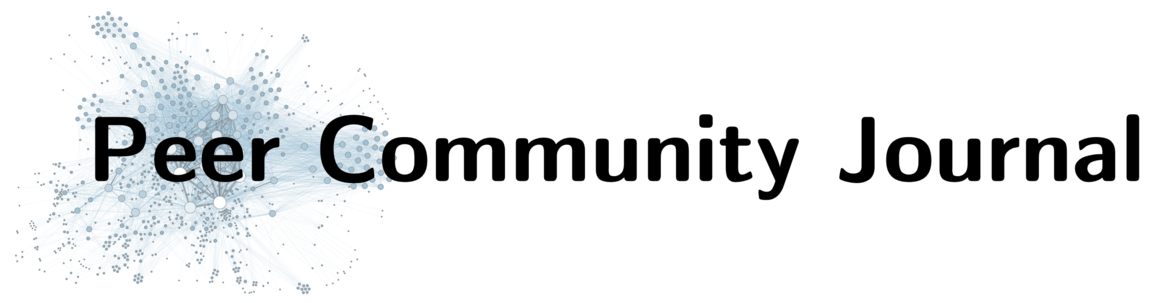
Section: Evolutionary Biology
Topic:
Evolution,
Genetics/Genomics,
Population biology
Joint inference of adaptive and demographic history from temporal population genomic data
Corresponding author(s): de Navascués, Miguel (miguel.navascues@inrae.fr)
10.24072/pcjournal.203 - Peer Community Journal, Volume 2 (2022), article no. e78.
Get full text PDF Peer reviewed and recommended by PCIDisentangling the effects of selection and drift is a long-standing problem in population genetics. Simulations show that pervasive selection may bias the inference of demography. Ideally, models for the inference of demography and selection should account for the interaction between these two forces. With simulation-based likelihood-free methods such as Approximate Bayesian Computation (ABC), demography and selection parameters can be jointly estimated. We propose to use the ABC-Random Forests framework to jointly infer demographic and selection parameters from temporal population genomic data (e.g. experimental evolution, monitored populations, ancient DNA). Our framework allowed the separation of demography (census size, N) from the genetic drift (effective population size, Ne) and the estimation of genome-wide parameters of selection. Selection parameters informed us about the adaptive potential of a population (the scaled mutation rate of beneficial mutations, ), the realized adaptation (the number of mutations under strong selection), and population fitness (genetic load). We applied this approach to a dataset of feral populations of honey bees (Apis mellifera) collected in California, and we estimated parameters consistent with the biology and the recent history of this species.
Type: Research article
Pavinato, Vitor A. C. 1, 2, 3; De Mita, Stéphane 4, 5; Marin, Jean-Michel 2; de Navascués, Miguel 1, 6
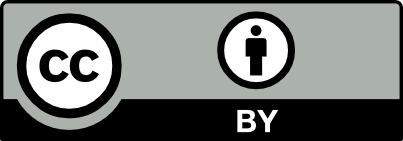
@article{10_24072_pcjournal_203, author = {Pavinato, Vitor A. C. and De Mita, St\'ephane and Marin, Jean-Michel and de Navascu\'es, Miguel}, title = {Joint inference of adaptive and demographic history from temporal population genomic data}, journal = {Peer Community Journal}, eid = {e78}, publisher = {Peer Community In}, volume = {2}, year = {2022}, doi = {10.24072/pcjournal.203}, language = {en}, url = {https://peercommunityjournal.org/articles/10.24072/pcjournal.203/} }
TY - JOUR AU - Pavinato, Vitor A. C. AU - De Mita, Stéphane AU - Marin, Jean-Michel AU - de Navascués, Miguel TI - Joint inference of adaptive and demographic history from temporal population genomic data JO - Peer Community Journal PY - 2022 VL - 2 PB - Peer Community In UR - https://peercommunityjournal.org/articles/10.24072/pcjournal.203/ DO - 10.24072/pcjournal.203 LA - en ID - 10_24072_pcjournal_203 ER -
%0 Journal Article %A Pavinato, Vitor A. C. %A De Mita, Stéphane %A Marin, Jean-Michel %A de Navascués, Miguel %T Joint inference of adaptive and demographic history from temporal population genomic data %J Peer Community Journal %D 2022 %V 2 %I Peer Community In %U https://peercommunityjournal.org/articles/10.24072/pcjournal.203/ %R 10.24072/pcjournal.203 %G en %F 10_24072_pcjournal_203
Pavinato, V. A. C.; De Mita, S.; Marin, J.-M.; de Navascués, M. Joint inference of adaptive and demographic history from temporal population genomic data. Peer Community Journal, Volume 2 (2022), article no. e78. https://doi.org/10.24072/pcjournal.203
PCI peer reviews and recommendation, and links to data, scripts, code and supplementary information: 10.24072/pci.evolbiol.100158
Conflict of interest of the recommender and peer reviewers:
The recommender in charge of the evaluation of the article and the reviewers declared that they have no conflict of interest (as defined in the code of conduct of PCI) with the authors or with the content of the article.
[1] Thinking too positive? Revisiting current methods of population genetic selection inference, Trends in Genetics, Volume 30 (2014) no. 12, pp. 540-546 | DOI
[2] Likelihood-Free Inference of Population Structure and Local Adaptation in a Bayesian Hierarchical Model, Genetics, Volume 185 (2010) no. 2, pp. 587-602 | DOI
[3] Using Genomic Data to Infer Historic Population Dynamics of Nonmodel Organisms, Annual Review of Ecology, Evolution, and Systematics, Volume 49 (2018) no. 1, pp. 433-456 | DOI
[4] Assessing the Evolutionary Impact of Amino Acid Mutations in the Human Genome, PLoS Genetics, Volume 4 (2008) no. 5 | DOI
[5] Random Forests, Machine Learning, Volume 45 (2001) no. 1, pp. 5-32 | DOI
[6] The Linked Selection Signature of Rapid Adaptation in Temporal Genomic Data, Genetics, Volume 213 (2019) no. 3, pp. 1007-1045 | DOI
[7] Estimating the genome-wide contribution of selection to temporal allele frequency change, Proceedings of the National Academy of Sciences, Volume 117 (2020) no. 34, pp. 20672-20680 | DOI
[8] Molecular Population Genetics, Genetics, Volume 205 (2017) no. 3, pp. 1003-1035 | DOI
[9] Genome Sequencing of Museum Specimens Reveals Rapid Changes in the Genetic Composition of Honey Bees in California, Genome Biology and Evolution, Volume 10 (2018) no. 2, pp. 458-472 | DOI
[10] Approximate Bayesian Computation (ABC) in practice, Trends in Ecology & Evolution, Volume 25 (2010) no. 7, pp. 410-418 | DOI
[11] The Effect of Strong Purifying Selection on Genetic Diversity, Genetics, Volume 209 (2018) no. 4, pp. 1235-1278 | DOI
[12] Inference of natural selection from ancient DNA, Evolution Letters, Volume 4 (2020) no. 2, pp. 94-108 | DOI
[13] Finding the missing honey bee genes: lessons learned from a genome upgrade, BMC Genomics, Volume 15 (2014) no. 1 | DOI
[14] Precise assessment of the number of patrilines and of genetic relatedness in honeybee colonies, Proceedings of the Royal Society of London. Series B: Biological Sciences, Volume 258 (1994) no. 1351, pp. 1-7 | DOI
[15] Hitchhiking Under Positive Darwinian Selection, Genetics, Volume 155 (2000) no. 3, pp. 1405-1413 | DOI
[16] Identifying Signatures of Selection in Genetic Time Series, Genetics, Volume 196 (2014) no. 2, pp. 509-522 | DOI
[17] The clarifying role of time series data in the population genetics of HIV, PLOS Genetics, Volume 17 (2021) no. 1 | DOI
[18] Intermediate degrees of synergistic pleiotropy drive adaptive evolution in ecological time, Nature Ecology & Evolution, Volume 1 (2017) no. 10, pp. 1551-1561 | DOI
[19] DILS: Demographic inferences with linked selection by using ABC, Molecular Ecology Resources, Volume 21 (2021) no. 8, pp. 2629-2644 | DOI
[20] Asymptotic properties of approximate Bayesian computation, Biometrika, Volume 105 (2018) no. 3, pp. 593-607 | DOI
[21] Tree‐sequence recording in SLiM opens new horizons for forward‐time simulation of whole genomes, Molecular Ecology Resources, Volume 19 (2019) no. 2, pp. 552-566 | DOI
[22] SLiM 2: Flexible, Interactive Forward Genetic Simulations, Molecular Biology and Evolution, Volume 34 (2017) no. 1, pp. 230-240 | DOI
[23] Estimating the mutation load in human genomes, Nature Reviews Genetics, Volume 16 (2015) no. 6, pp. 333-343 | DOI
[24] Soft sweeps and beyond: understanding the patterns and probabilities of selection footprints under rapid adaptation, Methods in Ecology and Evolution, Volume 8 (2017) no. 6, pp. 700-716 | DOI
[25] Distinguishing Between Selective Sweeps and Demography Using DNA Polymorphism Data, Genetics, Volume 170 (2005) no. 3, pp. 1401-1410 | DOI
[26] On the Utility of Linkage Disequilibrium as a Statistic for Identifying Targets of Positive Selection in Nonequilibrium Populations, Genetics, Volume 176 (2007) no. 4, pp. 2371-2379 | DOI
[27] Toward an Evolutionarily Appropriate Null Model: Jointly Inferring Demography and Purifying Selection, Genetics, Volume 215 (2020) no. 1, pp. 173-192 | DOI
[28] Evidence that Adaptation in Drosophila Is Not Limited by Mutation at Single Sites, PLoS Genetics, Volume 6 (2010) no. 6 | DOI
[29] Linkage Disequilibrium as a Signature of Selective Sweeps, Genetics, Volume 167 (2004) no. 3, pp. 1513-1524 | DOI
[30] Impacts of Recurrent Hitchhiking on Divergence and Demographic Inference in Drosophila, Genome Biology and Evolution, Volume 10 (2018) no. 8, pp. 1882-1891 | DOI
[31] A genome-wide Approximate Bayesian Computation approach suggests only limited numbers of soft sweeps in humans over the last 100,000 years, bioRxiv | DOI
[32] Improving SNP discovery by base alignment quality, Bioinformatics, Volume 27 (2011) no. 8, pp. 1157-1158 | DOI
[33] Joint analysis of demography and selection in population genetics: where do we stand and where could we go?, Molecular Ecology, Volume 21 (2012) no. 1, pp. 28-44 | DOI
[34] Distinguishing Positive Selection From Neutral Evolution: Boosting the Performance of Summary Statistics, Genetics, Volume 187 (2011) no. 1, pp. 229-244 | DOI
[35] Direct Determination of the Mutation Rate in the Bumblebee Reveals Evidence for Weak Recombination-Associated Mutation and an Approximate Rate Constancy in Insects, Molecular Biology and Evolution, Volume 34 (2017) no. 1, pp. 119-130 | DOI
[36] Adaptive protein evolution at the Adh locus in Drosophila, Nature, Volume 351 (1991) no. 6328, pp. 652-654 | DOI
[37] Frequent adaptation and the McDonald–Kreitman test, Proceedings of the National Academy of Sciences, Volume 110 (2013) no. 21, pp. 8615-8620 | DOI
[38] Genetic Draft, Selective Interference, and Population Genetics of Rapid Adaptation, Annual Review of Ecology, Evolution, and Systematics, Volume 44 (2013) no. 1, pp. 195-215 | DOI
[39] Genetic drift and estimation of effective population size, Genetics, Volume 98 (1981) no. 3, pp. 625-640 | DOI
[40] Molecular Signatures of Natural Selection, Annual Review of Genetics, Volume 39 (2005) no. 1, pp. 197-218 | DOI
[41] Effective population size in eusocial Hymenoptera with worker-produced males, Heredity, Volume 109 (2012) no. 5, pp. 261-268 | DOI
[42] Ohio Supercomputer Center, 1987 (http://osc.edu/ark:/19495/f5slph73)
[43] Tracking-selection. Version v1.0.1, zenodo (2022) | DOI
[44] Genetic load in marine animals: a review, Current Zoology, Volume 62 (2016) no. 6, pp. 567-579 | DOI
[45] Population genetic inference from genomic sequence variation, Genome Research, Volume 20 (2010) no. 3, pp. 291-300 | DOI
[46] Reliable ABC model choice via random forests, Bioinformatics, Volume 32 (2016) no. 6, pp. 859-866 | DOI
[47] R: A Language and Environment for Statistical Computing, R: A Language and Environment for Statistical Computing (R Foundation for Statistical Computing. Vienna, Austria), 2020 (https://www.R-project.org/)
[48] ABC random forests for Bayesian parameter inference, Bioinformatics, Volume 35 (2019) no. 10, pp. 1720-1728 | DOI
[49] Population genomics of eusocial insects: the costs of a vertebrate-like effective population size, Journal of Evolutionary Biology, Volume 27 (2014) no. 3, pp. 593-603 | DOI
[50] Is adaptation limited by mutation? A timescale-dependent effect of genetic diversity on the adaptive substitution rate in animals, PLOS Genetics, Volume 16 (2020) no. 4 | DOI
[51] Shedding Light on the Grey Zone of Speciation along a Continuum of Genomic Divergence, PLOS Biology, Volume 14 (2016) no. 12 | DOI
[52] Effective size of populations under selection, Genetics, Volume 139 (1995) no. 2, pp. 1013-1030 | DOI
[53] Population genetics of polymorphism and divergence, Genetics, Volume 132 (1992) no. 4, pp. 1161-1176 | DOI
[54] Background Selection Does Not Mimic the Patterns of Genetic Diversity Produced by Selective Sweeps, Genetics, Volume 216 (2020) no. 2, pp. 499-519 | DOI
[55] Supervised Machine Learning for Population Genetics: A New Paradigm, Trends in Genetics, Volume 34 (2018) no. 4, pp. 301-312 | DOI
[56] Effects of Linked Selective Sweeps on Demographic Inference and Model Selection, Genetics, Volume 204 (2016) no. 3, pp. 1207-1223 | DOI
[57] Pervasive Natural Selection in the Drosophila Genome?, PLoS Genetics, Volume 5 (2009) no. 6 | DOI
[58] Deep Learning for Population Genetic Inference, PLOS Computational Biology, Volume 12 (2016) no. 3 | DOI
[59] EggLib3: A python package for population genetics and genomics, Molecular Ecology Resources, Volume 22 (2022) no. 8, pp. 3176-3187 | DOI
[60] Genetic hitchhiking versus background selection: the controversy and its implications, Philosophical Transactions of the Royal Society B: Biological Sciences, Volume 365 (2010) no. 1544, pp. 1245-1253 | DOI
[61] Statistical method for testing the neutral mutation hypothesis by DNA polymorphism, Genetics, Volume 123 (1989) no. 3, pp. 585-595 | DOI
[62] Detecting and Measuring Selection from Gene Frequency Data, Genetics, Volume 196 (2014) no. 3, pp. 799-817 | DOI
[63] Evolution and Selection of Quantitative Traits, Oxford Scholarship Online, Oxford University Press, 2018 | DOI
[64] Estimating F-Statistics for the Analysis of Population Structure, Evolution, Volume 38 (1984) no. 6 | DOI
[65] Drug Resistance Evolution in HIV in the Late 1990s: Hard Sweeps, Soft Sweeps, Clonal Interference and the Accumulation of Drug Resistance Mutations, G3 Genes|Genomes|Genetics, Volume 10 (2020) no. 4, pp. 1213-1223 | DOI
[66] Effective population size in Hymenoptera with complementary sex determination, Heredity, Volume 93 (2004) no. 6, pp. 627-630 | DOI
Cited by Sources: