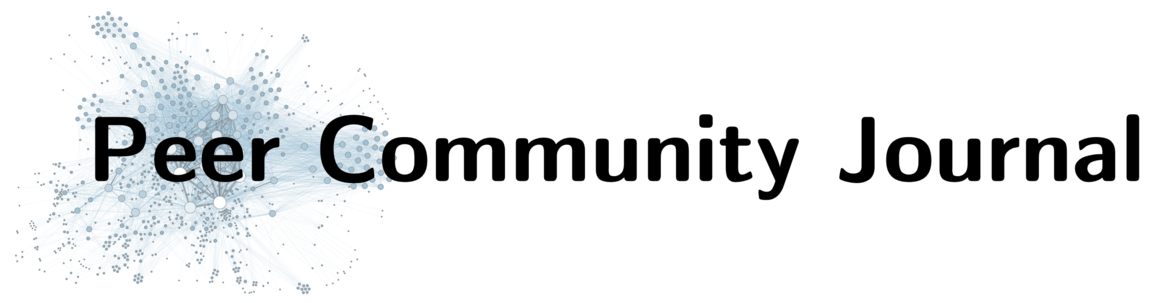
Section: Neuroscience
Topic:
Neuroscience
Nonlinear computations in spiking neural networks through multiplicative synapses
Corresponding author(s): Nardin, Michele (michele.nardin@ist.ac.at); Keemink, Sander W. (sander.keemink@donders.ru.nl)
10.24072/pcjournal.69 - Peer Community Journal, Volume 1 (2021), article no. e68.
Get full text PDF Peer reviewed and recommended by PCIThe brain efficiently performs nonlinear computations through its intricate networks of spiking neurons, but how this is done remains elusive. While nonlinear computations can be implemented successfully in spiking neural networks, this requires supervised training and the resulting connectivity can be hard to interpret. In contrast, the required connectivity for any computation in the form of a linear dynamical system can be directly derived and understood with the spike coding network (SCN) framework. These networks also have biologically realistic activity patterns and are highly robust to cell death. Here we extend the SCN framework to directly implement any polynomial dynamical system, without the need for training. This results in networks requiring a mix of synapse types (fast, slow, and multiplicative), which we term multiplicative spike coding networks (mSCNs). Using mSCNs, we demonstrate how to directly derive the required connectivity for several nonlinear dynamical systems. We also show how to carry out higher-order polynomials with coupled networks that use only pair-wise multiplicative synapses, and provide expected numbers of connections for each synapse type. Overall, our work demonstrates a novel method for implementing nonlinear computations in spiking neural networks, while keeping the attractive features of standard SCNs (robustness, realistic activity patterns, and interpretable connectivity). Finally, we discuss the biological plausibility of our approach, and how the high accuracy and robustness of the approach may be of interest for neuromorphic computing.
Type: Research article
Nardin, Michele 1; Phillips, James W. 2, 3; Podlaski, William F. 4; Keemink, Sander W. 5, 6
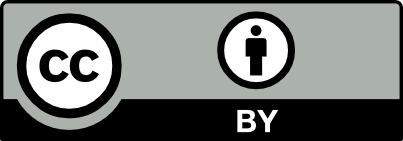
@article{10_24072_pcjournal_69, author = {Nardin, Michele and Phillips, James W. and Podlaski, William F. and Keemink, Sander W.}, title = {Nonlinear computations in spiking neural networks through multiplicative synapses}, journal = {Peer Community Journal}, eid = {e68}, publisher = {Peer Community In}, volume = {1}, year = {2021}, doi = {10.24072/pcjournal.69}, language = {en}, url = {https://peercommunityjournal.org/articles/10.24072/pcjournal.69/} }
TY - JOUR AU - Nardin, Michele AU - Phillips, James W. AU - Podlaski, William F. AU - Keemink, Sander W. TI - Nonlinear computations in spiking neural networks through multiplicative synapses JO - Peer Community Journal PY - 2021 VL - 1 PB - Peer Community In UR - https://peercommunityjournal.org/articles/10.24072/pcjournal.69/ DO - 10.24072/pcjournal.69 LA - en ID - 10_24072_pcjournal_69 ER -
%0 Journal Article %A Nardin, Michele %A Phillips, James W. %A Podlaski, William F. %A Keemink, Sander W. %T Nonlinear computations in spiking neural networks through multiplicative synapses %J Peer Community Journal %D 2021 %V 1 %I Peer Community In %U https://peercommunityjournal.org/articles/10.24072/pcjournal.69/ %R 10.24072/pcjournal.69 %G en %F 10_24072_pcjournal_69
Nardin, M.; Phillips, J. W.; Podlaski, W. F.; Keemink, S. W. Nonlinear computations in spiking neural networks through multiplicative synapses. Peer Community Journal, Volume 1 (2021), article no. e68. https://doi.org/10.24072/pcjournal.69
PCI peer reviews and recommendation, and links to data, scripts, code and supplementary information: 10.24072/pci.cneuro.100003
Conflict of interest of the recommender and peer reviewers:
The recommender in charge of the evaluation of the article and the reviewers declared that they have no conflict of interest (as defined in the code of conduct of PCI) with the authors or with the content of the article.
[1] Building functional networks of spiking model neurons, Nature Neuroscience, Volume 19 (2016) no. 3, pp. 350-355 | DOI
[2] Learning nonlinear dynamics in efficient, balanced spiking networks using local plasticity rules. In: Thirty-second aaai conference on artificial intelligence. URL: https://ojs. aaai.org/index.php/AAAI/article/view/11320, (2018)
[3] Multiplicative and Additive Modulation of Neuronal Tuning with Population Activity Affects Encoded Information, Neuron, Volume 89 (2016) no. 6, pp. 1305-1316 | DOI
[4] Local Calcium Signaling in Neurons, Neuron, Volume 40 (2003) no. 2, pp. 331-346 | DOI
[5] Nonlinear stimulus representations in neural circuits with approximate excitatory-inhibitory balance, PLOS Computational Biology, Volume 16 (2020) no. 9 | DOI
[6] Recurrent neural networks as versatile tools of neuroscience research, Current Opinion in Neurobiology, Volume 46 (2017), pp. 1-6 | DOI
[7] Optimal compensation for neuron loss, eLife, Volume 5 (2016) | DOI
[8] Experimental evidence for sparse firing in the neocortex, Trends in Neurosciences, Volume 35 (2012) no. 6, pp. 345-355 | DOI
[9] Predictive Coding of Dynamical Variables in Balanced Spiking Networks, PLoS Computational Biology, Volume 9 (2013) no. 11 | DOI
[10] Robustness in spiking networks: a geometric perspective, (2020) | DOI
[11] Dimensionality reduction for large-scale neural recordings, Nature Neuroscience, Volume 17 (2014) no. 11, pp. 1500-1509 | DOI
[12] Loihi: A Neuromorphic Manycore Processor with On-Chip Learning, IEEE Micro, Volume 38 (2018) no. 1, pp. 82-99 | DOI
[13] Theoretical neuroscience: computational and mathematical modeling of neural systems. Computational Neuroscience Series. URL: https://mitpress.mit.edu/ books/theoretical-neuroscience, (2021)
[14] The Stone-Weierstrass theorem, Proceedings of the American Mathematical Society, Volume 10 (1959) no. 5, pp. 822-824 | DOI
[15] Efficient codes and balanced networks, Nature Neuroscience, Volume 19 (2016) no. 3, pp. 375-382 | DOI
[16] A Unified Approach to Building and Controlling Spiking Attractor Networks, Neural Computation, Volume 17 (2005) no. 6, pp. 1276-1314 | DOI
[17] Neural engineering: Computation, representation, and dynamics in neurobiological systems, MIT press, (2004)
[18] A Large-Scale Model of the Functioning Brain, Science, Volume 338 (2012) no. 6111, pp. 1202-1205 | DOI
[19] Dense Inhibitory Connectivity in Neocortex, Neuron, Volume 69 (2011) no. 6, pp. 1188-1203 | DOI
[20] Multiplicative computation in a visual neuron sensitive to looming, Nature, Volume 420 (2002) no. 6913, pp. 320-324 | DOI
[21] Cortical connectivity and sensory coding, Nature, Volume 503 (2013) no. 7474, pp. 51-58 | DOI
[22] Neuromorphic Silicon Neuron Circuits, Frontiers in Neuroscience, Volume 5 (2011) | DOI
[23] The “echo state” approach to analysing and training recurrent neural networks- with an erratum note. Bonn, Germany: German National Research Center for Information Tech- nology GMD Technical Report 148, 13. URL: https://www.ai.rug.nl/minds/uploads/ EchoStatesTechRep.pdf, (2001)
[24] Saturated Reconstruction of a Volume of Neocortex, Cell, Volume 162 (2015) no. 3, pp. 648-661 | DOI
[25] Decoding and encoding (de)mixed population responses, Current Opinion in Neurobiology, Volume 58 (2019), pp. 112-121 | DOI
[26] Functional specificity of local synaptic connections in neocortical networks, Nature, Volume 473 (2011) no. 7345, pp. 87-91 | DOI
[27] Multiplying with Synapses and Neurons, Single Neuron Computation, Elsevier, 1992, pp. 315-345 | DOI
[28] Synaptic clustering by dendritic signalling mechanisms, Current Opinion in Neurobiology, Volume 18 (2008) no. 3, pp. 321-331 | DOI
[29] The Excitatory Neuronal Network of the C2 Barrel Column in Mouse Primary Somatosensory Cortex, Neuron, Volume 61 (2009) no. 2, pp. 301-316 | DOI
[30] Double pendulum: An experiment in chaos, American Journal of Physics, Volume 61 (1993) no. 11, pp. 1038-1044 | DOI
[31] Robust neuronal dynamics in premotor cortex during motor planning, Nature, Volume 532 (2016) no. 7600, pp. 459-464 | DOI
[32] Dendritic Computation, Annual Review of Neuroscience, Volume 28 (2005) no. 1, pp. 503-532 | DOI
[33] Deterministic Nonperiodic Flow, Journal of the Atmospheric Sciences, Volume 20 (1963) no. 2, pp. 130-141 | DOI
[34] Real-Time Computing Without Stable States: A New Framework for Neural Computation Based on Perturbations, Neural Computation, Volume 14 (2002) no. 11, pp. 2531-2560 | DOI
[35] Understanding spiking networks through convex optimization. Advances in Neural Information Processing Systems 33. URL: https://proceedings.neurips.cc/paper/ 2020/file/64714a86909d401f8feb83e8c2d94b23-Paper.pdf, (2020)
[36] Context-dependent computation by recurrent dynamics in prefrontal cortex, Nature, Volume 503 (2013) no. 7474, pp. 78-84 | DOI
[37] Linking Connectivity, Dynamics, and Computations in Low-Rank Recurrent Neural Networks, Neuron, Volume 99 (2018) no. 3, pp. 609-623 | DOI
[38] A million spiking-neuron integrated circuit with a scalable communication network and interface, Science, Volume 345 (2014) no. 6197, pp. 668-673 | DOI
[39] Shunting Inhibition Modulates Neuronal Gain during Synaptic Excitation, Neuron, Volume 38 (2003) no. 3, pp. 433-445 | DOI
[40] Surrogate Gradient Learning in Spiking Neural Networks: Bringing the Power of Gradient-Based Optimization to Spiking Neural Networks, IEEE Signal Processing Magazine, Volume 36 (2019) no. 6, pp. 51-63 | DOI
[41] Accurate multiplication with noisy spiking neurons, Journal of Neural Engineering, Volume 8 (2011) no. 3 | DOI
[42] Auditory Spatial Receptive Fields Created by Multiplication, Science, Volume 292 (2001) no. 5515, pp. 249-252 | DOI
[43] A synaptic organizing principle for cortical neuronal groups, Proceedings of the National Academy of Sciences, Volume 108 (2011) no. 13, pp. 5419-5424 | DOI
[44] Pyramidal Neuron as Two-Layer Neural Network, Neuron, Volume 37 (2003) no. 6, pp. 989-999 | DOI
[45] Complexity of contacts between synaptic boutons and dendritic spines in adult rat hippocampus: Three-dimensional reconstructions from serial ultrathin sections in vivo, Synapse, Volume 63 (2009) no. 5, pp. 369-377 | DOI
[46] The importance of mixed selectivity in complex cognitive tasks, Nature, Volume 497 (2013) no. 7451, pp. 585-590 | DOI
[47] The Stabilized Supralinear Network: A Unifying Circuit Motif Underlying Multi-Input Integration in Sensory Cortex, Neuron, Volume 85 (2015) no. 2, pp. 402-417 | DOI
[48] A model of multiplicative neural responses in parietal cortex., Proceedings of the National Academy of Sciences, Volume 93 (1996) no. 21, pp. 11956-11961 | DOI
[49] NMDA spikes in basal dendrites of cortical pyramidal neurons, Nature, Volume 404 (2000) no. 6775, pp. 285-289 | DOI
[50] Cortical Areas Interact through a Communication Subspace, Neuron, Volume 102 (2019) no. 1, pp. 249-259 | DOI
[51] Highly Nonrandom Features of Synaptic Connectivity in Local Cortical Circuits, PLoS Biology, Volume 3 (2005) no. 3 | DOI
[52] High-dimensional geometry of population responses in visual cortex, Nature, Volume 571 (2019) no. 7765, pp. 361-365 | DOI
[53] Spontaneous behaviors drive multidimensional, brainwide activity, Science, Volume 364 (2019) | DOI
[54] Nonlinear dynamics and chaos with student solutions manual: With applications to physics, biology, chemistry, and engineering, CRC press, (2018)
[55] Neural circuits as computational dynamical systems, Current Opinion in Neurobiology, Volume 25 (2014), pp. 156-163 | DOI
[56] Generating Coherent Patterns of Activity from Chaotic Neural Networks, Neuron, Volume 63 (2009) no. 4, pp. 544-557 | DOI
[57] Learning Universal Computations with Spikes, PLOS Computational Biology, Volume 12 (2016) no. 6 | DOI
[58] A Review of Spiking Neuromorphic Hardware Communication Systems, IEEE Access, Volume 7 (2019), pp. 135606-135620 | DOI
[59] The Remarkable Robustness of Surrogate Gradient Learning for Instilling Complex Function in Spiking Neural Networks, Neural Computation, Volume 33 (2021) no. 4, pp. 899-925 | DOI
[60] Nonlinear multiplicative dendritic integration in neuron and network models, Frontiers in Computational Neuroscience, Volume 7 (2013) | DOI
[61] Multiplicative Computation in the Vestibulo-Ocular Reflex (VOR), Journal of Neurophysiology, Volume 97 (2007) no. 4, pp. 2780-2789 | DOI
Cited by Sources: