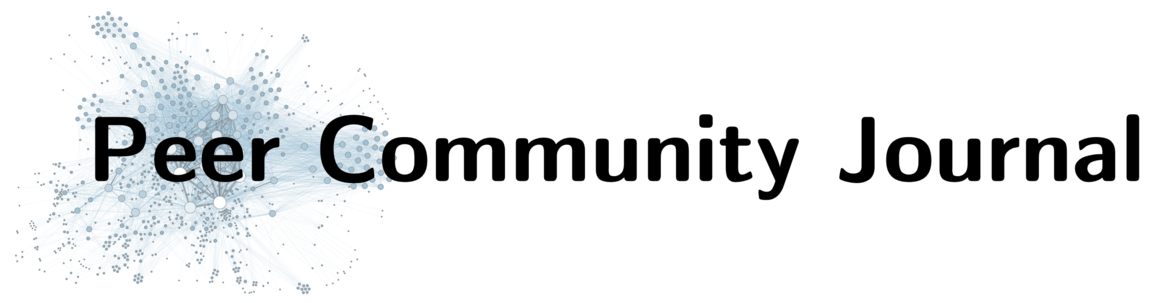
Section: Evolutionary Biology
Topic: Evolution, Genetics/genomics, Computer sciences
Simultaneous Inference of Past Demography and Selection from the Ancestral Recombination Graph under the Beta Coalescent
10.24072/pcjournal.397 - Peer Community Journal, Volume 4 (2024), article no. e33.
Get full text PDF Peer reviewed and recommended by PCIThe reproductive mechanism of a species is a key driver of genome evolution. The standard Wright-Fisher model for the reproduction of individuals in a population assumes that each individual produces a number of offspring negligible compared to the total population size. Yet many species of plants, invertebrates, prokaryotes or fish exhibit neutrally skewed offspring distribution or strong selection events yielding few individuals to produce a number of offspring of up to the same magnitude as the population size. As a result, the genealogy of a sample is characterized by multiple individuals (more than two) coalescing simultaneously to the same common ancestor. The current methods developed to detect such multiple merger events do not account for complex demographic scenarios or recombination, and require large sample sizes. We tackle these limitations by developing two novel and different approaches to infer multiple merger events from sequence data or the ancestral recombination graph (ARG): a sequentially Markovian coalescent (SMβC) and a graph neural network (GNNcoal). We first give proof of the accuracy of our methods to estimate the multiple merger parameter and past demographic history using simulated data under the β-coalescent model. Secondly, we show that our approaches can also recover the effect of positive selective sweeps along the genome. Finally, we are able to distinguish skewed offspring distribution from selection while simultaneously inferring the past variation of population size. Our findings stress the aptitude of neural networks to leverage information from the ARG for inference but also the urgent need for more accurate ARG inference approaches.
Type: Research article
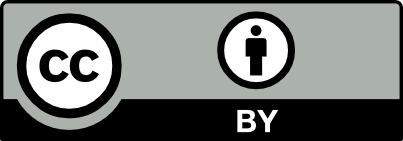
@article{10_24072_pcjournal_397, author = {Korfmann, Kevin and Sellinger, Thibaut Paul Patrick and Freund, Fabian and Fumagalli, Matteo and Tellier, Aur\'elien}, title = {Simultaneous {Inference} of {Past} {Demography} and {Selection} from the {Ancestral} {Recombination} {Graph} under the {Beta} {Coalescent}}, journal = {Peer Community Journal}, eid = {e33}, publisher = {Peer Community In}, volume = {4}, year = {2024}, doi = {10.24072/pcjournal.397}, language = {en}, url = {https://peercommunityjournal.org/articles/10.24072/pcjournal.397/} }
TY - JOUR AU - Korfmann, Kevin AU - Sellinger, Thibaut Paul Patrick AU - Freund, Fabian AU - Fumagalli, Matteo AU - Tellier, Aurélien TI - Simultaneous Inference of Past Demography and Selection from the Ancestral Recombination Graph under the Beta Coalescent JO - Peer Community Journal PY - 2024 VL - 4 PB - Peer Community In UR - https://peercommunityjournal.org/articles/10.24072/pcjournal.397/ DO - 10.24072/pcjournal.397 LA - en ID - 10_24072_pcjournal_397 ER -
%0 Journal Article %A Korfmann, Kevin %A Sellinger, Thibaut Paul Patrick %A Freund, Fabian %A Fumagalli, Matteo %A Tellier, Aurélien %T Simultaneous Inference of Past Demography and Selection from the Ancestral Recombination Graph under the Beta Coalescent %J Peer Community Journal %D 2024 %V 4 %I Peer Community In %U https://peercommunityjournal.org/articles/10.24072/pcjournal.397/ %R 10.24072/pcjournal.397 %G en %F 10_24072_pcjournal_397
Korfmann, Kevin; Sellinger, Thibaut Paul Patrick; Freund, Fabian; Fumagalli, Matteo; Tellier, Aurélien. Simultaneous Inference of Past Demography and Selection from the Ancestral Recombination Graph under the Beta Coalescent. Peer Community Journal, Volume 4 (2024), article no. e33. doi : 10.24072/pcjournal.397. https://peercommunityjournal.org/articles/10.24072/pcjournal.397/
Peer reviewed and recommended by PCI : 10.24072/pci.evolbiol.100699
Conflict of interest of the recommender and peer reviewers:
The recommender in charge of the evaluation of the article and the reviewers declared that they have no conflict of interest (as defined in the code of conduct of PCI) with the authors or with the content of the article.
[1] Selection, recombination, and the ancestral initiation graph, Theoretical Population Biology, Volume 142 (2021), pp. 46-56 | DOI
[2] Nucleotide variation and balancing selection at the Ckma gene in Atlantic cod: analysis with multiple merger coalescent models, PeerJ, Volume 3 (2015) | DOI
[3] Sweepstakes reproductive success via pervasive and recurrent selective sweeps, Elife, Volume 12 (2023) | DOI
[4] The landscape of nucleotide diversity in Drosophila melanogaster is shaped by mutation rate variation, Peer Community Journal, Volume 3 (2023) | DOI
[5] Inference of recombination maps from a single pair of genomes and its application to ancient samples, PLOS Genetics, Volume 15 (2019) no. 11 | DOI
[6] Predicting geographic location from genetic variation with deep neural networks, eLife, Volume 9 (2020) | DOI
[7] Efficient ancestry and mutation simulation with msprime 1.0, Genetics, Volume 220 (2021) no. 3 | DOI
[8] An Ancestral Recombination Graph for Diploid Populations with Skewed Offspring Distribution, Genetics, Volume 193 (2013) no. 1, pp. 255-290 | DOI
[9] A modified lookdown construction for the Xi-Fleming-Viot process with mutation and populations with recurrent bottlenecks, arXiv (2008) | DOI
[10] Coalescent results for diploid exchangeable population models, Electronic Journal of Probability, Volume 23 (2018) | DOI
[11] Sweeps in time: leveraging the joint distribution of branch lengths, Genetics, Volume 219 (2021) no. 2 | DOI
[12] The seed bank coalescent with simultaneous switching, Electronic Journal of Probability, Volume 25 (2020) | DOI
[13] Inferring Population Size History from Large Samples of Genome-Wide Molecular Data - An Approximate Bayesian Computation Approach, PLOS Genetics, Volume 12 (2016) no. 3 | DOI
[14] On Ruelle's probability cascades and an abstract cavity method, Communications in mathematical physics, Volume 197 (1998) no. 2, pp. 247-276 | DOI
[15] Evaluation of methods for estimating coalescence times using ancestral recombination graphs, Genetics, Volume 221 (2022) no. 1 | DOI
[16] The Promise of Inferring the Past using the Ancestral Recombination Graph (ARG), Genome Biology and Evolution (2024) | DOI
[17] Geometric Deep Learning: Going beyond Euclidean data, IEEE Signal Processing Magazine, Volume 34 (2017) no. 4, pp. 18-42 | DOI
[18] Noisy traveling waves: Effect of selection on genealogies, Europhysics Letters, Volume 76 (2006) no. 1, pp. 1-7 | DOI
[19] Effect of selection on ancestry: An exactly soluble case and its phenomenological generalization, Physical Review E, Volume 76 (2007) no. 4, 1 | DOI
[20] Neural networks for self-adjusting mutation rate estimation when the recombination rate is unknown, PLOS Computational Biology, Volume 18 (2022) no. 8, pp. 1-17 | DOI
[21] A Comprehensive Survey on Geometric Deep Learning, IEEE Access, Volume 8 (2020), pp. 35929-35949 | DOI
[22] The Symmetric Coalescent and Wright-Fisher models with bottlenecks, arXiv (2020) | DOI
[23] Population size may shape the accumulation of functional mutations following domestication, BMC Evolutionary Biology, Volume 18 (2018) no. 1 | DOI
[24] Population size may shape the accumulation of functional mutations following domestication, BMC Evolutionary Biology, Volume 18 (2018) no. 1 | DOI
[25] Adaptive value, entropy and survivorship curves, Nature, Volume 275 (1978) no. 5677, pp. 213-214 | DOI
[26] On the length of an external branch in the Beta-coalescent, Stochastic Processes and their Applications, Volume 123 (2013) no. 5, pp. 1691-1715 | DOI
[27] Bursts of coalescence within population pedigrees whenever big families occur, bioRxiv (2023) | DOI
[28] Particle representations for measure-valued population models, Annals of Probability, Volume 27 (1999) no. 1, pp. 166-205 | DOI
[29] A coalescent model for the effect of advantageous mutations on the genealogy of a population, Stochastic Processes and their Applications, Volume 115 (2005) no. 10, pp. 1628-1657 | DOI
[30] Beyond the standard coalescent: demographic inference with complete genomes and graph neural networks under the beta coalescent, Peer Community in Evolutionary Biology (2024) | DOI
[31] Coalescent processes when the distribution of offspring number among individuals is highly skewed, Genetics, Volume 172 (2006) no. 4, pp. 2621-2633 | DOI
[32] Can the Site-Frequency Spectrum Distinguish Exponential Population Growth from Multiple-Merger Coalescents?, Genetics, Volume 199 (2015) no. 3 | DOI
[33] Fast Graph Representation Learning with PyTorch Geometric, arXiv (2019) | DOI
[34] The Unreasonable Effectiveness of Convolutional Neural Networks in Population Genetic Inference, Molecular Biology and Evolution, Volume 36 (2018) no. 2, pp. 220-238 | DOI
[35] Interpreting the pervasive observation of U-shaped Site Frequency Spectra, PLOS Genetics, Volume 19 (2023) no. 3 | DOI
[36] Inferring population size changes with sequence and SNP data: lessons from human bottlenecks, Heredity, Volume 110 (2013) no. 5, pp. 409-419 | DOI
[37] Inferring Past Effective Population Size from Distributions of Coalescent Times, Genetics, Volume 204 (2016) no. 3, pp. 1191-1206 | DOI
[38] Tree‐sequence recording in SLiM opens new horizons for forward‐time simulation of whole genomes, Molecular Ecology Resources, Volume 19 (2019) no. 2, pp. 552-566 | DOI
[39] Considering Genomic Scans for Selection as Coalescent Model Choice, Genome Biology and Evolution, Volume 12 (2020) no. 6, pp. 871-877 | DOI
[40] Sweepstakes reproductive success in highly fecund marine fish and shellfish: a review and Commentary, Bulletin of Marine Science, Volume 87 (2011) no. 4, pp. 971-1002 | DOI
[41] A Deep-Learning Approach for Inference of Selective Sweeps from the Ancestral Recombination Graph, Molecular Biology and Evolution, Volume 39 (2021) no. 1 | DOI
[42] Inference of Ancestral Recombination Graphs Using ARGweaver, Statistical Population Genomics (Methods in Molecular Biology), Volume 2090, Springer US, New York, NY, 2020, pp. 231-266 | DOI
[43] Properties of a neutral allele model with intragenic recombination, Theoretical Population Biology, Volume 23 (1983) no. 2, pp. 183-201 | DOI
[44] Aleatoric and epistemic uncertainty in machine learning: an introduction to concepts and methods, Machine Learning, Volume 110 (2021) no. 3, pp. 457-506 | DOI
[45] Distinguishing between recent balancing selection and incomplete sweep using deep neural networks, Molecular Ecology Resources, Volume 21 (2021) no. 8, pp. 2706-2718 | DOI
[46] Recommendations for improving statistical inference in population genomics, PLOS Biology, Volume 20 (2022) no. 5 | DOI
[47] Toward an Evolutionarily Appropriate Null Model: Jointly Inferring Demography and Purifying Selection, Genetics, Volume 215 (2020) no. 1, pp. 173-192 | DOI
[48] The Impact of Purifying and Background Selection on the Inference of Population History: Problems and Prospects, Molecular Biology and Evolution, Volume 38 (2021) no. 7, pp. 2986-3003 | DOI
[49] Sweepstake evolution revealed by population-genetic analysis of copy-number alterations in single genomes of breast cancer, Royal Society of Open Science, Volume 4 (2017) no. 9 | DOI
[50] Efficient pedigree recording for fast population genetics simulation, PLOS Computational Biology, Volume 14 (2018) no. 11 | DOI
[51] Inferring whole-genome histories in large population datasets, Nature Genetics, Volume 51 (2019) no. 9, pp. 1330-1338 | DOI
[52] The evolving beta coalescent, Electronic Journal of Probability, Volume 19 (2014) | DOI
[53] Exact decoding of the sequentially Markov coalescent, bioRxiv (2020) | DOI
[54] How Many Subpopulations Is Too Many? Exponential Lower Bounds for Inferring Population Histories, Journal of Computational Biology, Volume 27 (2020) no. 4, pp. 613-625 | DOI
[55] The Coalescent, Stochastic Processes and their Applications, Volume 13 (1982) | DOI
[56] Semi-Supervised Classification with Graph Convolutional Networks, arXiv (2016) | DOI
[57] Kevin Korfmann/GNNcoal: GNNcoal (PCI recommendation), Zenodo, 2024 | DOI
[58] Kevin Korfmann/GNNcoal-analysis: GNNcoal-analysis (PCI recommendation), Zenodo, 2024 | DOI
[59] Supplementary Information for "Simultaneous Inference of Past Demography and Selection from the Ancestral Recombination Graph under the Beta Coalescent" publication. In PCJ. , Zenodo, 2024 | DOI
[60] Deep Learning in Population Genetics, Genome Biology and Evolution, Volume 15 (2023) no. 2, p. evad008 | DOI
[61] Multi-locus data distinguishes between population growth and multiple merger coalescents, Statistical Applications in Genetics and Molecular Biology, Volume 17 (2018) no. 3 | DOI
[62] Robust model selection between population growth and multiple merger coalescents, Mathematical Biosciences, Volume 311 (2019), pp. 1-12 | DOI
[63] Graph Classification using Structural Attention, Proceedings of the 24th ACM SIGKDD International Conference on Knowledge Discovery & Data Mining, London United Kingdom, 2018, pp. 1666-1674 | DOI
[64] The era of the ARG: An introduction to ancestral recombination graphs and their significance in empirical evolutionary genomics, PLOS Genetics, Volume 20 (2024) no. 1 | DOI
[65] Inference of human population history from individual whole-genome sequences, Nature, Volume 475 (2011) no. 7357, p. 493-U84 | DOI
[66] Bayesian inference of ancestral recombination graphs, PLOS Computational Biology, Volume 18 (2022) no. 3 | DOI
[67] A genomic history of Aboriginal Australia, Nature, Volume 538 (2016) no. 7624, pp. 207-214 | DOI
[68] Fast "coalescent" simulation, BMC Genetics, Volume 7 (2006) no. 1 | DOI
[69] Coalescent Processes with Skewed Offspring Distributions and Nonequilibrium Demography, Genetics, Volume 208 (2018) no. 1, pp. 323-338 | DOI
[70] Approximating the coalescent with recombination, Philosophical Transactions of the Royal Society B: Biological Sciences, Volume 360 (2005) no. 1459, pp. 1387-1393 | DOI
[71] Multiple Merger Genealogies in Outbreaks of Mycobacterium tuberculosis, Molecular Biology and Evolution, Volume 38 (2020) no. 1, pp. 290-306 | DOI
[72] cggh/scikit-allel: v1.3.3, Zenodo, 2021 | DOI
[73] A classification of coalescent processes for haploid exchangeable population models, Annals of Probability, Volume 29 (2001) no. 4, pp. 1547-1562 | DOI
[74] Evaluating the contributions of purifying selection and progeny-skew in dictating within-host Mycobacterium tuberculosis evolution, Evolution, Volume 74 (2020) no. 5, pp. 992-1001 | DOI
[75] Genealogies of rapidly adapting populations, Proceedings of the National Academy of Sciences, Volume 110 (2013) no. 2, pp. 437-442 | DOI
[76] Accounting for long-range correlations in genome-wide simulations of large cohorts, PLOS Genetics, Volume 16 (2020) no. 5 | DOI
[77] Reproductive skew in Japanese sardine inferred from DNA sequences, ICES Journal of Marine Science, Volume 73 (2016) no. 9, pp. 2181-2189 | DOI
[78] Automatic differentiation in PyTorch, OpenReview (2017) (https://openreview.net/forum?id=BJJsrmfCZ)
[79] Coalescents with multiple collisions, Annals of Probability, Volume 27 (1999) no. 4, pp. 1870-1902 | DOI
[80] Deciphering signatures of natural selection via deep learning, bioRxiv (2021) | DOI
[81] Genome-Wide Inference of Ancestral Recombination Graphs, PLoS Genetics, Volume 10 (2014) no. 5 | DOI
[82] Distinguishing multiple-merger from Kingman coalescence using two-site frequency spectra, bioRxiv (2018) | DOI
[83] Linkage Disequilibrium Between Loci With Unknown Phase, Genetics, Volume 182 (2009) no. 3, pp. 839-844 | DOI
[84] Inferring Demography and Selection in Organisms Characterized by Skewed Offspring Distributions, Genetics, Volume 211 (2019) no. 3, pp. 1019-1028 | DOI
[85] Convergence to the coalescent with simultaneous multiple mergers, Journal of Applied Probability, Volume 40 (2003) no. 4, pp. 839-854 | DOI
[86] The general coalescent with asynchronous mergers of ancestral lines, Journal of Applied Probability, Volume 36 (1999) no. 4, pp. 1116-1125 | DOI
[87] Deep learning for population size history inference: Design, comparison and combination with approximate Bayesian computation, Molecular Ecology Resources, Volume 21 (2020) no. 8, pp. 2645-2660 | DOI
[88] Current progress and open challenges for applying deep learning across the biosciences, Nature Communications, Volume 13 (2022) no. 1 | DOI
[89] A coalescent process with simultaneous multiple mergers for approximating the gene genealogies of many marine organisms, Theoretical population biology, Volume 74 (2008) no. 1, pp. 104-114 | DOI
[90] Inferring human population size and separation history from multiple genome sequences, Nature Genetics, Volume 46 (2014) no. 8, pp. 919-925 | DOI
[91] Modeling Relational Data with Graph Convolutional Networks, arXiv, 2017 | DOI
[92] Coalescent processes obtained from supercritical Galton-Watson processes, Stochastic Processes and their Applications, Volume 106 (2003) no. 1, pp. 107-139 | DOI
[93] Sellinger, Thibaut/eSMC2: eSMC2 : 5.1.3, Zenodo, 2024 | DOI
[94] Limits and convergence properties of the sequentially Markovian coalescent, Molecular Ecology Resources, Volume 21 (2021) no. 7, pp. 2231-2248 | DOI
[95] Inference of past demography, dormancy and self-fertilization rates from whole genome sequence data, PLOS Genetics, Volume 16 (2020) no. 4 | DOI
[96] Deep Learning for Population Genetic Inference, PLOS Computational Biology, Volume 12 (2016) no. 3 | DOI
[97] A method for genome-wide genealogy estimation for thousands of samples, Nature Genetics, Volume 51 (2019) no. 9, pp. 1321-1329 | DOI
[98] Analysis of DNA sequence variation within marine species using Beta-coalescents, Theoretical Population Biology, Volume 87 (2013), pp. 15-24 | DOI
[99] Selective Sweeps, Genetics, Volume 211 (2019) no. 1, pp. 5-13 | DOI
[100] Inference of evolutionary transitions to self-fertilization using whole-genome sequences, bioRxiv (2022)
[101] Coalescence 2.0: a multiple branching of recent theoretical developments and their applications, Molecular Ecology, Volume 23 (2014) no. 11, pp. 2637-2652 | DOI
[102] Robust and scalable inference of population history froth hundreds of unphased whole genomes, Nature Genetics, Volume 49 (2017) no. 2, pp. 303-309 | DOI
[103] Robust Inference of Population Size Histories from Genomic Sequencing Data, bioRxiv, 2021 | DOI
[104] Tracking human population structure through time from whole genome sequences, PLOS Genetics, Volume 16 (2020) no. 3 | DOI
[105] Automatic inference of demographic parameters using generative adversarial networks, Molecular Ecology Resources, Volume 21 (2021) no. 8, pp. 2689-2705 | DOI
[106] Tree sequences as a general-purpose tool for population genetic inference, bioRxiv (2024) | DOI
[107] The SMC' Is a Highly Accurate Approximation to the Ancestral Recombination Graph, Molecular Biology and Evolution, Volume 200 (2015) no. 1, p. 343-U637 | DOI
[108] Recombination as a point process along sequences, Theoretical Population Biology, Volume 55 (1999) no. 3, pp. 248-259 | DOI
[109] A general and efficient representation of ancestral recombination graphs, bioRxiv (2023) | DOI
[110] How Powerful are Graph Neural Networks?, arXiv (2019) | DOI
[111] Revisiting Semi-Supervised Learning with Graph Embeddings, arXiv (2016) | DOI
[112] Creating artificial human genomes using generative neural networks, PLOS Genetics, Volume 17 (2021) no. 2, pp. 1-22 | DOI
[113] Hierarchical Graph Representation Learning with Differentiable Pooling, arXiv (2019) | DOI
[114] Link Prediction Based on Graph Neural Networks, Advances in Neural Information Processing Systems, Volume 31 (2018) (https://proceedings.neurips.cc/paper_files/paper/2018/hash/53f0d7c537d99b3824f0f99d62ea2428-Abstract.html)
[115] Graph neural networks: A review of methods and applications, AI Open, Volume 1 (2020), pp. 57-81 | DOI
Cited by Sources: