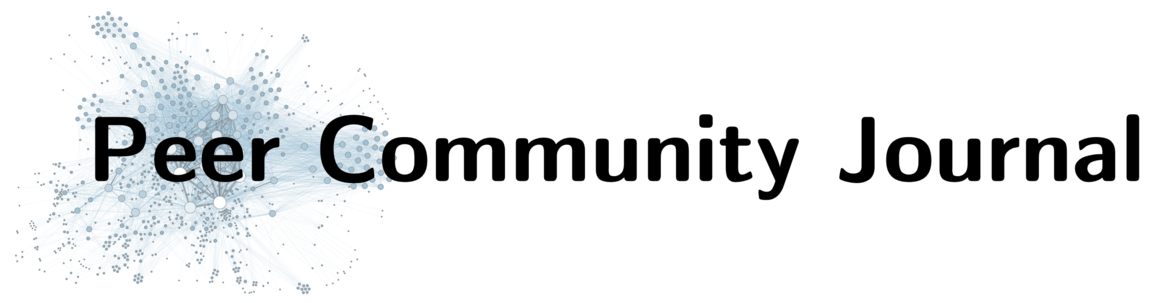
Section: Ecology
Topic: Ecology
Rapid literature mapping on the recent use of machine learning for wildlife imagery
10.24072/pcjournal.261 - Peer Community Journal, Volume 3 (2023), article no. e35.
Get full text PDF Peer reviewed and recommended by PCI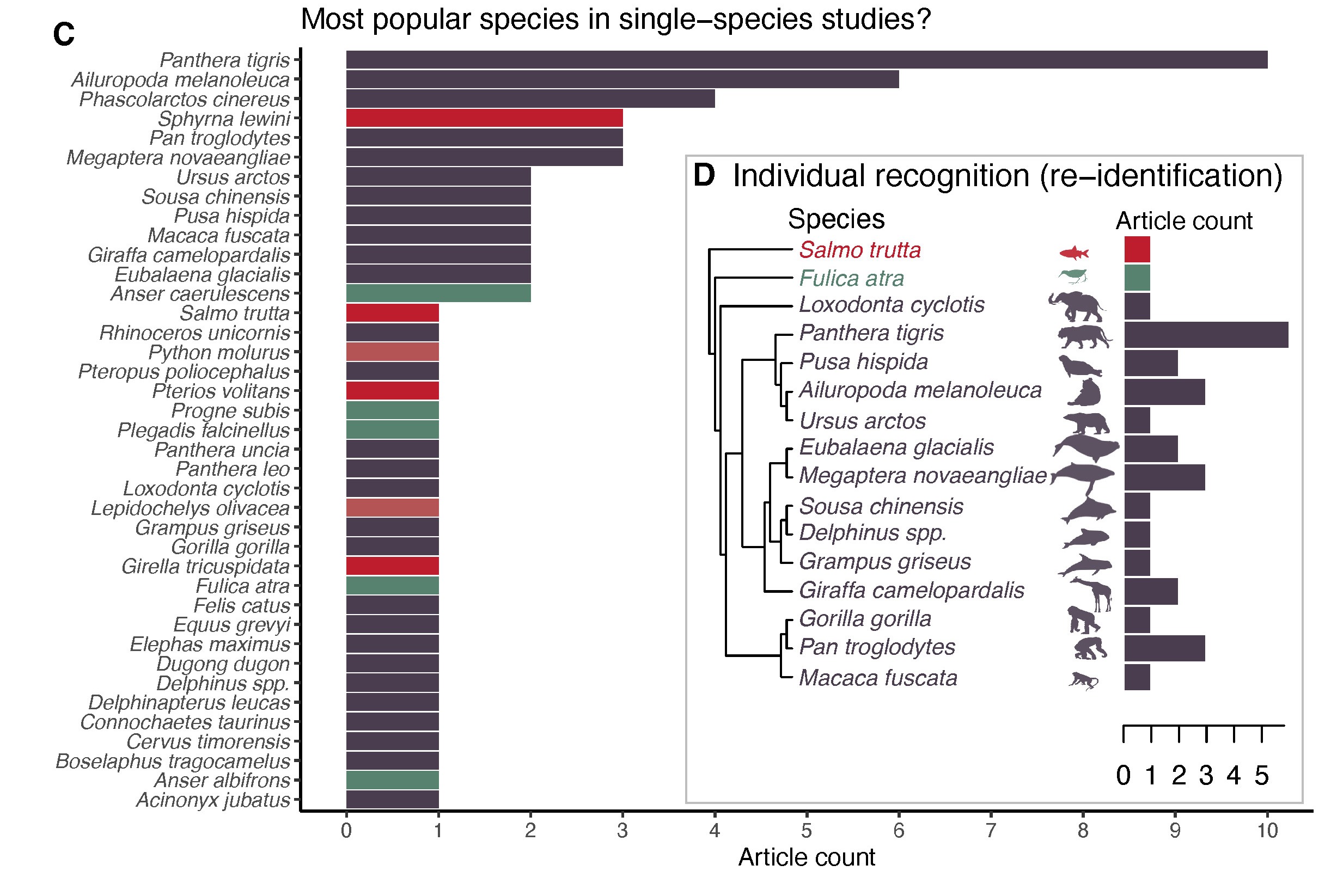
Machine (especially deep) learning algorithms are changing the way wildlife imagery is processed. They dramatically speed up the time to detect, count, and classify animals and their behaviours. Yet, we currently have very few systematic literature surveys on its use in wildlife imagery. Through a literature survey (a ‘rapid’ review) and bibliometric mapping, we explored its use across: 1) species (vertebrates), 2) image types (e.g., camera traps, or drones), 3) study locations, 4) alternative machine learning algorithms, 5) outcomes (e.g., recognition, classification, or tracking), 6) reporting quality and openness, 7) author affiliation, and 8) publication journal types. We found that an increasing number of studies used convolutional neural networks (i.e., deep learning). Typically, studies have focused on large charismatic or iconic mammalian species. An increasing number of studies have been published in ecology-specific journals indicating the uptake of deep learning to transform the detection, classification and tracking of wildlife. Sharing of code was limited, with only 20% of studies providing links to analysis code. Much of the published research and focus on animals came from India, China, Australia, or the USA. There were relatively few collaborations across countries. Given the power of machine learning, we recommend increasing collaboration and sharing approaches to utilise increasing amounts of wildlife imagery more rapidly and transform and improve understanding of wildlife behaviour and conservation. Our survey, augmented with bibliometric analyses, provides valuable signposts for future studies to resolve and address shortcomings, gaps, and biases.
Type: Review article
Nakagawa, Shinichi 1; Lagisz, Malgorzata 1; Francis, Roxane 2; Tam, Jessica 2; Li, Xun 3; Elphinstone, Andrew 4; Jordan, Neil R. 2, 4; O'Brien, Justine K. 2, 4; Pitcher, Benjamin J. 4, 5; Van Sluys, Monique 4; Sowmya, Arcot 3; Kingsford, Richard T. 2
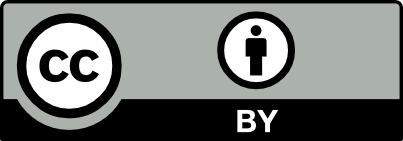
@article{10_24072_pcjournal_261, author = {Nakagawa, Shinichi and Lagisz, Malgorzata and Francis, Roxane and Tam, Jessica and Li, Xun and Elphinstone, Andrew and Jordan, Neil R. and O'Brien, Justine K. and Pitcher, Benjamin J. and Van Sluys, Monique and Sowmya, Arcot and Kingsford, Richard T.}, title = {Rapid literature mapping on the recent use of machine learning for wildlife imagery}, journal = {Peer Community Journal}, eid = {e35}, publisher = {Peer Community In}, volume = {3}, year = {2023}, doi = {10.24072/pcjournal.261}, url = {https://peercommunityjournal.org/articles/10.24072/pcjournal.261/} }
TY - JOUR AU - Nakagawa, Shinichi AU - Lagisz, Malgorzata AU - Francis, Roxane AU - Tam, Jessica AU - Li, Xun AU - Elphinstone, Andrew AU - Jordan, Neil R. AU - O'Brien, Justine K. AU - Pitcher, Benjamin J. AU - Van Sluys, Monique AU - Sowmya, Arcot AU - Kingsford, Richard T. TI - Rapid literature mapping on the recent use of machine learning for wildlife imagery JO - Peer Community Journal PY - 2023 VL - 3 PB - Peer Community In UR - https://peercommunityjournal.org/articles/10.24072/pcjournal.261/ DO - 10.24072/pcjournal.261 ID - 10_24072_pcjournal_261 ER -
%0 Journal Article %A Nakagawa, Shinichi %A Lagisz, Malgorzata %A Francis, Roxane %A Tam, Jessica %A Li, Xun %A Elphinstone, Andrew %A Jordan, Neil R. %A O'Brien, Justine K. %A Pitcher, Benjamin J. %A Van Sluys, Monique %A Sowmya, Arcot %A Kingsford, Richard T. %T Rapid literature mapping on the recent use of machine learning for wildlife imagery %J Peer Community Journal %D 2023 %V 3 %I Peer Community In %U https://peercommunityjournal.org/articles/10.24072/pcjournal.261/ %R 10.24072/pcjournal.261 %F 10_24072_pcjournal_261
Nakagawa, Shinichi; Lagisz, Malgorzata; Francis, Roxane; Tam, Jessica; Li, Xun; Elphinstone, Andrew; Jordan, Neil R.; O'Brien, Justine K.; Pitcher, Benjamin J.; Van Sluys, Monique; Sowmya, Arcot; Kingsford, Richard T. Rapid literature mapping on the recent use of machine learning for wildlife imagery. Peer Community Journal, Volume 3 (2023), article no. e35. doi : 10.24072/pcjournal.261. https://peercommunityjournal.org/articles/10.24072/pcjournal.261/
PCI peer reviews and recommendation, and links to data, scripts, code and supplementary information: 10.24072/pci.ecology.100513
Conflict of interest of the recommender and peer reviewers:
The recommender in charge of the evaluation of the article and the reviewers declared that they have no conflict of interest (as defined in the code of conduct of PCI) with the authors or with the content of the article.
[1] Combining abbreviated literature searches with single-reviewer screening: three case studies of rapid reviews, Systematic Reviews, Volume 9 (2020) no. 1 | DOI
[2] Automated Bird Counting with Deep Learning for Regional Bird Distribution Mapping, Animals, Volume 10 (2020) no. 7 | DOI
[3] A Review on Computer Vision-Based Methods for Human Action Recognition, Journal of Imaging, Volume 6 (2020) no. 6 | DOI
[4] Futurecasting ecological research: the rise of technoecology, Ecosphere, Volume 9 (2018) no. 5 | DOI
[5] A real‐world dataset and data simulation algorithm for automated fish species identification, Geoscience Data Journal, Volume 8 (2021) no. 2, pp. 199-209 | DOI
[6] Decolonizing field ecology, Biotropica, Volume 51 (2019) no. 3, pp. 288-292 | DOI
[7] Recent trends in crowd analysis: A review, Machine Learning with Applications, Volume 4 (2021) | DOI
[8] Towards the fully automated monitoring of ecological communities, Ecology Letters, Volume 25 (2022) no. 12, pp. 2753-2775 | DOI
[9] Taxonomic chauvinism, Trends in Ecology & Evolution, Volume 17 (2002) no. 1, pp. 1-3 | DOI
[10] Toward Using Citizen Scientists to Drive Automated Ecological Object Detection in Aerial Imagery, 2017 IEEE 13th International Conference on e-Science (e-Science) (2017) | DOI
[11] Detecting Wildlife in Unmanned Aerial Systems Imagery Using Convolutional Neural Networks Trained with an Automated Feedback Loop, Lecture Notes in Computer Science, Springer International Publishing, Cham, 2018, pp. 69-82 | DOI
[12] ggalluvial: Layered Grammar for Alluvial Plots, Journal of Open Source Software, Volume 5 (2020) no. 49 | DOI
[13] On Safari with TensorFlow: Assisting Tourism in Rural Southern Africa Using Machine Learning, 2018 International Conference on Advances in Big Data, Computing and Data Communication Systems (icABCD) (2018) | DOI
[14] Collaborating with our community to increase code sharing, PLOS Computational Biology, Volume 17 (2021) no. 3 | DOI
[15] A review of camera trapping for conservation behaviour research, Remote Sensing in Ecology and Conservation, Volume 3 (2017) no. 3, pp. 109-122 | DOI
[16] Exploring Low-light Object Detection Techniques, arXiv (2021) | DOI
[17] Applications for deep learning in ecology, Methods in Ecology and Evolution, Volume 10 (2019) no. 10, pp. 1632-1644 | DOI
[18] Science Mapping Software Tools: Review, Analysis, and Cooperative Study Among Tools, Journal of the American Society for Information Science and Technology, Volume 62 (2011) no. 7, pp. 1382-1402 (781sl) | DOI
[19] Taxonomic bias and international biodiversity conservation research, FACETS, Volume 1 (2017) no. 1, pp. 105-113 | DOI
[20] Using very‐high‐resolution satellite imagery and deep learning to detect and count African elephants in heterogeneous landscapes, Remote Sensing in Ecology and Conservation, Volume 7 (2020) no. 3, pp. 369-381 | DOI
[21] Expediting systematic reviews: methods and implications of rapid reviews, Implementation Science, Volume 5 (2010) no. 1 | DOI
[22] Review of machine learning uses for the analysis of images on wildlife, Peer Community in Ecology (2023) | DOI
[23] Whale counting in satellite and aerial images with deep learning, Scientific Reports, Volume 9 (2019) no. 1 | DOI
[24] GRADE guidelines: 2. Framing the question and deciding on important outcomes, Journal of Clinical Epidemiology, Volume 64 (2011) no. 4, pp. 395-400 | DOI
[25] Egg recognition: The importance of quantifying multiple repeatable features as visual identity signals, PLOS ONE, Volume 16 (2021) no. 3 | DOI
[26] What are the best methodologies for rapid reviews of the research evidence for evidence-informed decision making in health policy and practice: a rapid review, Health Research Policy and Systems, Volume 14 (2016) no. 1 | DOI
[27] The benefits of systematic mapping to evidence-based environmental management, Ambio, Volume 45 (2016) no. 5, pp. 613-620 | DOI
[28] ROSES RepOrting standards for Systematic Evidence Syntheses: pro forma, flow-diagram and descriptive summary of the plan and conduct of environmental systematic reviews and systematic maps, Environmental Evidence, Volume 7 (2018) no. 1 | DOI
[29] Deep learning and computer vision will transform entomology, Proceedings of the National Academy of Sciences, Volume 118 (2021) no. 2 | DOI
[30] Dawn of Drone Ecology: Low-Cost Autonomous Aerial Vehicles for Conservation, Tropical Conservation Science, Volume 5 (2012) no. 2, pp. 121-132 | DOI
[31] Data, statistical scripts, command lines and simulation code. mlagisz/SM_machine_learning_animals: first release updated (v1.0.1), Zenodo (2023) | DOI
[32] Rapid systematic reviews for synthesizing research on built environment, Environmental Development, Volume 43 (2022) | DOI
[33] Deep learning for environmental conservation, Current Biology, Volume 29 (2019) no. 19 | DOI
[34] Deep learning, Nature, Volume 521 (2015) no. 7553, pp. 436-444 | DOI
[35] A survey and performance evaluation of deep learning methods for small object detection, Expert Systems with Applications, Volume 172 (2021) | DOI
[36] Towards Automatic Detection of Animals in Camera-Trap Images, 2018 26th European Signal Processing Conference (Eusipco) (2018), pp. 1805-1809 (Bl7tu)
[37] A logical calculus of the ideas immanent in nervous activity, Bulletin of Mathematical Biology, Volume 52 (1990) no. 1-2, pp. 99-115 | DOI
[38] Camera trapping: wildlife management and research, 2014 Paul Meek and Peter Fleming (principal editors) ; Guy Ballard, Peter Banks, Andrew Claridge, Jim Sanderson and Don Swann (editors).
[39] rotl: an R package to interact with the Open Tree of Life data, Methods in Ecology and Evolution, Volume 7 (2016) no. 12, pp. 1476-1481 | DOI
[40] Empathy and compassion toward other species decrease with evolutionary divergence time, Scientific Reports, Volume 9 (2019) no. 1 | DOI
[41] Large-Scale Automatic Species Identification, AI 2017: Advances in Artificial Intelligence, Springer International Publishing, Cham, 2017, pp. 301-312 | DOI
[42] Identifying the PECO: A framework for formulating good questions to explore the association of environmental and other exposures with health outcomes, Environment International, Volume 121 (2018), pp. 1027-1031 | DOI
[43] A Systematic Mapping of the Advancing Use of Machine Learning Techniques for Predictive Maintenance in the Manufacturing Sector, Applied Sciences, Volume 11 (2021) no. 6 | DOI
[44] Research Weaving: Visualizing the Future of Research Synthesis, Trends in Ecology & Evolution, Volume 34 (2019) no. 3, pp. 224-238 | DOI
[45] Advances in image acquisition and processing technologies transforming animal ecological studies, Ecological Informatics, Volume 61 (2021) | DOI
[46] Automatically identifying, counting, and describing wild animals in camera-trap images with deep learning, Proceedings of the National Academy of Sciences, Volume 115 (2018) no. 25 | DOI
[47] Rayyan—a web and mobile app for systematic reviews, Systematic Reviews, Volume 5 (2016) no. 1 | DOI
[48] The PRISMA 2020 statement: An updated guideline for reporting systematic reviews, PLOS Medicine, Volume 18 (2021) no. 3 | DOI
[49] The ARRIVE guidelines 2.0: Updated guidelines for reporting animal research, PLOS Biology, Volume 18 (2020) no. 7 | DOI
[50] Overview of SnakeCLEF 2021: Automatic snake species identification with country-level focus, Working Notes of CLEF (2021) (http://hdl.handle.net/11025/47274)
[51] Taxonomic Chauvinism in Pesticide Ecotoxicology, Environmental Toxicology and Chemistry, Volume 40 (2021) no. 12, pp. 3223-3225 | DOI
[52] R: A language and environment for statistical computing (2022)
[53] PakhiChini: Automatic Bird Species Identification Using Deep Learning, Proceedings of the 2020 Fourth World Conference on Smart Trends in Systems, Security and Sustainability (Worlds4 2020) (2020), pp. 1-6 (Br0hs)
[54] Detecting animals in African Savanna with UAVs and the crowds, Remote Sensing of Environment, Volume 200 (2017), pp. 341-351 | DOI
[55] Taxonomic bias in animal behaviour publications, Animal Behaviour, Volume 127 (2017), pp. 83-89 | DOI
[56] An Automated Fish Species Identification System Based on Crow Search Algorithm, The International Conference on Advanced Machine Learning Technologies and Applications (AMLTA2018), Springer International Publishing, Cham, 2018, pp. 112-123 | DOI
[57] Reviews: Rapid! Rapid! Rapid! …and systematic, Systematic Reviews, Volume 4 (2015) no. 1 | DOI
[58] rworldmap: a new R package for mapping global data, The R Journal, Volume 3 (2011) no. 1 | DOI
[59] Exploring reporting quality of systematic reviews and Meta-analyses on nursing interventions in patients with Alzheimer’s disease before and after PRISMA introduction, BMC Medical Research Methodology, Volume 18 (2018) no. 1 | DOI
[60] Machine learning to classify animal species in camera trap images: Applications in ecology, Methods in Ecology and Evolution, Volume 10 (2018) no. 4, pp. 585-590 | DOI
[61] Quantifying research interests in 7,521 mammalian species with h-index: a case study, GigaScience, Volume 11 (2022) | DOI
[62] A scoping review of rapid review methods, BMC Medicine, Volume 13 (2015) no. 1 | DOI
[63] Decoloniality and anti-oppressive practices for a more ethical ecology, Nature Ecology & Evolution, Volume 5 (2021) no. 9, pp. 1205-1212 | DOI
[64] Taxonomic bias in biodiversity data and societal preferences, Scientific Reports, Volume 7 (2017) no. 1 | DOI
[65] Perspectives in machine learning for wildlife conservation, Nature Communications, Volume 13 (2022) no. 1 | DOI
[66] Towards automatic wild animal monitoring: Identification of animal species in camera-trap images using very deep convolutional neural networks, Ecological Informatics, Volume 41 (2017), pp. 24-32 | DOI
[67] Deep learning for biology, Nature, Volume 554 (2018) no. 7693, pp. 555-557 | DOI
[68] A computer vision for animal ecology, Journal of Animal Ecology, Volume 87 (2017) no. 3, pp. 533-545 | DOI
[69] ggplot2, Use R!, Springer International Publishing, Cham, 2016 | DOI
[70] The FAIR Guiding Principles for scientific data management and stewardship, Scientific Data, Volume 3 (2016) no. 1 | DOI
[71] Identifying animal species in camera trap images using deep learning and citizen science, Methods in Ecology and Evolution, Volume 10 (2018) no. 1, pp. 80-91 | DOI
[72] Deep learning analysis of nest camera video recordings reveals temperature-sensitive incubation behavior in the purple martin (Progne subis), Behavioral Ecology and Sociobiology, Volume 74 (2019) no. 1 | DOI
[73] Image-Based Recognition of Individual Trouts in the Wild, 2019 8th European Workshop on Visual Information Processing (Euvip 2019) (2019), pp. 82-87 (Bo7ww)
Cited by Sources: