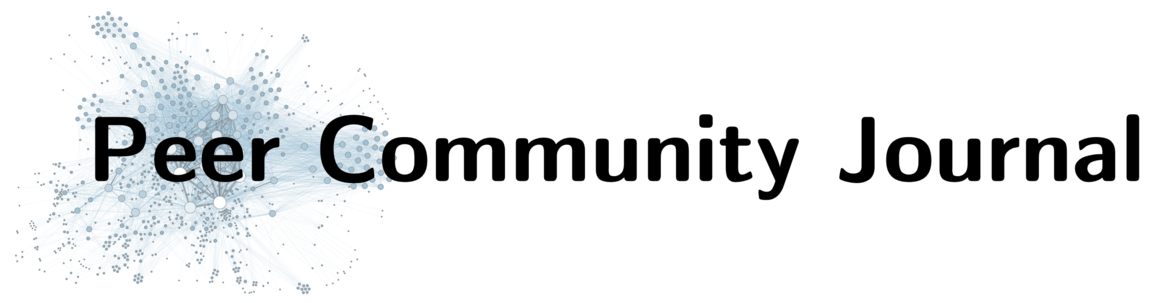
Section: Ecotoxicology & Environmental Chemistry
Topic: Environmental sciences
Machine learning models based on molecular descriptors to predict human and environmental toxicological factors in continental freshwater
10.24072/pcjournal.90 - Peer Community Journal, Volume 2 (2022), article no. e15.
Get full text PDF Peer reviewed and recommended by PCI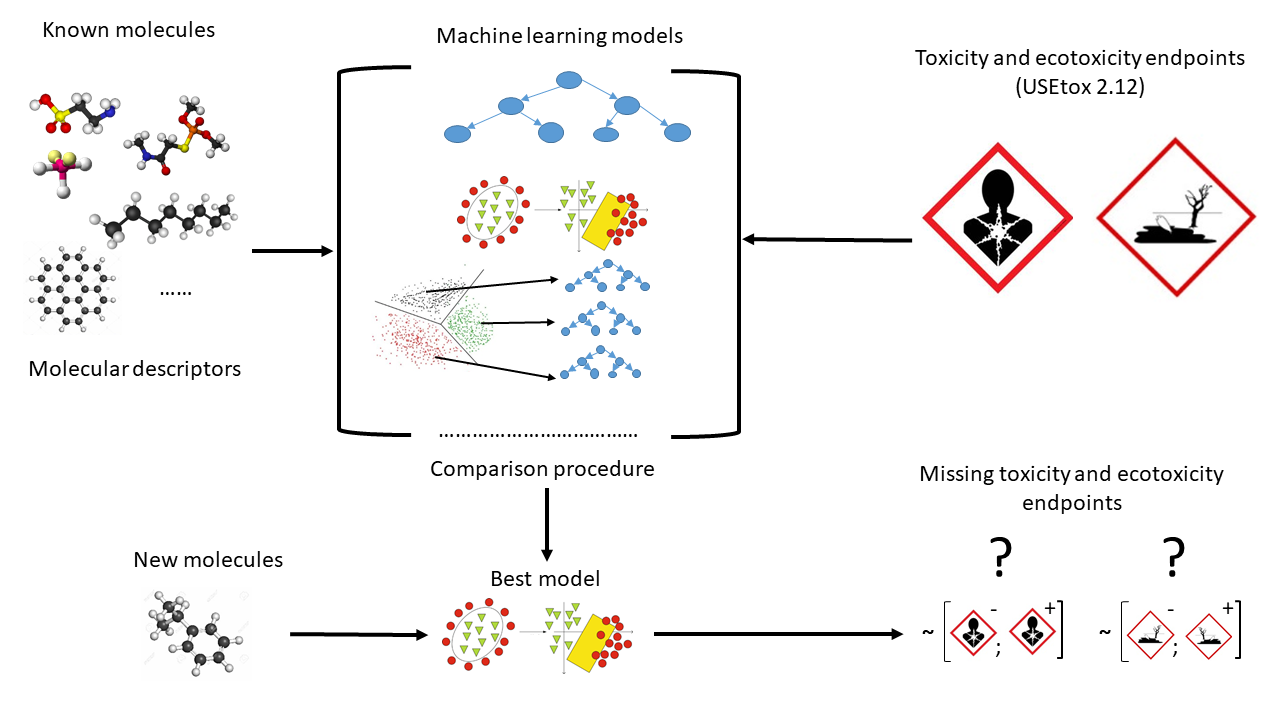
It is a real challenge for life cycle assessment practitioners to identify all relevant substances contributing to ecotoxicity. Once this identification has been made, the lack of corresponding ecotoxicity factors can make the results partial and difficult to interpret. So, it is a real and important challenge to provide ecotoxicity factors for a wide range of compounds. Nevertheless, obtaining such factors using experiments is tedious, time-consuming, and made at a high cost. A modeling method that could predict these factors from easy-to-obtain information on each chemical would be of great value. Here, we present such a method, based on machine learning algorithms, that used molecular descriptors to predict two specific endpoints in continental freshwater for ecotoxicological and human impacts. The different tested machine learning algorithms show good performances on a learning database and the non-linear methods tend to outperform the linear ones. The cluster-then-predict approaches usually show the best performances, which suggests that these predicted models must be derived for somewhat similar compounds. Finally, predictions were derived from the validated model for compounds with missing toxicity/ecotoxicity factors.
Type: Research article
Servien, Rémi 1, 2; Latrille, Eric 1, 2; Patureau, Dominique 2; Hélias, Arnaud 3, 4
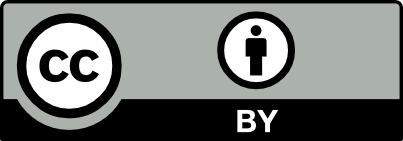
@article{10_24072_pcjournal_90, author = {Servien, R\'emi and Latrille, Eric and Patureau, Dominique and H\'elias, Arnaud}, title = {Machine learning models based on molecular descriptors to predict human and environmental toxicological factors in continental freshwater}, journal = {Peer Community Journal}, eid = {e15}, publisher = {Peer Community In}, volume = {2}, year = {2022}, doi = {10.24072/pcjournal.90}, url = {https://peercommunityjournal.org/articles/10.24072/pcjournal.90/} }
TY - JOUR AU - Servien, Rémi AU - Latrille, Eric AU - Patureau, Dominique AU - Hélias, Arnaud TI - Machine learning models based on molecular descriptors to predict human and environmental toxicological factors in continental freshwater JO - Peer Community Journal PY - 2022 VL - 2 PB - Peer Community In UR - https://peercommunityjournal.org/articles/10.24072/pcjournal.90/ DO - 10.24072/pcjournal.90 ID - 10_24072_pcjournal_90 ER -
%0 Journal Article %A Servien, Rémi %A Latrille, Eric %A Patureau, Dominique %A Hélias, Arnaud %T Machine learning models based on molecular descriptors to predict human and environmental toxicological factors in continental freshwater %J Peer Community Journal %D 2022 %V 2 %I Peer Community In %U https://peercommunityjournal.org/articles/10.24072/pcjournal.90/ %R 10.24072/pcjournal.90 %F 10_24072_pcjournal_90
Servien, Rémi; Latrille, Eric; Patureau, Dominique; Hélias, Arnaud. Machine learning models based on molecular descriptors to predict human and environmental toxicological factors in continental freshwater. Peer Community Journal, Volume 2 (2022), article no. e15. doi : 10.24072/pcjournal.90. https://peercommunityjournal.org/articles/10.24072/pcjournal.90/
PCI peer reviews and recommendation, and links to data, scripts, code and supplementary information: 10.24072/pci.ecotoxenvchem.100001
Conflict of interest of the recommender and peer reviewers:
The recommender in charge of the evaluation of the article and the reviewers declared that they have no conflict of interest (as defined in the code of conduct of PCI) with the authors or with the content of the article.
[1] Impact assessment of a large panel of organic and inorganic micropollutants released by wastewater treatment plants at the scale of France, Water Research, Volume 188 (2021) | DOI
[2] Interpretable random forests via rule extraction In: International Conference on Artificial Intelligence and Statistics (Proceedings of Machine Learning Research), Volume 130 (2021), pp. 937-945
[3] VEGA-QSAR: AI Inside a Platform for Predictive Toxicology, CEUR Workshop Proceedings (2013), pp. 21-28
[4] Categorizing chlordecone potential degradation products to explore their environmental fate, Science of The Total Environment, Volume 574 (2017), pp. 781-795 | DOI
[5] Machine Learning, 45 (2001) no. 1, pp. 5-32 | DOI
[6] Support-vector networks, Machine Learning, Volume 20 (1995) no. 3, pp. 273-297 | DOI
[7] Confronting variability with uncertainty in the ecotoxicological impact assessment of down-the-drain products, Environment International, Volume 126 (2019), pp. 37-45 | DOI
[8] Support Vector Regression Machines In: Proceedings of the 9th International Conference on Neural Information Processing Systems (NIPS'96) , Volume 9, MIT Press, Cambridge, MA, USA (1997), pp. 155-161 | DOI
[9] Danish QSAR database. Danish QSAR group, National Food Institute, Technical University of Denmark, 2015
[10] The New International Standards for Life Cycle Assessment: ISO 14040 and ISO 14044, The International Journal of Life Cycle Assessment, Volume 11 (2006) no. 2, pp. 80-85 | DOI
[11] The Combined QSAR-ICE Models: Practical Application in Ecological Risk Assessment and Water Quality Criteria, Environmental Science & Technology, Volume 51 (2017) no. 16, pp. 8877-8878 | DOI
[12] USEtox fate and ecotoxicity factors for comparative assessment of toxic emissions in life cycle analysis: sensitivity to key chemical properties, The International Journal of Life Cycle Assessment, Volume 16 (2011) no. 8, pp. 701-709 | DOI
[13] Toxic Substances Control Act. , Environmental Law Practice Guide, 4, 2016
[14] Estimate ecotoxicity characterization factors for chemicals in life cycle assessment using machine learning models, Environment International, Volume 135 (2020) | DOI
[15] Rapid Prediction of Chemical Ecotoxicity Through Genetic Algorithm Optimized Neural Network Models, ACS Sustainable Chemistry & Engineering, Volume 8 (2020) no. 32, pp. 12168-12176 | DOI
[16] Comparison of locally weighted PLS strategies for regression and discrimination on agronomic NIR data, Journal of Chemometrics, Volume 34 (2020) no. 5 | DOI
[17] , R News, Volume 2, 2002 no. 3, pp. 18-22
[18] An integrative machine learning approach for prediction of toxicity-related drug safety, Life Science Alliance, Volume 1 (2018) no. 6 | DOI
[19] Prediction of the Fate of Organic Compounds in the Environment From Their Molecular Properties: A Review, Critical Reviews in Environmental Science and Technology, Volume 45 (2015) no. 12, pp. 1277-1377 | DOI
[20] Assessment of pesticides volatilization potential based on their molecular properties using the TyPol tool, Journal of Hazardous Materials, Volume 415 (2021) | DOI
[21] Variables Selection for Ecotoxicity and Human Toxicity Characterization Using Gamma Test, Proceedings of the 14th International Conference on Computational Science and Applications (ICCSA 2014), Springer International Publishing, 2014, pp. 640-652 | DOI
[22] Random Forest for Toxicity of Chemical Emissions: Features Selection and Uncertainty Quantification, Journal of Environmental Accounting and Management, Volume 3 (2015) no. 3, pp. 229-241 | DOI
[23] Methodology document for the ECOlogical Structure-Activity Relationship Model (ECOSAR) class program: estimating toxicity of industrial chemicals to aquatic organisms using ECOSAR class program (Ver. 1.1). In: US Environmental Protection Agency, Office of Chemical Safety and Pollution Prevention, Office of Pollution Prevention and Toxics, Washington, DC, 2011
[24] e1071: Misc Functions of the Department of Statistics, Probability Theory Group (Formerly: E1071), TU Wien. R package version 1.7-2. https://CRAN.R-project.org/package=e1071, 2019
[25] Optimization of classification and regression analysis of four monoclonal antibodies from Raman spectra using collaborative machine learning approach, Talanta, Volume 184 (2018), pp. 260-265 | DOI
[26] Toxicity Testing in the 21st Century, National Academies Press, Washington, D.C., 2007 | DOI
[27] R: A Language and Environment for Statistical Computing. R Foundation for Statistical Computing, Vienna, Austria. http://www.r-project.org/index.html., 2019
[28] mixOmics: An R package for ‘omics feature selection and multiple data integration, PLOS Computational Biology, Volume 13 (2017) no. 11 | DOI
[29] A flexible matrix algebra framework for the multimedia multipathway modeling of emission to impacts, Environment International, Volume 33 (2007) no. 5, pp. 624-634 | DOI
[30] USEtox—the UNEP-SETAC toxicity model: recommended characterisation factors for human toxicity and freshwater ecotoxicity in life cycle impact assessment, The International Journal of Life Cycle Assessment, Volume 13 (2008) no. 7, pp. 532-546 | DOI
[31] Environmental Footprint: Update of Life Cycle Impact Assessment Methods – Ecotoxicity freshwater, human toxicity cancer, and non-cancer, Publications Office of the European Union, Luxembourg, 2020 | DOI
[32] TyPol – A new methodology for organic compounds clustering based on their molecular characteristics and environmental behavior, Chemosphere, Volume 111 (2014), pp. 613-622 | DOI
[33] Improved impact assessment of micropollutants release from WWTPs, Case Studies in Chemical and Environmental Engineering, Volume 5 (2022) | DOI
[34] An Innovative ‘Cluster-then-Predict’ Approach for Improved Sentiment Prediction, Advanced Computing and Communication Technologies, Springer Singapore, Singapore, 2016, pp. 131-140 | DOI
[35] Rapid Life-Cycle Impact Screening Using Artificial Neural Networks, Environmental Science & Technology, Volume 51 (2017) no. 18, pp. 10777-10785 | DOI
[36] Accelerating the pace of ecotoxicological assessment using artificial intelligence, Ambio, Volume 51 (2021) no. 3, pp. 598-610 | DOI
[37] Identification and characterization of tebuconazole transformation products in soil by combining suspect screening and molecular typology, Environmental Pollution, Volume 208 (2016), pp. 537-545 | DOI
[38] Clustering pesticides according to their molecular properties, fate, and effects by considering additional ecotoxicological parameters in the TyPol method, Environmental Science and Pollution Research, Volume 25 (2018) no. 5, pp. 4728-4738 | DOI
[39] Combining cluster analysis with classifier ensembles to predict financial distress, Information Fusion, Volume 16 (2014), pp. 46-58 | DOI
[40] Global Guidance for Life Cycle Impact Assessment Indicators: Volume 2. https://www.lifecycleinitiative.org/training-resources/global-guidance-for-life-cycle-impact-assessment-indicators-volume-2/ (accessed Nov 22, 2020).
[41] USEtox® database system, https://USEtox®.org/model/download, 2020
[42] LCIA framework and cross-cutting issues guidance within the UNEP-SETAC Life Cycle Initiative, Journal of Cleaner Production, Volume 161 (2017), pp. 957-967 | DOI
[43] Advantages of the mean absolute error (MAE) over the root mean square error (RMSE) in assessing average model performance, Climate Research, Volume 30 (2005), pp. 79-82 | DOI
[44] Partial least squares, In: Encyclopedia of statistical sciences, Kotz, Samuel and Johnson, Norman L. (eds.) , Volume 6, Wiley, New York (1985)
[45] Machine Learning Based Toxicity Prediction: From Chemical Structural Description to Transcriptome Analysis, International Journal of Molecular Sciences, Volume 19 (2018) no. 8 | DOI
[46] Compound Cytotoxicity Profiling Using Quantitative High-Throughput Screening, Environmental Health Perspectives, Volume 116 (2008) no. 3, pp. 284-291 | DOI
Cited by Sources: