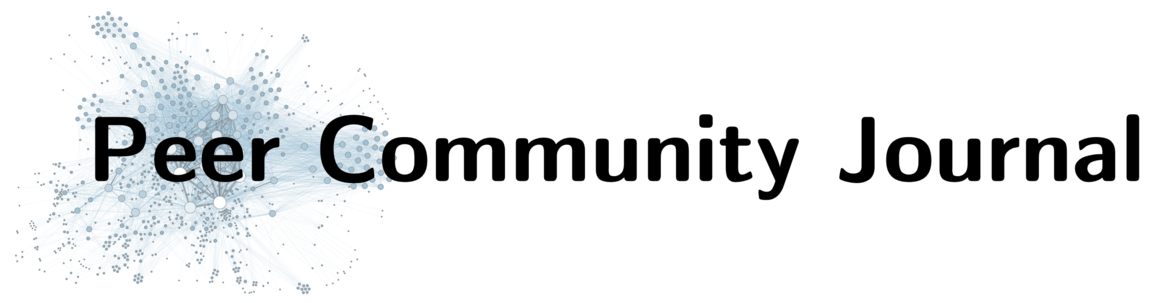
Section: Mathematical & Computational Biology
Topic:
Biophysics and computational biology,
Microbiology,
Systems biology
In silico identification of switching nodes in metabolic networks
Corresponding author(s): Mairet, Francis (francis.mairet@ifremer.fr)
10.24072/pcjournal.480 - Peer Community Journal, Volume 4 (2024), article no. e102.
Get full text PDF Peer reviewed and recommended by PCICells modulate their metabolism according to environmental conditions. A major challenge to better understand metabolic regulation is to identify, from the hundreds or thousands of molecules, the key metabolites where the re-orientation of fluxes occurs. Here, a method called ISIS (for In Silico Identification of Switches) is proposed to locate these nodes in a metabolic network, based on the analysis of a set of flux vectors (obtained e.g. by parsimonious flux balance analysis with different inputs). A metabolite is considered as a switch if the fluxes at this point are redirected in a different way when conditions change. The soundness of ISIS is shown with four case studies, using both core and genome-scale metabolic networks of Escherichia coli, Saccharomyces cerevisiae and the diatom Phaeodactylum tricornutum. Through these examples, we show that ISIS can identify hot-spots where fluxes are reoriented. Additionally, switch metabolites are deeply involved in post-translational modification of proteins, showing their importance in cellular regulation. In P. tricornutum, we show that Erythrose 4-phosphate is an important switch metabolite for mixotrophy suggesting the importance of this metabolite in the non-oxidative pentose phosphate pathway to orchestrate the flux variations between glycolysis, the Calvin cycle and the oxidative pentose phosphate pathway when the trophic mode changes. Finally, a comparison between ISIS and reporter metabolites identified with transcriptomic data confirms the key role of metabolites such as L-glutamate or L-aspartate in the yeast response to nitrogen input variation. Overall, ISIS opens up new possibilities for studying cellular metabolism and regulation, as well as potentially for developing metabolic engineering.
Type: Research article
Mairet, Francis 1
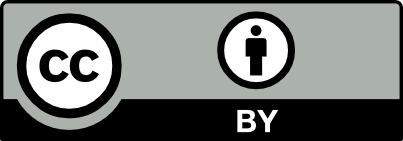
@article{10_24072_pcjournal_480, author = {Mairet, Francis}, title = {\protect\emph{In silico} identification of switching nodes in metabolic networks}, journal = {Peer Community Journal}, eid = {e102}, publisher = {Peer Community In}, volume = {4}, year = {2024}, doi = {10.24072/pcjournal.480}, language = {en}, url = {https://peercommunityjournal.org/articles/10.24072/pcjournal.480/} }
TY - JOUR AU - Mairet, Francis TI - In silico identification of switching nodes in metabolic networks JO - Peer Community Journal PY - 2024 VL - 4 PB - Peer Community In UR - https://peercommunityjournal.org/articles/10.24072/pcjournal.480/ DO - 10.24072/pcjournal.480 LA - en ID - 10_24072_pcjournal_480 ER -
Mairet, Francis. In silico identification of switching nodes in metabolic networks. Peer Community Journal, Volume 4 (2024), article no. e102. doi : 10.24072/pcjournal.480. https://peercommunityjournal.org/articles/10.24072/pcjournal.480/
PCI peer reviews and recommendation, and links to data, scripts, code and supplementary information: 10.24072/pci.mcb.100193
Conflict of interest of the recommender and peer reviewers:
The recommender in charge of the evaluation of the article and the reviewers declared that they have no conflict of interest (as defined in the code of conduct of PCI) with the authors or with the content of the article.
[1] The paradoxes hidden behind the Droop model highlighted by a metabolic approach, Frontiers in Plant Science, Volume 13 (2022) | DOI
[2] DRUM: A New Framework for Metabolic Modeling under Non-Balanced Growth. Application to the Carbon Metabolism of Unicellular Microalgae, PLoS ONE, Volume 9 (2014) no. 8 | DOI
[3] Addressing uncertainty in genome-scale metabolic model reconstruction and analysis, Genome Biology, Volume 22 (2021) no. 1 | DOI
[4] Characterizing posttranslational modifications in prokaryotic metabolism using a multiscale workflow, Proceedings of the National Academy of Sciences, Volume 115 (2018) no. 43, pp. 11096-11101 | DOI
[5] Flux coupling analysis of genome-scale metabolic network reconstructions, Genome research, Volume 14 (2004) no. 2, pp. 301-312 | DOI
[6] A computational method to identify key players in metabolic rewiring, Peer Community in Mathematical and Computational Biology (2024) | DOI
[7] Coordination of microbial metabolism, Nature Reviews Microbiology, Volume 12 (2014) no. 5, pp. 327-340 | DOI
[8] Reconstructing organisms in silico: genome-scale models and their emerging applications, Nature Reviews Microbiology, Volume 18 (2020) no. 12, pp. 731-743 | DOI
[9] A genome-scale metabolic reconstruction for it Escherichia coli K-12 MG1655 that accounts for 1260 ORFs and thermodynamic information, Molecular Systems Biology, Volume 3 (2007) no. 1, p. 121 | DOI
[10] Resource allocation in living organisms, Biochemical Society Transactions, Volume 45 (2017) no. 4, pp. 945-952 | DOI
[11] Current status and applications of genome-scale metabolic models, Genome biology, Volume 20 (2019) no. 1, pp. 1-18 | DOI
[12] Creation and analysis of biochemical constraint-based models using the COBRA Toolbox v.3.0, Nature Protocols, Volume 14 (2019) no. 3, pp. 639-702 | DOI
[13] A consensus yeast metabolic network reconstruction obtained from a community approach to systems biology, Nature Biotechnology, Volume 26 (2008) no. 10, pp. 1155-1160 | DOI
[14] Flux balance analysis of primary metabolism in the diatom it Phaeodactylum tricornutum, The Plant journal, Volume 85 (2016) no. 1, pp. 161-176 | DOI
[15] Metabolite essentiality elucidates robustness of Escherichia coli metabolism, Proceedings of the National Academy of Sciences, Volume 104 (2007) no. 34, pp. 13638-13642 | DOI
[16] Escher: A Web Application for Building, Sharing, and Embedding Data-Rich Visualizations of Biological Pathways, PLOS Computational Biology, Volume 11 (2015) no. 8 | DOI
[17] An Introduction to Metabolic Networks and Their Structural Analysis, IEEE/ACM Transactions on Computational Biology and Bioinformatics, Volume 5 (2008) no. 4, pp. 594-617 | DOI
[18] Combining graph and flux-based structures to decipher phenotypic essential metabolites within metabolic networks, PeerJ, Volume 5 (2017) | DOI
[19] Omic data from evolved E. coli are consistent with computed optimal growth from genome‐scale models, Molecular Systems Biology, Volume 6 (2010) no. 1 | DOI
[20] The connectivity structure, giant strong component and centrality of metabolic networks, Bioinformatics, Volume 19 (2003) no. 11, pp. 1423-1430 | DOI
[21] fmairet/ISIS: In silico identification of switching nodes. Version v1.0, 2024 | DOI
[22] optGpSampler: an improved tool for uniformly sampling the solution-space of genome-scale metabolic networks, PloS one, Volume 9 (2014) no. 2, p. e86587 | DOI
[23] Using genome-scale models to predict biological capabilities, Cell, Volume 161 (2015) no. 5, pp. 971-987 | DOI
[24] Reconstruction and use of microbial metabolic networks: the core it Escherichia coli metabolic model as an educational guide, EcoSal plus, Volume 4 (2010) no. 1 | DOI
[25] What is flux balance analysis?, Nature biotechnology, Volume 28 (2010) no. 3, p. 245 | DOI
[26] Uncovering transcriptional regulation of metabolism by using metabolic network topology, Proceedings of the national academy of sciences, Volume 102 (2005) no. 8, pp. 2685-2689 | DOI
[27] Integrating –omics data into genome-scale metabolic network models: principles and challenges, Essays in Biochemistry, Volume 62 (2018) no. 4, pp. 563-574 | DOI
[28] Comparison of different approaches for identifying subnetworks in metabolic networks, Journal of bioinformatics and computational biology, Volume 15 (2017) no. 06, p. 1750025 | DOI
[29] A metabolite-centric view on flux distributions in genome-scale metabolic models, BMC Systems Biology, Volume 7 (2013) no. 1 | DOI
[30] The PEP-pyruvate-oxaloacetate node as the switch point for carbon flux distribution in bacteria, FEMS microbiology reviews, Volume 29 (2005) no. 4, pp. 765-794 | DOI
[31] Global transcriptional and physiological responses of it Saccharomyces cerevisiae to ammonium, L-alanine, or L-glutamine limitation, Applied and environmental microbiology, Volume 72 (2006) no. 9, pp. 6194-6203 | DOI
[32] Seeing the wood for the trees: a forest of methods for optimization and omic-network integration in metabolic modelling, Briefings in Bioinformatics (2017) | DOI
[33] The small world inside large metabolic networks, Proceedings of the Royal Society of London. Series B: Biological Sciences, Volume 268 (2001) no. 1478, pp. 1803-1810 | DOI
[34] Experimental methods for dissecting the it terraincognita of protein-metabolite interactomes, Current Opinion in Systems Biology, Volume 28 (2021), p. 100403 | DOI
[35] Understanding and mathematical modelling of cellular resource allocation in microorganisms: a comparative synthesis, BMC bioinformatics, Volume 22 (2021), pp. 1-22 | DOI
Cited by Sources: