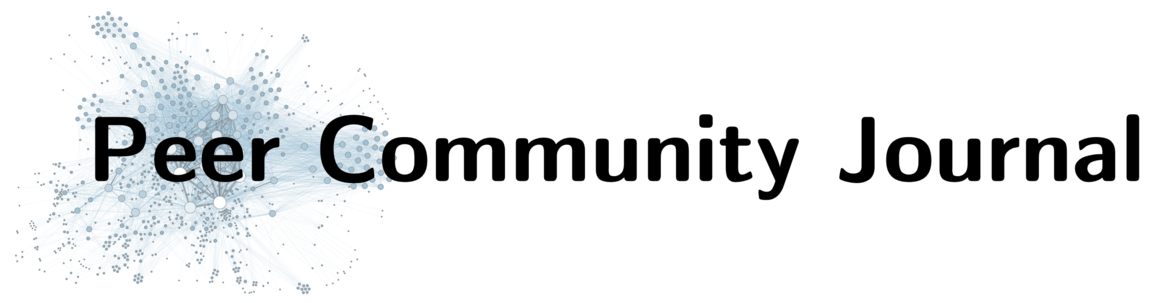
Section: Ecology
Topic:
Biophysics and computational biology,
Ecology,
Environmental sciences
Reconstructing prevalence dynamics of wildlife pathogens from pooled and individual samples
Corresponding author(s): Borremans, Benny (bennyborremans@gmail.com)
10.24072/pcjournal.455 - Peer Community Journal, Volume 4 (2024), article no. e80.
Get full text PDF Peer reviewed and recommended by PCI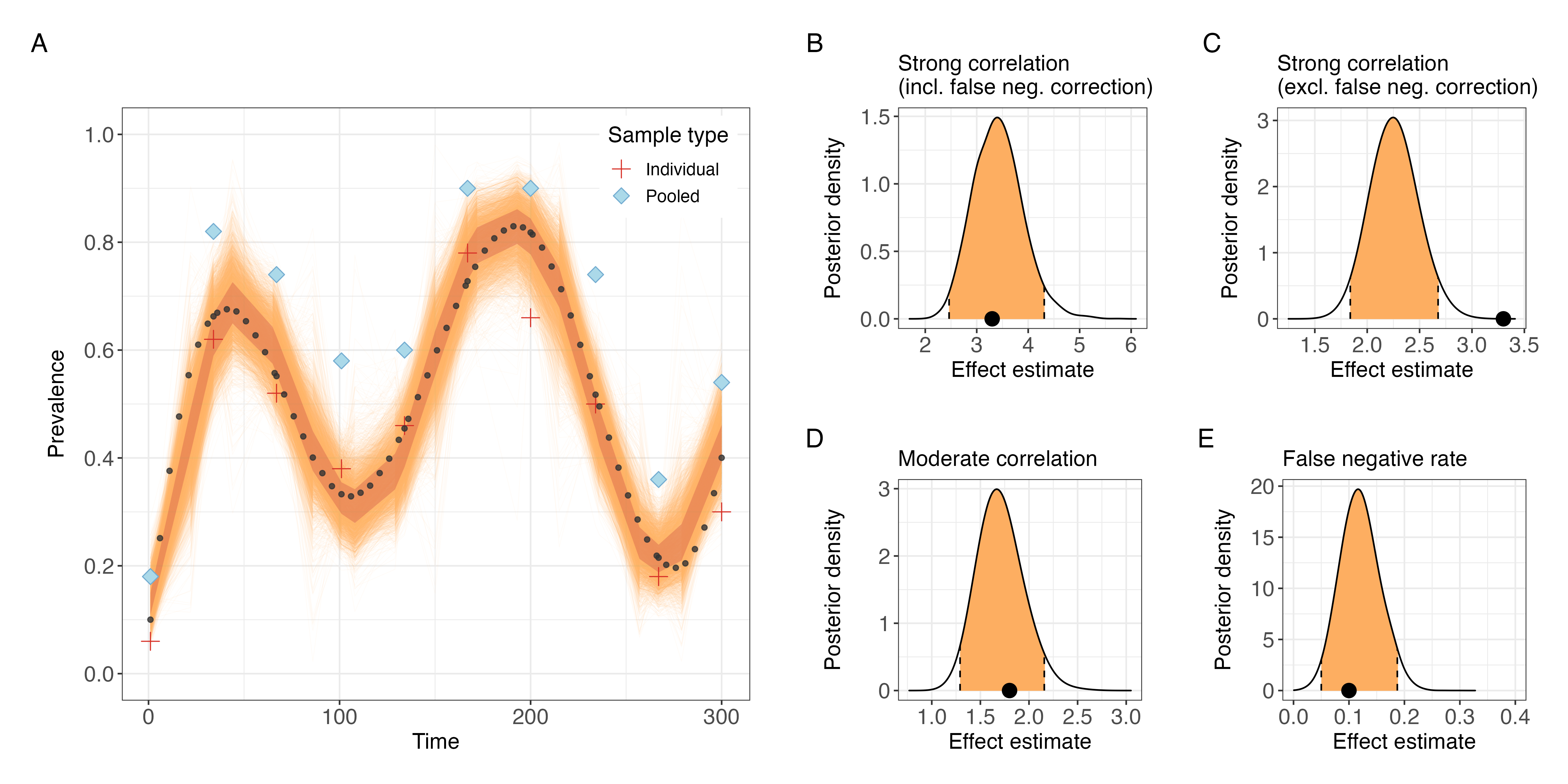
Pathogen transmission studies require sample collection over extended periods, which can be challenging and costly, especially in the case of wildlife. A useful strategy can be to collect pooled samples, but this presents challenges when the goal is to estimate prevalence. This is because pooling can introduce a dilution effect where pathogen concentration is lowered by the inclusion of negative or lower-concentration samples, while at the same time a pooled sample can test positive even when some of the contributing samples are negative. If these biases are taken into account, the concentration of a pooled sample can be leveraged to infer the most likely proportion of positive individuals, and thus improve overall prevalence reconstruction, but few methods exist that account for the sample mixing process. We present a Bayesian multilevel model that estimates prevalence dynamics over time using pooled and individual samples in a wildlife setting. The model explicitly accounts for the complete mixing process that determines pooled sample concentration, thus enabling accurate prevalence estimation even from pooled samples only. As it is challenging to link individual-level metrics such as age, sex, or immune markers to infection status when using pooled samples, the model also allows the incorporation of individual-level samples. Crucially, when individual samples can test false negative, a potentially strong bias is introduced that results in incorrect estimates of regression coefficients. The model, however, can account for this by leveraging the combination of pooled and individual samples. Last, the model enables estimation of extrinsic environmental effects on prevalence dynamics. Using a simulated dataset inspired by virus transmission in flying foxes, we show that the model is able to accurately estimate prevalence dynamics, false negative rate, and covariate effects. We test model performance for a range of realistic sampling scenarios and find that while it is generally robust, there are a number of factors that should be considered in order to maximize performance. The model presents an important advance in the use of pooled samples for estimating prevalence dynamics in a wildlife setting, can be used with any biomarker of infection (Ct values, antibody levels, other infection biomarkers) and can be applied to a wide range of host-pathogen systems.
Type: Research article
Borremans, Benny 1, 2, 3; Falvo, Caylee A 2; Crowley, Daniel E 2; Hoegh, Andrew 4; Lloyd-Smith, James O 5; Peel, Alison J 6; Restif, Olivier 7; Ruiz-Aravena, Manuel 2, 6, 8; Plowright, Raina K 2
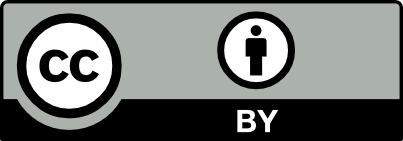
@article{10_24072_pcjournal_455, author = {Borremans, Benny and Falvo, Caylee A and Crowley, Daniel E and Hoegh, Andrew and Lloyd-Smith, James O and Peel, Alison J and Restif, Olivier and Ruiz-Aravena, Manuel and Plowright, Raina K}, title = {Reconstructing prevalence dynamics of wildlife pathogens from pooled and individual samples}, journal = {Peer Community Journal}, eid = {e80}, publisher = {Peer Community In}, volume = {4}, year = {2024}, doi = {10.24072/pcjournal.455}, language = {en}, url = {https://peercommunityjournal.org/articles/10.24072/pcjournal.455/} }
TY - JOUR AU - Borremans, Benny AU - Falvo, Caylee A AU - Crowley, Daniel E AU - Hoegh, Andrew AU - Lloyd-Smith, James O AU - Peel, Alison J AU - Restif, Olivier AU - Ruiz-Aravena, Manuel AU - Plowright, Raina K TI - Reconstructing prevalence dynamics of wildlife pathogens from pooled and individual samples JO - Peer Community Journal PY - 2024 VL - 4 PB - Peer Community In UR - https://peercommunityjournal.org/articles/10.24072/pcjournal.455/ DO - 10.24072/pcjournal.455 LA - en ID - 10_24072_pcjournal_455 ER -
%0 Journal Article %A Borremans, Benny %A Falvo, Caylee A %A Crowley, Daniel E %A Hoegh, Andrew %A Lloyd-Smith, James O %A Peel, Alison J %A Restif, Olivier %A Ruiz-Aravena, Manuel %A Plowright, Raina K %T Reconstructing prevalence dynamics of wildlife pathogens from pooled and individual samples %J Peer Community Journal %D 2024 %V 4 %I Peer Community In %U https://peercommunityjournal.org/articles/10.24072/pcjournal.455/ %R 10.24072/pcjournal.455 %G en %F 10_24072_pcjournal_455
Borremans, Benny; Falvo, Caylee A; Crowley, Daniel E; Hoegh, Andrew; Lloyd-Smith, James O; Peel, Alison J; Restif, Olivier; Ruiz-Aravena, Manuel; Plowright, Raina K. Reconstructing prevalence dynamics of wildlife pathogens from pooled and individual samples. Peer Community Journal, Volume 4 (2024), article no. e80. doi : 10.24072/pcjournal.455. https://peercommunityjournal.org/articles/10.24072/pcjournal.455/
PCI peer reviews and recommendation, and links to data, scripts, code and supplementary information: 10.24072/pci.ecology.100598
Conflict of interest of the recommender and peer reviewers:
The recommender in charge of the evaluation of the article and the reviewers declared that they have no conflict of interest (as defined in the code of conduct of PCI) with the authors or with the content of the article.
[1] Group testing: an information theory perspective, Foundations and Trends in Communications and Information Theory, Volume 15 (2019) no. 3-4, pp. 196-392 | DOI
[2] Supplementary Information (Code) to Reconstructing prevalence dynamics of wildlife pathogens from pooled and individual samples, Zenodo, 2024 | DOI
[3] Shedding dynamics of Morogoro virus, an African arenavirus closely related to Lassa virus, in its natural reservoir host Mastomys natalensis, Scientific Reports, Volume 5 (2015), p. 10445 | DOI
[4] Hendra virus infection dynamics in the grey-headed flying fox (Pteropus poliocephalus) at the southern-most extent of its range: further evidence this species does not readily transmit the virus to horses, PLoS ONE, Volume 11 (2016) no. 6, p. e0155252 | DOI
[5] Using viral load and epidemic dynamics to optimize pooled testing in resource-constrained settings, Science Translational Medicine, Volume 13 (2021), p. eabf1568 | DOI
[6] Estimating prevalence using composites, Environmental and Ecological Statistics, Volume 8 (2001), pp. 213-236 | DOI
[7] Impact of intestinal microorganisms and protozoan parasites on drinking water quality in Harare, Zimbabwe, Journal of Water, Sanitation and Hygiene for Development, Volume 1 (2011) no. 3, pp. 153-163 | DOI
[8] The detection of defective members of large populations, The Annals of Mathematical Statistics, Volume 14 (1943) no. 4, pp. 436-440 | DOI
[9] Time of year, age class and body condition predict Hendra virus infection in Australian black flying foxes (Pteropus alecto), Epidemiology and Infection, Volume 147 (2019), p. e240 | DOI
[10] Estimation of animal-level prevalence from pooled samples in animal production, Preventive Veterinary Medicine, Volume 49 (2001), pp. 175-190 | DOI
[11] The dynamics of measles in sub-Saharan Africa, Nature, Volume 451 (2008) no. 7179, p. 679-84 | DOI
[12] Spatiotemporal aspects of Hendra virus infection in Pteropid bats (flying-foxes) in Eastern Australia, PLoS ONE, Volume 10 (2015) no. 12, p. e0144055 | DOI
[13] Challenges of copro-parasitological surveys in wild Iberian ibex (Capra pyrenaica) populations addressed through a combination of molecular and statistical tools, Ecology and Evolution, Volume 13 (2023) no. 6, p. e10172 | DOI
[14] Real-time forecasting of infectious disease dynamics with a stochastic semi-mechanistic model, Epidemics, Volume 22 (2018), pp. 56-61 | DOI
[15] Bayesian Data Analysis, Chapman and Hall/CRC, 2013 | DOI
[16] Optimizing noninvasive sampling of a zoonotic bat virus, Ecology and Evolution, Volume 11 (2021) no. 18, pp. 12307-12321 | DOI
[17] Estimating epidemiologic dynamics from cross-sectional viral load distributions, Science, Volume 373 (2021) no. 6552 | DOI
[18] Estimating viral prevalence with data fusion for adaptive two-phase pooled sampling, Ecol Evol, Volume 11 (2021) no. 20, pp. 14012-14023 | DOI
[19] Refractory periods and climate forcing in cholera dynamics, Nature, Volume 436 (2005) no. 7051, pp. 696-700 | DOI
[20] Monte Carlo methods, Wiley Interdisciplinary Reviews: Computational Statistics, Volume 4 (2012) no. 1, pp. 48-58 | DOI
[21] Assessing the evidence supporting fruit bats as the primary reservoirs for Ebola viruses, EcoHealth, Volume 13 (2016) no. 1, pp. 18-25 | DOI
[22] Periodic shifts in viral load increase risk of spillover from bats, bioRxiv (2023) | DOI
[23] Density dependence and persistence of Morogoro arenavirus transmission in a fluctuating population of its reservoir host, Journal of Animal Ecology, Volume 89 (2020) no. 2, pp. 506-518 | DOI
[24] Avian influenza virus infection dynamics in shorebird hosts, Journal of Wildlife Diseases, Volume 48 (2012) no. 2, pp. 322-334 | DOI
[25] COVID-19 wastewater epidemiology: a model to estimate infected populations, Lancet Planet Health, Volume 5 (2021) no. 12, p. e874-e881 | DOI
[26] Bayesian regression for group testing data, Biometrics, Volume 73 (2017) no. 4, pp. 1443-1452 | DOI
[27] A pooled testing strategy for identifying SARS-CoV-2 at low prevalence, Nature, Volume 589 (2021) no. 7841, pp. 276-280 | DOI
[28] RColorBrewer: ColorBrewer Palettes. R package version 1.1-3, 2022 | DOI
[29] Structure of general-population antibody titer distributions to influenza A virus, Scientific Reports, Volume 7 (2017) no. 1, p. 6060 | DOI
[30] patchwork: The Composer of Plots. R package version 1.1.2, 2022 | DOI
[31] Pooled samples hold information about the prevalence of wildlife pathogens, Peer Community in Ecology (2024), p. 100598 | DOI
[32] Prevalence of Leptospira species in environmental soil and water from national parks in Sarawak, Malaysia, Borneo Journal of Resource Science and Technology, Volume 5 (2015) no. 1, pp. 49-57 | DOI
[33] R: A language and environment for statistical computing. R Foundation for Statistical Computing, Vienna, Austria. Version 4.2.1., 2022 (https://www.R-project.org/)
[34] Model-guided fieldwork: practical guidelines for multidisciplinary research on wildlife ecological and epidemiological dynamics, Ecology Letters, Volume 15 (2012) no. 10, p. 1083-94 | DOI
[35] Estimation of Covid-19 Prevalence Dynamics from Pooled Data, Journal of Survey Statistics and Methodology, Volume 11 (2023) no. 5, pp. 1229-1245 | DOI
[36] Capturing the pool dilution effect in group testing regression: A Bayesian approach, Statistics in Medicine, Volume 41 (2022) no. 23, pp. 4682-4696 | DOI
[37] Stan Modeling Language Users Guide and Reference Manual v2.32, 2022 (https://mc-stan.org)
[38] RStan: the R interface to Stan. R package version, 2022 (https://mc-stan.org/)
[39] Calculating the prevalence of soil-transmitted helminth infection through pooling of stool samples: Choosing and optimizing the pooling strategy, PLoS Neglected Tropical Diseases, Volume 13 (2019) no. 3, p. e0007196 | DOI
[40] Emerging zoonotic Influenza A virus detection in Myanmar: surveillance practices and findings, Health Security, Volume 15 (2017) no. 5, pp. 483-493 | DOI
[41] Dynamics of a morbillivirus at the domestic-wildlife interface: Canine distemper virus in domestic dogs and lions, Proceedings of the National Academy of Sciences, Volume 112 (2015) no. 5, p. 1464-9 | DOI
[42] Pooled testing for HIV screening: Capturing the dilution effect, Operations Research, Volume 44 (1996), pp. 543-569 | DOI
[43] ggplot2: Elegant Graphics for Data Analysis, Springer-Verlag New York, 2016 | DOI
[44] ggridges: Ridgeline Plots in 'ggplot2'. R package version 0.5.3, 2021 | DOI
[45] RcppAlgos: High Performance Tools for Combinatorics and Computational Mathematics. R package version 2.6.0., 2022 | DOI
[46] Pooled testing for HIV prevalence estimation: exploiting the dilution effect, Statistics in Medicine, Volume 17 (1998), pp. 1447-1467 | DOI
Cited by Sources: