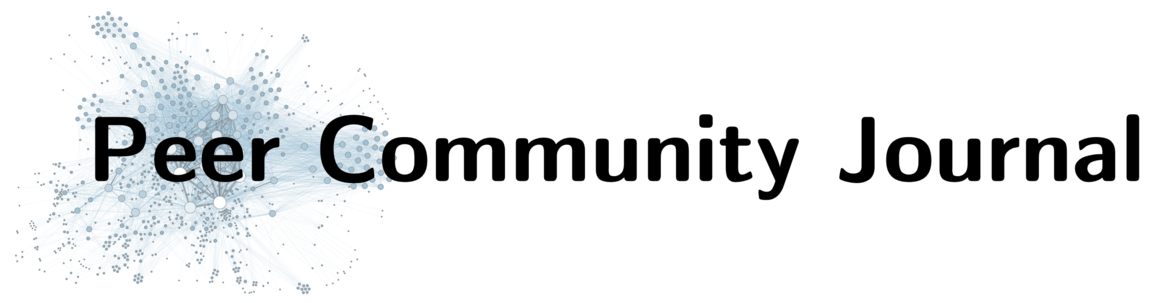
Section: Ecology
Topic: Ecology, Population biology
Modeling Tick Populations: An Ecological Test Case for Gradient Boosted Trees
10.24072/pcjournal.353 - Peer Community Journal, Volume 3 (2023), article no. e116.
Get full text PDF Peer reviewed and recommended by PCIGeneral linear models have been the foundational statistical framework used to discover the ecological processes that explain the distribution and abundance of natural populations. Analyses of the rapidly expanding cache of environmental and ecological data, however, require advanced statistical methods to contend with complexities inherent to extremely large natural data sets. Modern machine learning frameworks such as gradient boosted trees efficiently identify complex ecological relationships in massive data sets, which are expected to result in accurate predictions of the distribution and abundance of organisms in nature. However, rigorous assessments of the theoretical advantages of these methodologies on natural data sets are rare. Here we compare the abilities of gradient boosted and linear models to identify environmental features that explain observed variations in the distribution and abundance of blacklegged tick (Ixodes scapularis) populations in a data set collected across New York State over a ten-year period. The gradient boosted and linear models use similar environmental features to explain tick demography, although the gradient boosted models found non-linear relationships and interactions that are difficult to anticipate and often impractical to identify with a linear modeling framework. Further, the gradient boosted models predicted the distribution and abundance of ticks in years and areas beyond the training data with much greater accuracy than their linear model counterparts. The flexible gradient boosting framework also permitted additional model types that provide practical advantages for tick surveillance and public health. The results highlight the potential of gradient boosted models to discover novel ecological phenomena affecting pathogen demography and as a powerful public health tool to mitigate disease risks.
Type: Research article
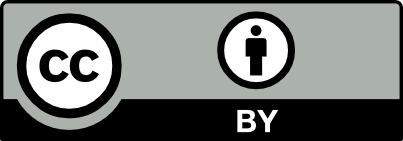
@article{10_24072_pcjournal_353, author = {Manley, William and Tran, Tam and Prusinski, Melissa and Brisson, Dustin}, title = {Modeling {Tick} {Populations:} {An} {Ecological} {Test} {Case} for {Gradient} {Boosted} {Trees}}, journal = {Peer Community Journal}, eid = {e116}, publisher = {Peer Community In}, volume = {3}, year = {2023}, doi = {10.24072/pcjournal.353}, language = {en}, url = {https://peercommunityjournal.org/articles/10.24072/pcjournal.353/} }
TY - JOUR AU - Manley, William AU - Tran, Tam AU - Prusinski, Melissa AU - Brisson, Dustin TI - Modeling Tick Populations: An Ecological Test Case for Gradient Boosted Trees JO - Peer Community Journal PY - 2023 VL - 3 PB - Peer Community In UR - https://peercommunityjournal.org/articles/10.24072/pcjournal.353/ DO - 10.24072/pcjournal.353 LA - en ID - 10_24072_pcjournal_353 ER -
%0 Journal Article %A Manley, William %A Tran, Tam %A Prusinski, Melissa %A Brisson, Dustin %T Modeling Tick Populations: An Ecological Test Case for Gradient Boosted Trees %J Peer Community Journal %D 2023 %V 3 %I Peer Community In %U https://peercommunityjournal.org/articles/10.24072/pcjournal.353/ %R 10.24072/pcjournal.353 %G en %F 10_24072_pcjournal_353
Manley, William; Tran, Tam; Prusinski, Melissa; Brisson, Dustin. Modeling Tick Populations: An Ecological Test Case for Gradient Boosted Trees. Peer Community Journal, Volume 3 (2023), article no. e116. doi : 10.24072/pcjournal.353. https://peercommunityjournal.org/articles/10.24072/pcjournal.353/
Peer reviewed and recommended by PCI : 10.24072/pci.ecology.100532
Conflict of interest of the recommender and peer reviewers:
The recommender in charge of the evaluation of the article and the reviewers declared that they have no conflict of interest (as defined in the code of conduct of PCI) with the authors or with the content of the article.
[1] Comparative Ecology of Galapagos Ground Finches (Geospiza Gould): Evaluation of the Importance of Floristic Diversity and Interspecific Competition, Ecological Monographs, Volume 47 (1977) no. 2, pp. 151-184 | DOI
[2] Niche Modeling of Dengue Fever Using Remotely Sensed Environmental Factors and Boosted Regression Trees, Remote Sensing, Volume 9 (2017) no. 4 | DOI
[3] New approaches to direct gradient analysis using environmental scalars and statistical curve-fitting procedures, Vegetation, Volume 55 (1984) no. 1, pp. 11-27 | DOI
[4] Measurement of the Realized Qualitative Niche: Environmental Niches of Five Eucalyptus Species, Ecological Monographs, Volume 60 (1990) no. 2, pp. 161-177 | DOI
[5] The Crimean‐Congo haemorrhagic fever tick vector Hyalomma marginatum in the south of France: Modelling its distribution and determination of factors influencing its establishment in a newly invaded area, Transboundary and Emerging Diseases, Volume 69 (2022) no. 5 | DOI
[6] Performance evaluation of cetacean species distribution models developed using generalized additive models and boosted regression trees, Ecology and Evolution, Volume 10 (2020) no. 12, pp. 5759-5784 | DOI
[7] A comparative analysis of gradient boosting algorithms, Artificial Intelligence Review, Volume 54 (2021) no. 3, pp. 1937-1967 | DOI
[8] Lyme Disease - a Tick-Borne Spirochetosis?, Science, Volume 216 (1982) no. 4552, pp. 1317-1319 | DOI
[9] On Over-fitting in Model Selection and Subsequent Selection Bias in Performance Evaluation, Journal of Machine Learning Research, Volume 11 (2010) no. 70, pp. 2079-2107
[10] Random Forests for Classification in Ecology, Ecology, Volume 88 (2007) no. 11, pp. 2783-2792 | DOI
[11] Boosted Trees for Ecological Modeling and Prediction, Ecology, Volume 88 (2007) no. 1, pp. 243-251 | DOI
[12] Classification and regression trees: a powerful yet simple technique for ecological data analysis, Ecology, Volume 81 (2000) no. 11, pp. 3178-3192 | DOI
[13] New types of deep neural network learning for speech recognition and related applications: an overview, 2013 IEEE International Conference on Acoustics, Speech and Signal Processing, IEEE, 2013, pp. 8599-8603 | DOI
[14] A working guide to boosted regression trees, Journal of Animal Ecology, Volume 77 (2008) no. 4, pp. 802-813 | DOI
[15] Novel methods improve prediction of species’ distributions from occurrence data, Ecography, Volume 29 (2006) no. 2, pp. 129-151 | DOI
[16] Species Distribution Models: Ecological Explanation and Prediction Across Space and Time, Annual Review of Ecology, Evolution, and Systematics, Volume 40 (2009) no. 1, pp. 677-697 | DOI
[17] Ecological niche modeling re-examined: A case study with the Darwin's fox, Ecology and Evolution, Volume 8 (2018) no. 10, pp. 4757-4770 | DOI
[18] Situating Ecology as a Big-Data Science: Current Advances, Challenges, and Solutions, BioScience, Volume 68 (2018) no. 8, pp. 563-576 | DOI
[19] Predicting the zoonotic capacity of mammals to transmit SARS-CoV-2, Proceedings of the Royal Society B: Biological Sciences, Volume 288 (2021) no. 1963, p. 20211651 | DOI
[20] Greedy function approximation: A gradient boosting machine., The Annals of Statistics, Volume 29 (2001) no. 5, pp. 1189-1232 | DOI
[21] Environmental drivers of spatiotemporal foraging intensity in fruit bats and implications for Hendra virus ecology, Scientific Reports, Volume 8 (2018) no. 1, p. 9555 | DOI
[22] Why do tree-based models still outperform deep learning on typical tabular data?, Advances in Neural Information Processing Systems, Volume 35 (2022), pp. 507-520
[23] Rodent reservoirs of future zoonotic diseases, Proceedings of the National Academy of Sciences, Volume 112 (2015) no. 22, pp. 7039-7044 | DOI
[24] Brain size and ecology in small mammals and primates., Proceedings of the National Academy of Sciences of the United States of America, Volume 77 (1980) no. 7, pp. 4387-4389
[25] The Elements of Statistical Learning: Data Mining, Inference, and Prediction., Springer, New York, 2001
[26] Geographical and environmental factors driving the increase in the Lyme disease vector Ixodes scapularis, Ecosphere, Volume 3 (2012) no. 10, p. 85 | DOI
[27] Body size and metabolic rate, Physiological Reviews, Volume 27 (1947) no. 4, pp. 511-541 | DOI
[28] Will Culling White-Tailed Deer Prevent Lyme Disease?, Zoonoses and Public Health, Volume 63 (2016) no. 5, pp. 337-345 | DOI
[29] Physical and ecological factors explain the distribution of Ross Sea Weddell seals during the breeding season, Marine Ecology Progress Series, Volume 612 (2019), pp. 193-208 | DOI
[30] Ecosystems and the Biosphere as Complex Adaptive Systems, Ecosystems, Volume 1 (1998) no. 5, pp. 431-436 | DOI
[31] Biotic and abiotic factors predicting the global distribution and population density of an invasive large mammal, Scientific Reports, Volume 7 (2017) no. 1, p. 44152 | DOI
[32] A Unified Approach to Interpreting Model Predictions, Procedings of the 31st international conference on neural information processing systems, Volume 30, Curran Associates, Inc., 2017, pp. 4768-4777 | DOI
[33] The role of host phenology for parasite transmission, Theoretical Ecology, Volume 14 (2021) no. 1, pp. 123-143 | DOI
[34] Modeling Tick Populations: An Ecological Test Case for Gradient Boosting Trees. Mendeley Data, 2 (2023) | DOI
[35] Generalized Linear Models, CRC Press, 1989
[36] Sexual Selection and the Risk of Extinction of Introduced Birds on Oceanic Islands, Oikos, Volume 74 (1995) no. 1, pp. 27-34 | DOI
[37] A Comparative Assessment Between Three Machine Learning Models and Their Performance Comparison by Bivariate and Multivariate Statistical Methods in Groundwater Potential Mapping, Water Resources Management, Volume 29 (2015) no. 14, pp. 5217-5236 | DOI
[38] Gradient boosting machines, a tutorial, Frontiers in Neurorobotics, Volume 7 (2013) | DOI
[39] Machine Learning Methods Without Tears: A Primer for Ecologists, The Quarterly Review of Biology, Volume 83 (2008) no. 2, pp. 171-193 | DOI
[40] Climate, Deer, Rodents, and Acorns as Determinants of Variation in Lyme-Disease Risk, PLoS Biology, Volume 4 (2006) no. 6, p. e145 | DOI
[41] Scikit-learn: Machine Learning in Python, Journal of Machine Learning Research, Volume 12 (2011) no. 85, pp. 2825-2830
[42] Gradient Boosted Trees can deliver more than accurate ecological predictions, Peer Community in Ecology (2023), p. 100532 | DOI
[43] Prevalence of Borrelia burgdorferi (Spirochaetales: Spirochaetaceae), Anaplasma phagocytophilum (Rickettsiales: Anaplasmataceae), and Babesia microti (Piroplasmida: Babesiidae) in Ixodes scapularis (Acari: Ixodidae) Collected From Recreational Lands in the Hudson Valley Region, New York State, Journal of Medical Entomology, Volume 51 (2014) no. 1, pp. 226-236 | DOI
[44] No silver bullets in correlative ecological niche modelling: insights from testing among many potential algorithms for niche estimation, Methods in Ecology and Evolution, Volume 6 (2015) no. 10, pp. 1126-1136
[45] Predicting insect outbreaks using machine learning: A mountain pine beetle case study, Ecology and Evolution, Volume 11 (2021) no. 19, pp. 13014-13028 | DOI
[46] Harnessing Deep Learning in Ecology: An Example Predicting Bark Beetle Outbreaks, Frontiers in Plant Science, Volume 10 (2019) | DOI
[47] MLxtend: Providing machine learning and data science utilities and extensions to Python’s scientific computing stack, Journal of Open Source Software, Volume 3 (2018) no. 24 | DOI
[48] Deep Convolutional Neural Networks for Image Classification: A Comprehensive Review, Neural Computation, Volume 29 (2017) no. 9, pp. 2352-2449 | DOI
[49] Energy Constraints on Avian Distributions and Abundances, Ecology, Volume 69 (1988) no. 2, pp. 330-339 | DOI
[50] Stop explaining black box machine learning models for high stakes decisions and use interpretable models instead, Nature Machine Intelligence, Volume 1 (2019) no. 5, pp. 206-215 | DOI
[51] Explainable artificial intelligence enhances the ecological interpretability of black-box species distribution models, Ecography, Volume 44 (2021) no. 2, pp. 199-205 | DOI
[52] Potential Effects of Animal Activity on the Spatial Distribution of Ixodes scapularis and Amblyomma americanum (Acari: Ixodidae), Environmental Entomology, Volume 30 (2001) no. 3, pp. 568-577 | DOI
[53] A comparison of absolute performance of different correlative and mechanistic species distribution models in an independent area, Ecology and Evolution, Volume 6 (2016) no. 16, pp. 5973-5986 | DOI
[54] Malaria smear positivity among Kenyan children peaks at intermediate temperatures as predicted by ecological models, Parasites & Vectors, Volume 12 (2019) no. 1, p. 288 | DOI
[55] Malaria risk assessment and mapping using satellite imagery and boosted regression trees in the Peruvian Amazon, Scientific Reports, Volume 9 (2019) no. 1, p. 15173 | DOI
[56] Human Babesiosis on Nantucket Island, USA: Description of the Vector, Ixodes dammini, n. sp. (Acarina: Ixodidae), Journal of Medical Entomology, Volume 15 (1979) no. 3, pp. 218-234 | DOI
[57] Global Mammal Parasite Database version 2.0, Ecology, Volume 98 (2017) no. 5, p. 1476 | DOI
[58] Kirinyuh (Chromolaena odorata): species distribution modeling and the potential use of fungal pathogens for its eradication, IOP Conference Series: Earth and Environmental Science, Volume 762 (2021) no. 1, p. 012023 | DOI
[59] Perpetuation of the agent of human granulocytic ehrlichiosis in a deer tick-rodent cycle., Proceedings of the National Academy of Sciences, Volume 93 (1996) no. 12, pp. 6209-6214 | DOI
[60] Productivity and sustainability influenced by biodiversity in grassland ecosystems, Nature, Volume 379 (1996) no. 6567, pp. 718-720 | DOI
[61] Estimating disease vector population size from citizen science data, Journal of The Royal Society Interface, Volume 18 (2021b) no. 184, p. 20210610 | DOI
[62] Spatio-temporal variation in environmental features predicts the distribution and abundance of Ixodes scapularis, International Journal for Parasitology, Volume 51 (2021a) no. 4, pp. 311-320 | DOI
[63] Fox sightings in a city are related to certain land use classes and sociodemographics: results from a citizen science project, BMC Ecology, Volume 18 (2018) no. 1, p. 50 | DOI
[64] Taxonomic affinity, habitat and seed mass strongly predict seed desiccation response: a boosted regression trees analysis based on 17539 species, Annals of Botany, Volume 121 (2018) no. 1, pp. 71-83 | DOI
[65] Generalized additive models in plant ecology, Journal of Vegetation Science, Volume 2 (1991) no. 5, pp. 587-602 | DOI
[66] Duration and Regulation of the Developmental Cycle of Ixodes dammini (Acari: Ixodidae), Journal of Medical Entomology, Volume 27 (1990) no. 2, pp. 196-201 | DOI
Cited by Sources: