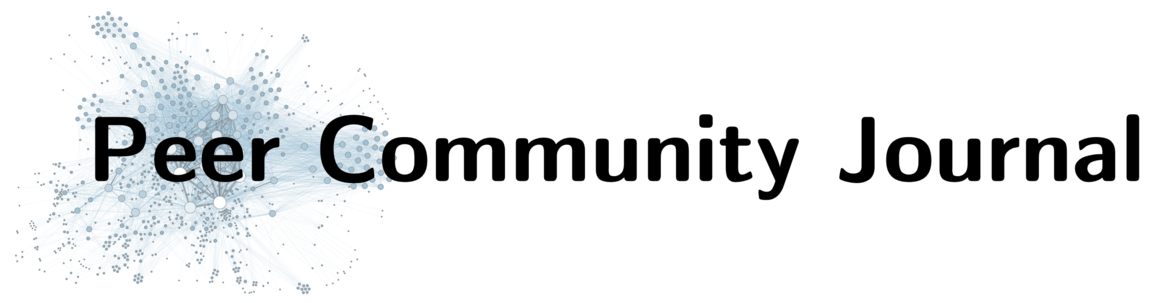
Section: Ecology
Topic:
Ecology,
Statistics
Data stochasticity and model parametrisation impact the performance of species distribution models: insights from a simulation study
Corresponding author(s): Lambert, Charlotte (charlotte.lambert@univ-lr.fr)
10.24072/pcjournal.263 - Peer Community Journal, Volume 3 (2023), article no. e34.
Get full text PDF Peer reviewed and recommended by PCISpecies distribution models (SDM) are widely used to describe and explain how species relate to their environment and predict their spatial distributions. As such, they are the cornerstone of most of spatial planning efforts worldwide. SDM can be implemented with a wide array of data types (presence-only, presence-absence, count...), which can either be point- or areal-based, and use a wide array of environmental conditions as predictor variables. The choice of the sampling type as well as the resolution of environmental conditions to be used are recognized as of crucial importance, yet we lack any quantification of the effects these decisions may have on SDM reliability. In the present work, we fill this gap with an unprecedented simulation procedure. We simulated 100 possible distributions of two different virtual species in two different regions. Species distribution were modelled using either segment- or areal-based sampling and five different spatial resolutions of environmental conditions. The SDM performances were inspected by statistical metrics, model composition, shapes of relationships and prediction quality. We provided clear evidence of stochasticity in the modelling process (particularly in the shapes of relationships): two dataset from the same survey, species and region could yield different results. Sampling type had stronger effects than spatial resolution on the final model relevance. The effect of coarsening the resolution was directly related to the resistance of the spatial features to changes of scale: SDM failed to adequately identify spatial distributions when the spatial features targeted by the species were diluted by resolution coarsening. These results have important implications for the SDM community, backing up some commonly accepted choices, but also by highlighting some up-to-now unexpected features of SDM (stochasticity). As a whole, this work calls for carefully weighted decisions in implementing models, and for caution in interpreting results.
Type: Research article
Lambert, Charlotte 1; Virgili, Auriane 2
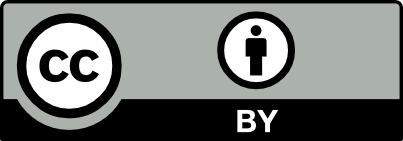
@article{10_24072_pcjournal_263, author = {Lambert, Charlotte and Virgili, Auriane}, title = {Data stochasticity and model parametrisation impact the performance of species distribution models: insights from a simulation study}, journal = {Peer Community Journal}, eid = {e34}, publisher = {Peer Community In}, volume = {3}, year = {2023}, doi = {10.24072/pcjournal.263}, language = {en}, url = {https://peercommunityjournal.org/articles/10.24072/pcjournal.263/} }
TY - JOUR AU - Lambert, Charlotte AU - Virgili, Auriane TI - Data stochasticity and model parametrisation impact the performance of species distribution models: insights from a simulation study JO - Peer Community Journal PY - 2023 VL - 3 PB - Peer Community In UR - https://peercommunityjournal.org/articles/10.24072/pcjournal.263/ DO - 10.24072/pcjournal.263 LA - en ID - 10_24072_pcjournal_263 ER -
%0 Journal Article %A Lambert, Charlotte %A Virgili, Auriane %T Data stochasticity and model parametrisation impact the performance of species distribution models: insights from a simulation study %J Peer Community Journal %D 2023 %V 3 %I Peer Community In %U https://peercommunityjournal.org/articles/10.24072/pcjournal.263/ %R 10.24072/pcjournal.263 %G en %F 10_24072_pcjournal_263
Lambert, C.; Virgili, A. Data stochasticity and model parametrisation impact the performance of species distribution models: insights from a simulation study. Peer Community Journal, Volume 3 (2023), article no. e34. https://doi.org/10.24072/pcjournal.263
PCI peer reviews and recommendation, and links to data, scripts, code and supplementary information: 10.24072/pci.ecology.100523
Conflict of interest of the recommender and peer reviewers:
The recommender in charge of the evaluation of the article and the reviewers declared that they have no conflict of interest (as defined in the code of conduct of PCI) with the authors or with the content of the article.
[1] Spatial Point Patterns: Methodology and Applications with R, Chapman and Hall/CRC Press, London, 2015
[2] Wildlife Population Assessment: Changing Priorities Driven by Technological Advances, Journal of Statistical Theory and Practice, Volume 17 (2023) no. 2, p. 20 | DOI
[3] Distance sampling: methods and applications, Springer, 2015 | DOI
[4] Monitoring of a quasi-stationary eddy in the Bay of Biscay by means of satellite, in situ and model results, Deep-Sea Research II, Volume 106 (2014), pp. 23-37 | DOI
[5] Effects of grain size and niche breadth on species distribution modeling, Ecography, Volume 41 (2017) no. 8, pp. 1270-1282 | DOI
[6] Species Distribution Models: Ecological Explanation and Prediction Across Space and Time, Annual Review of Ecology, Evolution, and Systematics, Volume 40 (2009) no. 1, pp. 677-697 | DOI
[7] The importance of temporal resolution for niche modelling in dynamic marine environments, Journal of Biogeography, Volume 44 (2017) no. 12, pp. 2816-2827 | DOI
[8] Mapping species distributions: spatial inference and prediction, Cambridge University Press, 2010 | DOI
[9] Species distribution models in conservation biogeography: developments and challenges, Diversity and distributions, Volume 19 (2013) no. 10, pp. 1217-1223 | DOI
[10] Influence of grain size on species–habitat models, Ecological Modelling, Volume 222 (2011) no. 18, pp. 3403-3412 | DOI
[11] Is my species distribution model fit for purpose? Matching data and models to applications, Global Ecology and Biogeography, Volume 24 (2015) no. 3, pp. 276-292 | DOI
[12] Sensitivity of predictive species distribution models to change in grain size, Diversity and distributions, Volume 13 (2007) no. 3, pp. 332-340 | DOI
[13] Predicting species distributions for conservation decisions, Ecology Letters, Volume 16 (2013) no. 12, pp. 1424-1435 | DOI
[14] Hmisc: Harrell Miscellaneous, R package version 4.4-2, 2020 (https://CRAN.R-project.org/package=Hmisc)
[15] The effect of sample size and species characteristics on performance of different species distribution modeling methods, Ecography, Volume 29 (2006) no. 5, pp. 773-785 | DOI
[16] raster: Geographic data analysis and modeling, 2014 (http://cran.r-project.org/web/packages/raster/index.html)
[17] Physical processes and hydrological structure related to the Bay of Biscay anchovy , Scientia Marina, Volume 60 (1996) no. Supl. 2, pp. 9-19
[18] Delayed response to environmental conditions and infra-seasonal changes in the spatial distribution of short-beaked common dolphin, Royal Society Open Science (2022) | DOI
[19] The effect of a multi-target protocol on cetacean detection and abundance estimation in aerial surveys, Royal Society open science, Volume 6 (2019) no. 9, p. 190296 | DOI
[20] Predicting cetacean habitats from their energetic needs and the distribution of their prey in two contrasted tropical regions, PLoS One, Volume 9 (2014) no. 8, p. e105958 | DOI
[21] Data stochasticity and model parametrisation impact the performance of species distribution models: insights from a simulation study [Data set], Zenodo, 2023 | DOI
[22] Spatial range shape drives the grain size effects in species distribution models, Ecography, Volume 36 (2013) no. 7, pp. 778-787 | DOI
[23] virtualspecies, an R package to generate virtual species distributions, Ecography, Volume 39 (2015) no. 6, pp. 599-607 | DOI
[24] Ecological geography of the sea, Academic Press, 2007 | DOI
[25] Temporal resolutions in species distribution models of highly mobile marine animals: Recommendations for ecologists and managers, Diversity and Distributions, Volume 23 (2017) no. 10, pp. 1098-1109 | DOI
[26] Predicting cetacean and seabird habitats across a productivity gradient in the South Pacific gyre, Progress in Oceanography, Volume 120 (2014), pp. 383-398 | DOI
[27] Predicting top predator habitats in the Southwest Indian Ocean, Ecography, Volume 37 (2014) no. 3, pp. 261-278 | DOI
[28] Species distribution model transferability and model grain size–finer may not always be better, Scientific reports, Volume 8 (2018) no. 1, pp. 1-9 | DOI
[29] Species distribution modelling to support marine conservation planning: The next steps, Marine Policy, Volume 45 (2014), pp. 330-332 | DOI
[30] dssd: Distance Sampling Survey Design, R package version 0.3.1, 2021 (https://CRAN.R-project.org/package=dssd)
[31] Scale mismatches between predictor and response variables in species distribution modelling: A review of practices for appropriate grain selection, Progress in Physical Geography: Earth and Environment (2023) | DOI
[32] Three anticyclonic Slope Water Oceanic eDDIES (SWODDIES) in the southern Bay of Biscay in 1990, Deep Sea Research, Volume 39 (1992) no. 7/8, pp. 1147-1175 | DOI
[33] Species Distribution Models: the delicate balance between signal and noise, Peer Community in Ecology (2023) | DOI
[34] R: A Language and Environment for Statistical Computing, 2021 (https://www.R-project.org/)
[35] Eddies and dipoles around South Madagascar: formation, pathways and large-scale impact, Deep Sea Research Part I: Oceanographic Research Papers, Volume 51 (2004) no. 3, pp. 383-400 | DOI
[36] Scale of inference: on the sensitivity of habitat models for wide-ranging marine predators to the resolution of environmental data, Ecography, Volume 40 (2016) no. 1, pp. 210-220 | DOI
[37] Eddies and variability in the Mozambique Channel, Deep Sea Research Part II: Topical Studies in Oceanography, Volume 50 (2003) no. 12-13, pp. 1987-2003 | DOI
[38] qpcR: Modelling and Analysis of Real-Time PCR Data, R package version 1.4-1, 2018 (https://CRAN.R-project.org/package=qpcR)
[39] Combining multiple visual surveys to model the habitat of deep-diving cetaceans at the basin scale, Global Ecology and Biogeography, Volume 28 (2018) no. 3, pp. 300-314 | DOI
[40] How many sightings to model rare marine species distributions, PLoS ONE, Volume 13 (2018) no. 3, p. e0193231 | DOI
[41] Comparison of habitat models for scarcely detected species, Ecological Modellling, Volume 346 (2017), pp. 88-98 | DOI
[42] Fast stable restricted maximum likelihood and marginal likelihood estimation of semiparametric generalized linear models, Journal of the Royal Statistical Society: Series B (Statistical Methodology), Volume 73 (2011) no. 1, pp. 3-36 | DOI
[43] Generalized Additive Models: An Introduction with R, Chapman and Hall/CRC, Boca Raton, FL, 2006
Cited by Sources: