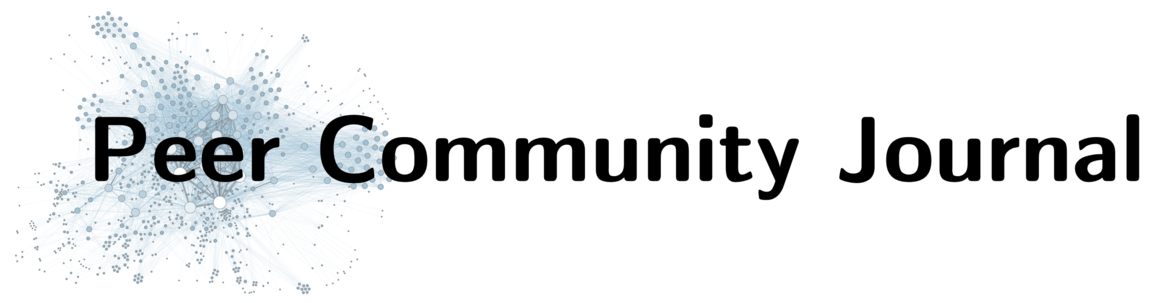
Section: Mathematical & Computational Biology
Topic:
Ecology,
Statistics
Proper account of auto-correlations improves decoding performances of state-space (semi) Markov models
Corresponding author(s): Bez, Nicolas (nicolas.bez@ird.fr)
10.24072/pcjournal.535 - Peer Community Journal, Volume 5 (2025), article no. e38.
Get full text PDF Peer reviewed and recommended by PCIState-space models are widely used in ecology to infer hidden behaviors. This study develops an extensive numerical simulation-estimation experiment to evaluate the state decoding accuracy of four simple state-space models. These models are obtained by combining different Markovian specifications (Markov and semi-Markov) for the hidden layer with the absence (model AR0) and presence (AR1) of auto-correlation for the observation layer. Model parameters are issued from two sets of real annotated trajectories. Three metrics are developed to help interpret model performance. The first is the Hellinger distance between Markov and semi-Markov sojourn time probability distributions. The second is sensitive to the overlap between the probability density functions of state-dependent variables (e.g., speed variables). The third quantifies the deterioration of the inference conditions between AR0 and AR1 formulations. It emerges that the most sensitive model choice concerns the auto-correlation of the random processes describing the state-dependent variables. Opting for the absence of auto-correlation in the model while the state-dependent variables are actually auto-correlated, is detrimental to state decoding performance. Regarding the hidden layer, imposing a Markov structure while the state process is semi-Markov (with negative Binomial sojourn times) does not impair the state decoding performances. The real-life estimates are consistent with our experimental finding that performance deteriorates when there are significant temporal correlations that are not accounted for in the model. In light of these findings, we recommend that researchers carefully consider the structure of the statistical model they suggest and confirm its alignment with the process being modeled, especially when considering the auto-correlation of observed variables.
Type: Research article
Bez, Nicolas 1; Gloaguen, Pierre 2; Etienne, Marie-Pierre 3; Lanco, Sophie 1; Joo, Rocío 4; Rivot, Etienne 5; Walker, Emily 6; Woillez, Mathieu 5; Mahévas, Stéphanie 1
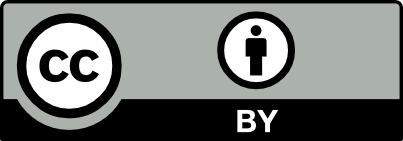
@article{10_24072_pcjournal_535, author = {Bez, Nicolas and Gloaguen, Pierre and Etienne, Marie-Pierre and Lanco, Sophie and Joo, Roc{\'\i}o and Rivot, Etienne and Walker, Emily and Woillez, Mathieu and Mah\'evas, St\'ephanie}, title = {Proper account of auto-correlations improves decoding performances of state-space (semi) {Markov} models}, journal = {Peer Community Journal}, eid = {e38}, publisher = {Peer Community In}, volume = {5}, year = {2025}, doi = {10.24072/pcjournal.535}, language = {en}, url = {https://peercommunityjournal.org/articles/10.24072/pcjournal.535/} }
TY - JOUR AU - Bez, Nicolas AU - Gloaguen, Pierre AU - Etienne, Marie-Pierre AU - Lanco, Sophie AU - Joo, Rocío AU - Rivot, Etienne AU - Walker, Emily AU - Woillez, Mathieu AU - Mahévas, Stéphanie TI - Proper account of auto-correlations improves decoding performances of state-space (semi) Markov models JO - Peer Community Journal PY - 2025 VL - 5 PB - Peer Community In UR - https://peercommunityjournal.org/articles/10.24072/pcjournal.535/ DO - 10.24072/pcjournal.535 LA - en ID - 10_24072_pcjournal_535 ER -
%0 Journal Article %A Bez, Nicolas %A Gloaguen, Pierre %A Etienne, Marie-Pierre %A Lanco, Sophie %A Joo, Rocío %A Rivot, Etienne %A Walker, Emily %A Woillez, Mathieu %A Mahévas, Stéphanie %T Proper account of auto-correlations improves decoding performances of state-space (semi) Markov models %J Peer Community Journal %D 2025 %V 5 %I Peer Community In %U https://peercommunityjournal.org/articles/10.24072/pcjournal.535/ %R 10.24072/pcjournal.535 %G en %F 10_24072_pcjournal_535
Bez, Nicolas; Gloaguen, Pierre; Etienne, Marie-Pierre; Lanco, Sophie; Joo, Rocío; Rivot, Etienne; Walker, Emily; Woillez, Mathieu; Mahévas, Stéphanie. Proper account of auto-correlations improves decoding performances of state-space (semi) Markov models. Peer Community Journal, Volume 5 (2025), article no. e38. doi : 10.24072/pcjournal.535. https://peercommunityjournal.org/articles/10.24072/pcjournal.535/
PCI peer reviews and recommendation, and links to data, scripts, code and supplementary information: 10.24072/pci.mcb.100316
Conflict of interest of the recommender and peer reviewers:
The recommender in charge of the evaluation of the article and the reviewers declared that they have no conflict of interest (as defined in the code of conduct of PCI) with the authors or with the content of the article.
[1] A multivariate approach to model skill assessment, Journal of Marine Systems, Volume 76 (2009) no. 1-2, pp. 83-94 | DOI
[2] Sampling rate and misidentification of Lévy and non-Lévy movement paths: comment, Ecology, Volume 92 (2011) no. 8, pp. 1699-1701 | DOI
[3] A guide to state–space modeling of ecological time series, Ecological Monographs, Volume 91 (2021) no. 4, p. e01470 | DOI
[4] An empirically parameterized individual based model of animal movement, perception, and memory, Ecological Modelling, Volume 251 (2013), pp. 158-172 | DOI
[5] Semi-Markov Chains and Hidden Semi-Markov Models toward Applications, Springer | DOI
[6] Scale-Invariant Movements of Fishermen: The Same Foraging Strategy as Natural Predators, Ecological Applications, Volume 17 (2007) no. 2, pp. 331-337 | DOI
[7] nicobez/H-S-MMMSA: MSA (v1.0.1), Zenodo (2024) | DOI
[8] nicobez/H-S-MMpaperfigures: figures (V1.0.0), Zenodo (2024) | DOI
[9] Fishing activity of tuna purse seiners estimated from vessel monitoring system (VMS) data, Canadian Journal of Fisheries and Aquatic Sciences, Volume 68 (2011) no. 11, pp. 1998-2010 | DOI
[10] Maximum Likelihood from Incomplete Data Via the EM Algorithm, Journal of the Royal Statistical Society: Series B (Methodological), Volume 39 (1977) no. 1, pp. 1-22 | DOI
[11] Path segmentation for beginners: an overview of current methods for detecting changes in animal movement patterns, Movement Ecology, Volume 4 (2016) no. 1, p. 21 | DOI
[12] Integrating vessel monitoring systems (VMS) data with daily catch data from logbooks to explore the spatial distribution of catch and effort at high resolution, ICES Journal of Marine Science, Volume 68 (2011) no. 1, pp. 245-252 | DOI
[13] Hidden Markov models: Pitfalls and opportunities in ecology, Methods in Ecology and Evolution, Volume 14 (2023) no. 1, pp. 43-56 | DOI
[14] An autoregressive model to describe fishing vessel movement and activity, Environmetrics, Volume 26 (2015) no. 1, pp. 17-28 | DOI
[15] Estimating Hidden Semi-Markov Chains from Discrete Sequences, Journal of Computational and Graphical Statistics, Volume 12 (2003) no. 3, p. 604
[16] Neue Begründung der Theorie quadratischer Formen von unendlichvielen Veränderlichen., Journal für die reine und angewandte Mathematik, Volume 1909 (1909) no. 136, pp. 210-271 | DOI
[17] Introduction to Hidden Semi-Markov Models, Cambridge University Press | DOI
[18] Bayesian Nonparametric Hidden Semi-Markov Models, Journal of Machine Learning Research, Volume 14 (2013) no. 20, pp. 673-701
[19] State-space models for bio-loggers: A methodological road map, Deep Sea Research Part II: Topical Studies in Oceanography, Volume 88-89 (2013), pp. 34-46 | DOI
[20] Hidden markov models: the best models for forager movements?, PloS one, Volume 8 (2013) no. 8, p. e71246 | DOI
[21] Mismatch between VMS data temporal resolution and fishing activity time scales, Fisheries Research, Volume 188 (2017), pp. 1-5 | DOI
[22] Flexible and practical modeling of animal telemetry data: hidden Markov models and extensions, Ecology, Volume 93 (2012) no. 11, pp. 2336-2342 | DOI
[23] Ergodicity and integral range, Journal of Microscopy, Volume 161 (1991), pp. 387-403 | DOI
[24] Skill assessment for coupled biological/physical models of marine systems, Journal of Marine Systems, Volume 76 (2009) no. 1-2, pp. 1-3 | DOI
[25] Worth the effort? A practical examination of random effects in hidden Markov models for animal telemetry data, Methods in Ecology and Evolution, Volume 12 (2021) no. 8, pp. 1475-1497 | DOI
[26] A general discrete-time modeling framework for animal movement using multistate random walks, Ecological Monographs, Volume 82 (2012) no. 3, pp. 335-349
[27] Uncovering ecological state dynamics with hidden Markov models, Ecology Letters, Volume 23 (2020) no. 12, pp. 1878-1903 | DOI
[28] Effect of temporal and spatial resolution on identification of fishing activities in small-scale fisheries using pots and traps, ICES Journal of Marine Science, Volume 76 (2019) no. 6, pp. 1601-1609 | DOI
[29] Extracting more out of relocation data: building movement models as mixtures of random walks, Ecology, Volume 85 (2004) no. 9, pp. 2436-2445 | DOI
[30] Human behavioral ecology: current research and future prospects, Behavioral Ecology, Volume 24 (2013) no. 5, pp. 1031-1040 | DOI
[31] Hidden Semi Markov Models for Multiple Observation Sequences: The mhsmm Package for R, Journal of Statistical Software, Volume 39 (2011) no. 4 | DOI
[32] Foraging theory upscaled: the behavioural ecology of herbivore movement, Philosophical Transactions of the Royal Society B: Biological Sciences, Volume 365 (2010) no. 1550, pp. 2267-2278 | DOI
[33] Peer Community in Mathematical and Computational Biology (2025) | DOI
[34] Selecting the Number of States in Hidden Markov Models: Pragmatic Solutions Illustrated Using Animal Movement, Journal of Agricultural, Biological and Environmental Statistics, Volume 22 (2017) no. 3, pp. 270-293 | DOI
[35] Linear predictive hidden Markov models and the speech signal, Proc.ICASSP ’82. pp.1291-1294, Paris, France, May 1982 (1982) | DOI
[36] R: A Language and Environment for Statistical Computing, https://www.R-project.org/, 2022
[37] A tutorial on hidden Markov models and selected applications in speech recognition, Proceedings of the IEEE, Volume 77 (1989) no. 2, pp. 257-286 | DOI
[38] Probabilités, analyses des données et statistique, Technip
[39] Robust classification of animal tracking data, Computers and Electronics in Agriculture, Volume 56 (2007) no. 1, pp. 46-59 | DOI
[40] An EM and a Stochastic Version of the EM Algorithm for Nonparametric Hidden Semi-Markov Models, Communications in Statistics - Simulation and Computation, Volume 39 (2010) no. 2, pp. 240-261 | DOI
[41] Improved supervised classification of accelerometry data to distinguish behaviors of soaring birds, PLOS ONE, Volume 12 (2017) no. 4, p. e0174785 | DOI
[42] A validated approach for supervised dive classification in diving vertebrates, Journal of Experimental Marine Biology and Ecology, Volume 363 (2008) no. 1, pp. 75-83 | DOI
[43] Identifying fishing trip behaviour and estimating fishing effort from VMS data using Bayesian Hidden Markov Models, Ecological Modelling, Volume 221 (2010) no. 15, pp. 1757-1769 | DOI
[44] Correlation Theory of Stationary and Related Random Functions, Springer
[45] Hidden Markov models for time series: an introduction using R, Monographs on statistics and applied probability, CRC Press, 2009 no. 110 | DOI
Cited by Sources: