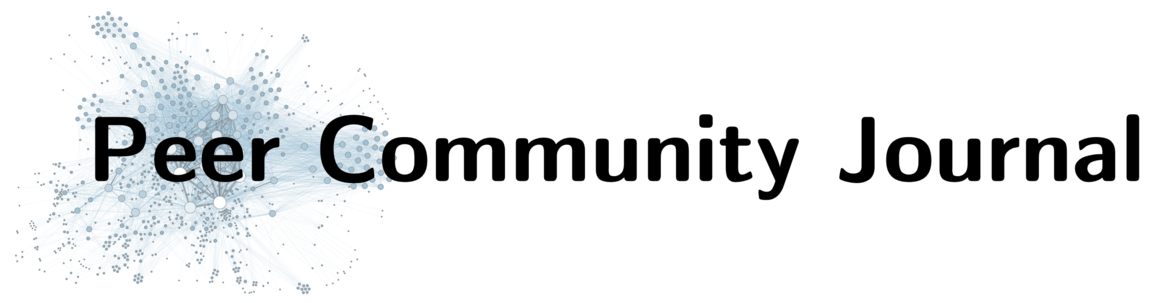
Section: Ecology
Topic:
Ecology,
Population biology,
Statistics
Efficient sampling designs to assess biodiversity spatial autocorrelation: should we go fractal?
10.24072/pcjournal.454 - Peer Community Journal, Volume 4 (2024), article no. e76.
Get full text PDF Peer reviewed and recommended by PCI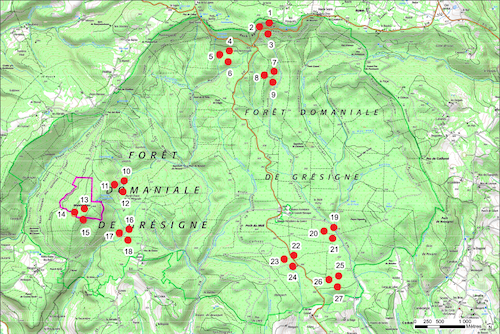
Quantifying the autocorrelation range of species distribution in space is necessary for applied ecological questions, like implementing protected area networks or monitoring programs. However, the power of spatial sampling designs to estimate this range is negatively related with other objectives such as estimating environmental effects acting upon species distribution. Mixing random sampling points and systematic grid (‘hybrid’ designs) is a classic solution to make a trade-off. However, fractal designs (i.e. self-similar designs with well-identified scales) could make an even better compromise, because they cover a wide array of possible autocorrelation range values across scales. Using maximum likelihood estimation in an optimal design of experiments approach, we compared errors of hybrid and fractal designs when simultaneously estimating an effect acting upon a response variable and the residual autocorrelation range. We found that Pareto-optimal sampling strategies depended on the feasible grid mesh size (FGMS) over the study area, given the sampling budget. When the FMGS was shorter than expected autocorrelation range values, grid design was the best option on all criteria. When the FMGS was around or larger than expected autocorrelation range values, the choice of designs depended on the effect under study. Fractal designs outperformed hybrid designs when studying the effect of a monotonic environmental gradient across space, while grid design was more efficient for other types of question. Beyond the niche identified in our analysis, fractal designs may also appear interesting when studying response variables with more heterogeneous spatial structure across scales, and when considering more practical criteria of performance such as the distance needed to cover the design.
Type: Research article
Laroche, Fabien 1
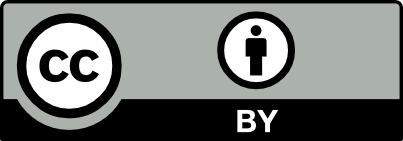
@article{10_24072_pcjournal_454, author = {Laroche, Fabien}, title = {Efficient sampling designs to assess biodiversity spatial autocorrelation: should we go fractal?}, journal = {Peer Community Journal}, eid = {e76}, publisher = {Peer Community In}, volume = {4}, year = {2024}, doi = {10.24072/pcjournal.454}, language = {en}, url = {https://peercommunityjournal.org/articles/10.24072/pcjournal.454/} }
TY - JOUR AU - Laroche, Fabien TI - Efficient sampling designs to assess biodiversity spatial autocorrelation: should we go fractal? JO - Peer Community Journal PY - 2024 VL - 4 PB - Peer Community In UR - https://peercommunityjournal.org/articles/10.24072/pcjournal.454/ DO - 10.24072/pcjournal.454 LA - en ID - 10_24072_pcjournal_454 ER -
%0 Journal Article %A Laroche, Fabien %T Efficient sampling designs to assess biodiversity spatial autocorrelation: should we go fractal? %J Peer Community Journal %D 2024 %V 4 %I Peer Community In %U https://peercommunityjournal.org/articles/10.24072/pcjournal.454/ %R 10.24072/pcjournal.454 %G en %F 10_24072_pcjournal_454
Laroche, Fabien. Efficient sampling designs to assess biodiversity spatial autocorrelation: should we go fractal?. Peer Community Journal, Volume 4 (2024), article no. e76. doi : 10.24072/pcjournal.454. https://peercommunityjournal.org/articles/10.24072/pcjournal.454/
PCI peer reviews and recommendation, and links to data, scripts, code and supplementary information: 10.24072/pci.ecology.100536
Conflict of interest of the recommender and peer reviewers:
The recommender in charge of the evaluation of the article and the reviewers declared that they have no conflict of interest (as defined in the code of conduct of PCI) with the authors or with the content of the article.
[1] Fisher information and maximum-likelihood estimation of covariance parameters in Gaussian stochastic processes, Canadian Journal of Statistics, Volume 26 (1998) no. 1, pp. 127-137 | DOI
[2] Sampling in ecology and evolution - bridging the gap between theory and practice, Ecography, Volume 33 (2010) no. 6, pp. 1028-1037 | DOI
[3] Optimising vegetation monitoring. A case study in A French lowland forest, Environmental Monitoring and Assessment, Volume 141 (2008) no. 1-3, pp. 19-25 | DOI
[4] Valid auto-models for spatially autocorrelated occupancy and abundance data, Methods in Ecology and Evolution, Volume 6 (2015) no. 10, pp. 1137-1149 | DOI
[5] Designing a benthic monitoring programme with multiple conflicting objectives, Methods in Ecology and Evolution, Volume 3 (2012) no. 3, pp. 526-536 | DOI
[6] Using Moment Equations to Understand Stochastically Driven Spatial Pattern Formation in Ecological Systems, Theoretical Population Biology, Volume 52 (1997) no. 3, pp. 179-197 | DOI
[7] Spatial autocorrelation patterns of stream invertebrates: exogenous and endogenous factors, Journal of Biogeography, Volume 39 (2011) no. 1, pp. 56-68 | DOI
[8] The incidence function approach to modeling of metapopula- tion dynamics In: In: Modeling spatiotemporal dynamics in ecology, Springer-Verlag, Berlin (1998), pp. 167-188
[9] Geostatistics, Statistics for Spatial Data, John Wiley & Sons, Ltd, 1993, pp. 27-104 | DOI
[10] Methods to account for spatial autocorrelation in the analysis of species distributional data: a review, Ecography, Volume 30 (2007) no. 5, pp. 609-628 | DOI
[11] Gene Dispersal and Spatial Genetic Structure, Evolution, Volume 51 (1997) no. 3, p. 672 | DOI
[12] A large-scale forest fragmentation experiment: the Stability of Altered Forest Ecosystems Project, Philosophical Transactions of the Royal Society B: Biological Sciences, Volume 366 (2011) no. 1582, pp. 3292-3302 | DOI
[13] Fractal geometry: mathematical foundations and applications, Wiley, Chichester, England, 2003 | DOI
[14] Measuring Spatial Aggregation in Binary Epidemics: Correlative Analysis and the Advantage of Fractal-Based Sampling, Phytopathology, Volume 94 (2004) no. 11, pp. 1215-1227 | DOI
[15] Spatial species-richness gradients across scales: a meta-analysis, Journal of Biogeography, Volume 36 (2009) no. 1, pp. 132-147 | DOI
[16] Computation of Multivariate Normal and t Probabilities, Lecture Notes in Statistics, Springer-Verlag, Heidelberg, 2009 | DOI
[17] Spatial patterns and autocorrelation challenges in ecological conservation, Peer Community in Ecology, Volume 1 (2024), p. 100536 | DOI
[18] Integrating gradient with scale in ecological and evolutionary studies, Ecology, Volume 104 (2023) no. 4, p. e3982 | DOI
[19] Optimal spatio-temporal hybrid sampling designs for ecological monitoring, Journal of Vegetation Science, Volume 20 (2009) no. 4, pp. 639-649 | DOI
[20] Epiphyte metapopulation dynamics are explained by species traits, connectivity, and patch dynamics, Ecology, Volume 93 (2012) no. 2, pp. 235-241 | DOI
[21] Causal assumptions and causal inference in ecological experiments, Trends in Ecology & Evolution, Volume 36 (2021) no. 12, pp. 1141-1152 | DOI
[22] Statistics and partitioning of species diversity, and similarity among multiple communities, Oikos, Volume 76 (1996) no. 1, pp. 5-13 | DOI
[23] Code used to generate and compare sampling design estimation error on effects and autocorrelation range in Laroche (2023), Zenodo, 2023 | DOI
[24] Appendix to "Efficient sampling designs to assess biodiversity spatial autocorrelation: should we go fractal?", Zeonodo, 2024 | DOI
[25] Spatial Autocorrelation: Trouble or New Paradigm?, Ecology, Volume 74 (1993) no. 6, pp. 1659-1673 | DOI
[26] Red‐shifts and red herrings in geographical ecology, Ecography, Volume 23 (2000) no. 1, pp. 101-113 | DOI
[27] The fractal geometry of nature, 173, WH freeman, New York, 1983
[28] Ten years of landscape genetics, Trends in Ecology & Evolution, Volume 28 (2013) no. 10, pp. 614-621 | DOI
[29] A fractal-based sampling design for ecological surveys quantifying beta-diversity, Methods in Ecology and Evolution, Volume 4 (2013) no. 1, pp. 63-72 | DOI
[30] Towards a unification of unified theories of biodiversity, Ecology Letters, Volume 13 (2010), pp. 627-642 | DOI
[31] Notes on continuous stochastic phenomena, Biometrika, Volume 37 (1950) no. 1-2, pp. 17-23 | DOI
[32] Collecting spatial data: optimum design of experiments for random fields, Springer, Berlin ; New York, 2007
[33] Optimal design for detecting dependencies with an application in spatial ecology, Environmetrics, Volume 23 (2012) no. 1, pp. 37-45 | DOI
[34] The distance decay of similarity in biogeography and ecology, Journal of Biogeography, Volume 26 (1999) no. 4, pp. 867-878 | DOI
[35] Population genetics, molecular markers and the study of dispersal in plants, Journal of Ecology, Volume 87 (1999) no. 4, pp. 551-568 | DOI
[36] Spatially balanced sampling methods are always more precise than random ones for estimating the size of aggregated populations, Methods in Ecology and Evolution, Volume 13 (2022) no. 12, pp. 2743-2756 | DOI
[37] An evaluation of patch connectivity measures, Ecological Applications, Volume 19 (2009) no. 5, pp. 1300-1310 | DOI
[38] R: A Language and Environment for Statistical Computing, R Foundation for Statistical Computing, Vienna, Austria, 2023
[39] A comparison of patch connectivity measures using data on invertebrates in hollow oaks, Ecography, Volume 33 (2010) no. 5, pp. 971-978 | DOI
[40] Monitoring temporal trends in spatially structured populations: how should sampling effort be allocated between space and time?, Ecography, Volume 34 (2011) no. 6, pp. 1040-1048 | DOI
[41] Disintegration of the ecological community, The American Naturalist, Volume 172 (2008) no. 6, pp. 741-750 | DOI
[42] Fractal triads efficiently sample ecological diversity and processes across spatial scales, Oikos, Volume 130 (2021) no. 12, pp. 2136-2147 | DOI
[43] Spatial autocorrelation in biology: 1. Methodology, Biological Journal of the Linnean Society, Volume 10 (1978) no. 2, pp. 199-228 | DOI
[44] Efficiency of spatial sampling designs in estimating abundance and species richness of carabids at the landscape level, Landscape Ecology, Volume 38 (2023) no. 4, pp. 919-932 | DOI
[45] From species distributions to meta-communities, Ecology Letters, Volume 18 (2015) no. 12, pp. 1321-1328 | DOI
[46] On the usage and measurement of landscape connectivity, Oikos, Volume 90 (2000) no. 1, pp. 7-19 | DOI
[47] New insights from fine-scale spatial genetic structure analyses in plant populations, Molecular Ecology, Volume 13 (2004) no. 4, pp. 921-935 | DOI
[48] Spatial sampling design for parameter estimation of the covariance function, Journal of Statistical Planning and Inference, Volume 134 (2005) no. 2, pp. 583-603 | DOI
[49] The virtual ecologist approach: simulating data and observers, Oikos, Volume 119 (2010) no. 4, pp. 622-635 | DOI
Cited by Sources: